Will AI Ever Pay Off? Those Footing the Bill Are Worrying Already
.jpg?width=1280&auto=webp&quality=95&format=jpg&disable=upscale)
Though there is some nervousness around how long soaring demand can last, no one
doubts the business models for those at the foundations of the AI stack.
Companies need the chips and manufacturing they, and they alone, offer. Other
winners are the cloud companies that provide data centers. But further up the
ecosystem, the questions become more interesting. That’s where the likes of
OpenAI, Anthropic and many other burgeoning AI startups are engaged in the much
harder job of finding business or consumer uses for this new technology, which
has gained a reputation for being unreliable and erratic. Even if these flaws
can be ironed out (more on that in a moment), there is growing worry about a
perennial mismatch between the cost of creating and running AI and what people
are prepared to pay to use it. ... Another big red flag, economist Daron
Acemoglu warns, lies in the shared thesis that by crunching more data and
engaging more computing power, generative AI tools will become more intelligent
and more accurate, fulfilling their potential as predicted. His comments were
shared in a recent Goldman Sachs report titled “Gen AI: Too Much Spend, Too
Little Benefit?”
How top IT leaders create first-mover advantage

“Some of the less talked about aspects of a high-performing team are the human
traits: trust, respect, genuine enjoyment of each other,” Sample says. “I’m
looking at experience and skills, but I’m also thinking about how the person
will function collaboratively with the team. Do I believe they’ll have the best
interest of the team at heart? Can the team trust their competency? Sample also
says he focuses on “will over skill.” “Qualities like curiosity and
craftsmanship are sustainable, flexible skills that can evolve with whatever the
new ‘toy’ in technology is,” he says. “If you’re approaching work with that
bounty of curiosity and that willing mindset, the skills can adapt.” ...
Steadiness and calm from the leader create the kind of culture where people are
encouraged to take risks and work together to solve big problems and execute on
bold agendas. That, ultimately, is what enables a technology organization to
capitalize on innovative technologies. In fact, reflecting on his legacy as a
CIO, Sample believes it’s not really about the technology; it’s about the
people. His success, he says, has been in building the teams that operate the
technology.
Can AI be Meaningfully Regulated, or is Regulation a Deceitful Fudge?
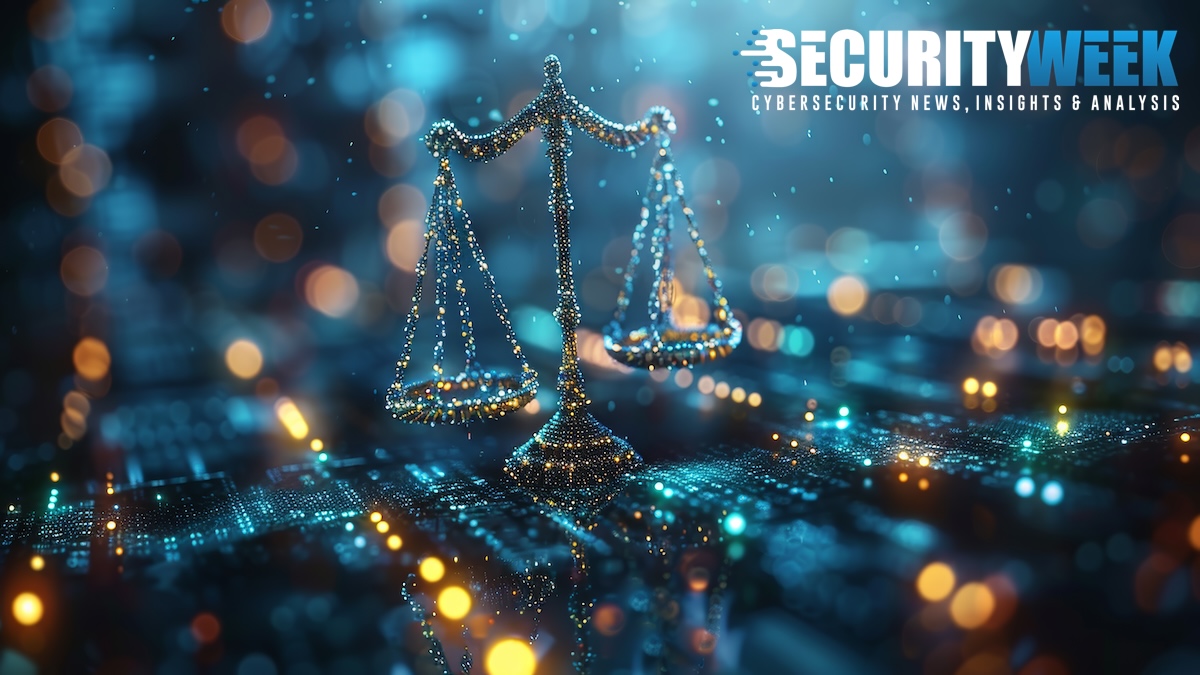
The patchwork approach is used by federal agencies in the US. Different
agencies have responsibility for different verticals and can therefore
introduce regulations more relevant to specific organizations. For example,
the FCC regulates interstate and international communications, the SEC
regulates capital markets and protects investors, and the FTC protects
consumers and promotes competition. ... The danger is that the EU’s recent
monolithic AI Act will go the same way as GDPR. Kolochenko prefers the US
model. He believes the smaller, more agile method of targeted regulations used
by US federal agencies can provide better outcomes than the unwieldy and
largely static monolithic approach adopted by the EU. ... To regulate or not
to regulate is a rhetorical question – of course AI must be regulated to
minimize current and future harms. The real questions are whether it will be
successful (no, it will not), partially successful (perhaps, but only so far
as the curate’s egg is good), and will it introduce new problems for AI-using
businesses (from empirical and historical evidence, yes).
The Team Sport of Cloud Security: Breaking Down the Rules of the Game
Cloud security today is too complicated to fall on the shoulders of one person
or party. For this reason, most cloud services operate on a shared
responsibility model that divvies security roles between the CSP and the
customer. Large players in this space, such as AWS and Microsoft Azure, have
even published frameworks to the lines of liability in the sand. While the
exact delineations can change depending on the service model ... However,
while the expectations laid out in shared responsibility models are designed
to reduce confusion, customers often struggle to conceptualize what this
framework looks like in practice. And unfortunately, when there’s a lack of
clarity, there’s a window of opportunity for threat actors. ... The best-case
scenario for mitigating cloud security risks is when CSPs and customers are
transparent and aligned on their responsibilities right from the beginning.
Even the most secure cloud services aren’t foolproof, so customers need to be
aware of what security elements they’re “owning” versus what falls in the
court of their CSP.
AI's new frontier: bringing intelligence to the data source
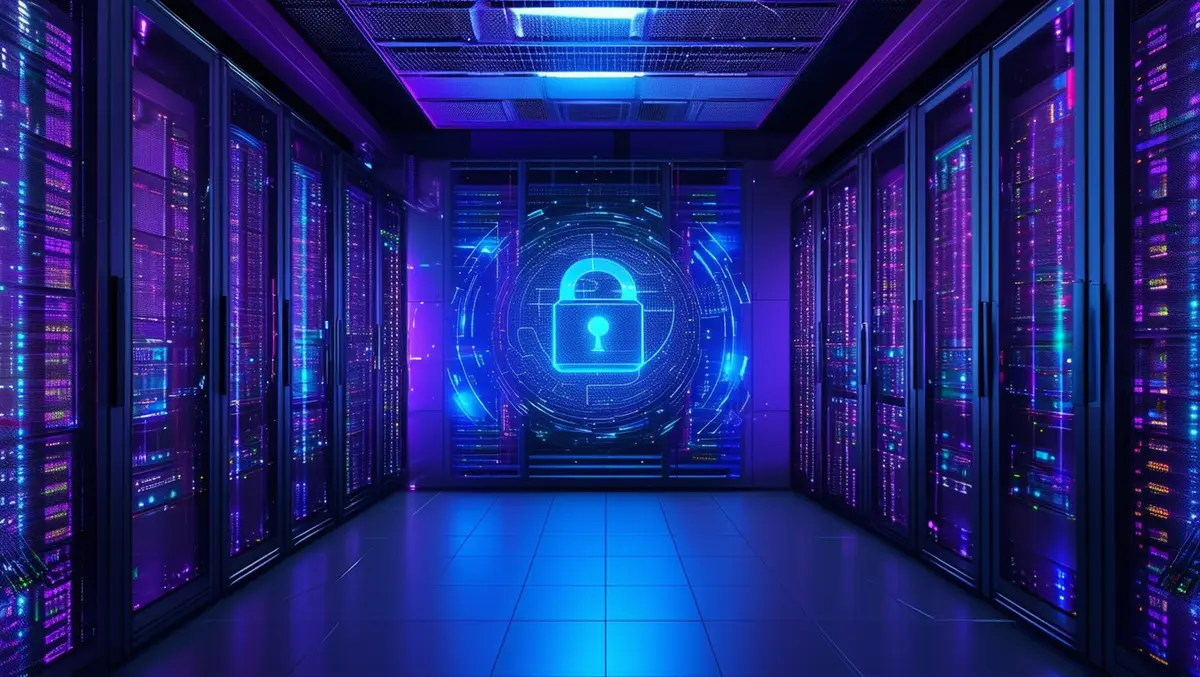
There has been a shift with organisations exploring how to bring AI to their
data rather than uploading proprietary data to AI providers. This shift
reflects a growing concern for data privacy and the desire to maintain control
over proprietary information. Business leaders believe they can better manage
security and privacy while still benefiting from AI advancements by keeping
data in-house. Bringing AI solutions directly to an organisation’s data
eliminates the need to move vast amounts of data, reducing security risks and
maintaining data integrity. Crucially, organisations can maintain strict
control over their data by implementing AI solutions within their own
infrastructure to ensure that sensitive information remains protected and
complies with privacy regulations. Additionally, keeping data in-house
minimises the risks associated with data breaches and unauthorised access from
third parties, providing peace of mind for both the organisation and its
clients. Advanced AI-driven data management tools deliver this solution to
businesses, automating data cleaning, validation, and transformation processes
to ensure high-quality data for AI training.
How AI helps decode cybercriminal strategies
The biggest use case for AI is its ability to process, analyze, and interpret
natural language communication efficiently. AI algorithms can quickly identify
patterns, correlations, and anomalies within massive datasets, providing
cybersecurity professionals with actionable insights. This capability not only
enhances the speed and accuracy of threat detection but also enables a more
proactive and comprehensive approach to securing organizations against dark
web-originated threats. This is vital in an environment where the difference
between detecting a threat early in the cyber kill chain vs once the attacker
has achieved their objective can be hundreds of thousands of dollars. ...
Another potential use case of
AI
is in quickly identifying and alerting specific threats relating to an
organization, helping with the prioritization of intelligence. One thing an AI
could look for in data is intention – to assess whether an actor is planning an
attack, is asking for advice, is looking to buy or to sell access or tooling.
Each of these indicates a different level of risk for the organization, which
can inform security operations.
Widely Used RADIUS Authentication Flaw Enables MITM Attacks
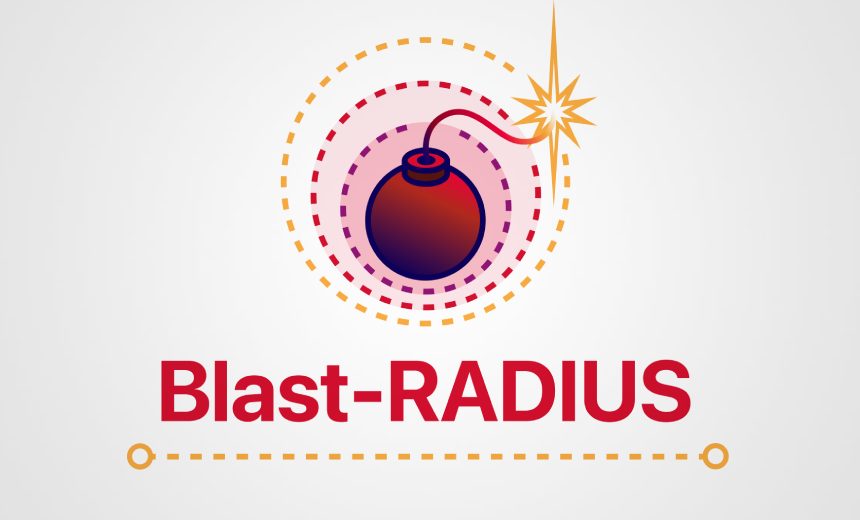
The attack scenario - researchers say a "a well-resourced attacker" could make
it practical - fools the Remote Authentication Dial-In User Service into
granting access to a malicious user without the attacker having to know or guess
a login password. Despite its 1990s heritage and reliance on the MD5 hashing
algorithm, many large enterprises still use the RADIUS protocol for
authentication to the VPN or Wi-Fi network. It's also "universally supported as
an access control method for routers, switches and other network
infrastructure," researchers said in a paper published Tuesday. The protocol is
used to safeguard industrial control systems and 5G cellular networks. ... For
the attack to succeed, the hacker must calculate a MD5 collision within the
client session timeout, where the common defaults are either 30 seconds or 60
seconds. The 60-second default is typically for users that have enabled
multifactor authentication. That's too fast for the researchers, who were able
to reduce the compute time down to minutes from hours, but not down to seconds.
An attacker working with better hardware or cloud computing resources might do
better, they said.
Can RAG solve generative AI’s problems?
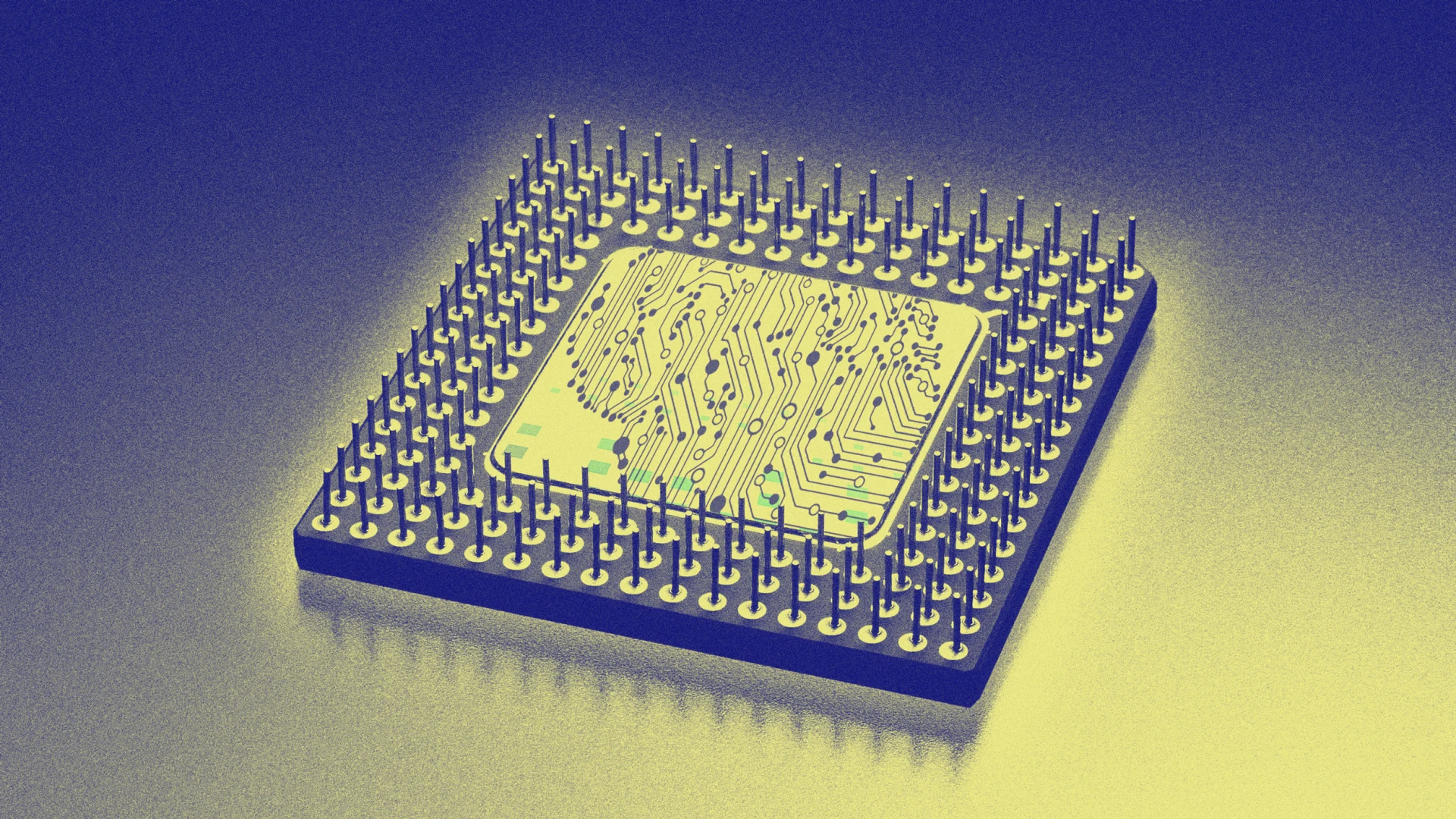
Currently, RAG offers probably the most effective way to enrich LLMs with novel
and domain-specific data. This challenge is particularly important for such
systems as chatbots, since the information they generate must be up to date.
However, RAG cannot reason iteratively, which means it is still dependent on the
underlying dataset (knowledge base, in RAG’s case). Even though this dataset is
dynamically updated, if the information there isn’t coherent or is poorly
categorized and labeled, the RAG model won’t be able to understand that the
retrieval data is irrelevant, incomplete, or erroneous. It would also be naive
to expect RAG to solve the AI hallucination problem. Generative AI algorithms
are statistical black boxes, meaning that developers do not always know why the
model hallucinates and whether it is caused by insufficient or conflicting data.
Moreover, dynamic data retrieval from external sources does not guarantee there
are no inherent biases or disinformation in this data. ... Therefore, RAG is in
no way a definitive solution. In the case of sensitive industries, such as
healthcare, law enforcement, or finance, fine-tuning LLMs with thoroughly
cleaned, domain-specific datasets might be a more reliable option.
Navigating the New Data Norms with Ethical Guardrails for Ethical AI
/dq/media/media_files/tXFNhWOdVvGImBv4k4us.jpg)
To convert ethical principles into a practical roadmap, businesses need a clear
framework aligned with industry standards and company values. Also, beyond
integrity and fairness, businesses must demonstrate tangible ROI by focusing on
metrics like customer acquisition cost, lifetime value, and employee engagement.
Operationalizing ethical guardrails involves creating a structured approach to
ensure AI deployment aligns with ethical standards. Companies can start by
fostering a culture of ethics through comprehensive employee education programs
that emphasize the importance of fairness, transparency, and accountability.
Establishing clear policies and guidelines is crucial, alongside implementing
robust risk assessment frameworks to identify and mitigate potential ethical
issues. Regular audits and continuous monitoring should be part of the process
to ensure adherence to these standards. Additionally, maintaining transparency
for end-users by openly sharing how AI systems make decisions, and providing
mechanisms for feedback, further strengthens trust and accountability.
How CIOs Should Approach DevOps
CIOs should have a vision for scaling DevOps across the enterprise for unlocking
its full range of benefits. A collaborative culture, automation, and technical
skills are all necessary for achieving scale. Besides these, the CIO needs to
think about the right team structure, security landscape, and technical tools
that will take DevOps safely from pilot to production to enterprise scale. It is
recommended to start small: dedicate a small platform team focused only on
building a platform that enables automation of various development tasks. Build
the platform in small steps, incrementally and iteratively. Put together another
small team with all the skills required to deliver value to customers.
Constantly gather customer feedback and incorporate it to improve development at
every stage. Ultimately, customer satisfaction is what matters the most in any
DevOps program. Security needs to be part of every DevOps process right from the
start. When a process is automated, so should its security and compliance
aspects. Frequent code reviews and building awareness among all the concerned
teams will help to create secure, resilient applications that can be scaled with
confidence.
Quote for the day:
“There is no failure except in no
longer trying.” -- Chris Bradford
No comments:
Post a Comment