Superintelligent AI May Be Impossible to Control; That's the Good News

The researchers suggested that any algorithm that sought to ensure a
superintelligent AI cannot harm people had to first simulate the machine’s
behavior to predict the potential consequences of its actions. This
containment algorithm then would need to halt the supersmart machine if it
might indeed do harm. However, the scientists said it was impossible for any
containment algorithm to simulate the AI’s behavior and predict with absolute
certainty whether its actions might lead to harm. The algorithm could fail to
correctly simulate the AI’s behavior or accurately predict the consequences of
the AI’s actions and not recognize such failures. “Asimov’s first law of
robotics has been proved to be incomputable,” Alfonseca says, “and therefore
unfeasible.” We may not even know if we have created a superintelligent
machine, the researchers say. This is a consequence of Rice’s theorem, which
essentially states that one cannot in general figure anything out about what a
computer program might output just by looking at the program, Alfonseca
explains. On the other hand, there’s no need to spruce up the guest room for
our future robot overlords quite yet. Three important caveats to the research
still leave plenty of uncertainty to the group’s predictions.
Rethinking Active Directory security
A change made within on-premises Active Directory by an attacker can provide
access to much more than just local resources. An attacker, can for example,
make a compromised on-premises user account a member of a Sales group in
Active Directory. This group likely would provide access to on-premises
systems, applications, and critical data. But because Active Directory often
federates with cloud applications via external IDP (e.g., Azure AD), it’s
reasonable to assume that this same change in membership could allow access to
a cloud-based CRM environment (like Salesforce), customer data (hopefully
contained to the breached account, but more likely to the entire
organizational data) and other resources. In many cyberattacks it’s more
complex than the example above, where it’s necessary to gain elevated
privileges via one account only to compromise a second, third, and so on, each
time moving from system to system, or – in the case of a hybrid environment –
from on-premises to cloud, leveraging access to on-premises Active Directory
to specifically target accounts known to have access in the cloud.
The Great Compromise In AI’s Buy Vs Build Dilemma
Building AI in-house presents a variety of benefits. When done right, a built
approach can lead to a stable, production-grade AI solution that is perfectly
tailored to the specific needs and requirements of an industry or
company. Digital natives have shown the impact of building AI from
scratch. IBM is a prominent example of a business that has launched successful
in-house AI into production. A recent report found IBM’s Watson Assistant AI
paid itself back in just 6 months, with a three-year ROI of 337%. For
digital adopters however, successfully building and implementing an AI
solution in house is easier said than done without access to sizable capital
and infrastructure. “When building an AI solution in-house, companies
typically hire a team without significantly investing in the foundational
elements that are required to stabilize AI in complex and dynamic
environments,” suggests Nurit Cohen Inger, VP of Products at AI company
BeyondMinds. “This approach, unfortunately, has typically meant a long and
costly process to reach ROI positivity or in the worst case, never achieving
production. Before developing AI solutions, businesses must heavily invest in
solving the barriers that hold them back from turning proof of concepts into
successful solutions in production.”
Training from the Back of the Room and Systems Thinking in Kanban Workshops
It’s very tempting to put everything you know on a training agenda, especially
when you, as a trainer, feel that you have to know everything and constantly
impress the learners. It’s always hard to chop workshop content into the bare
minimum, especially when you have a lot of knowledge, experience, and fun
stories to share. But if you are aiming for deep understanding and a lot of
practice, less content translates into more value. Overloading groups with new
information may lead to chaos during your class. They will struggle to
understand which new tool or technique they should use first. In the end, they
may just quit before they even start. ... Training From the Back of the Room
(TBR) is a fresh approach to learning, training, presenting and facilitating
that was developed by Sharon Bowman. It uses cognitive neuroscience and
brain-based learning techniques to help learners to retain new information. TBR
teaches you how to engage the five senses and keeps your learners active and
engaged throughout the class. The concept is recognized internationally as one
of the most effective frameworks for accelerated learning. It is a new way of
teaching adults.
How COVID-19 accelerated a digital revolution in the insurance industry
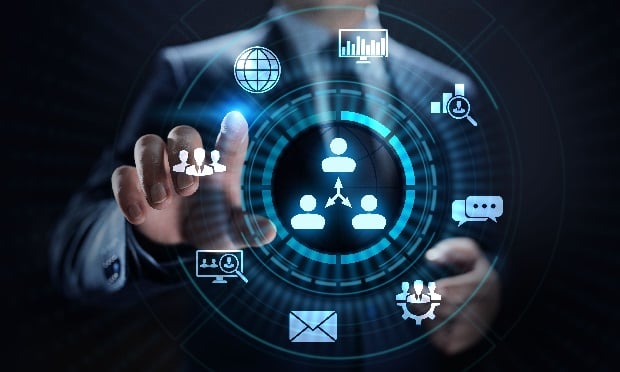
The pandemic reminded us that we’re human. This experience has taught us
compassion, grace, and the importance of both the health and wellbeing of
ourselves and our families. COVID-19 has fundamentally reshaped the way we
view protection products. In fact, two thirds (66%) of Americans say they now
better understand life insurance’s value, with another quarter buying coverage
for the first time. Awareness around the role of employers in providing access
to these products has also increased. In a recent LIMRA study, one in four
employees said they are more likely to sign up for certain benefits available
through their employer. Along with this heightened awareness of our mortality
and morbidity comes the realization that we thrive on human interaction. We
can’t take a digital-only approach. Bringing emotion—positive emotion and
empathy—to the experience and every interaction we have with customers will
help us get farther, faster. As we continue to invest in technology across the
insurance industry, we need to look for ways to make digital and human
experiences work together for customers, employers, and financial
professionals. Many of our customers tell us they don’t understand insurance
products and they don’t know where to start educating themselves.
7 Blindspots You Need to Uncover to Achieve Digital Banking Breakthrough

To explain the way that the “experience gap” might cause trouble, I'd like to
share a real-life example. Several years ago a quite known and respectable
Central European bank embarked on a voluminous digital transformation journey.
The bank's application had a rating of 3.5 and was outdated. In order to
digitalize, improve the bank's image and the competitive chances in the
growing digital market, the management intended to urgently create and launch
a modern looking banking application. Therefore, the initial design and
development period was 6 months. Nevertheless, the bank spent three times as
much time building the new application by themselves: 1 year and 8 months.
This was a serious project not only in terms of time but also the budget
invested. Judging by the scope of the project, the improvements made and the
timeline, the overall costs could be estimated at around half a million.
However, the result did not live up to expectations at all. After the new
application was released it decreased to 2.4 from the previous 3.5 and has
kept dropping even a year after its first release as it did not improve, but
significantly worsened the customer experience.
Riding out the wave of disruption
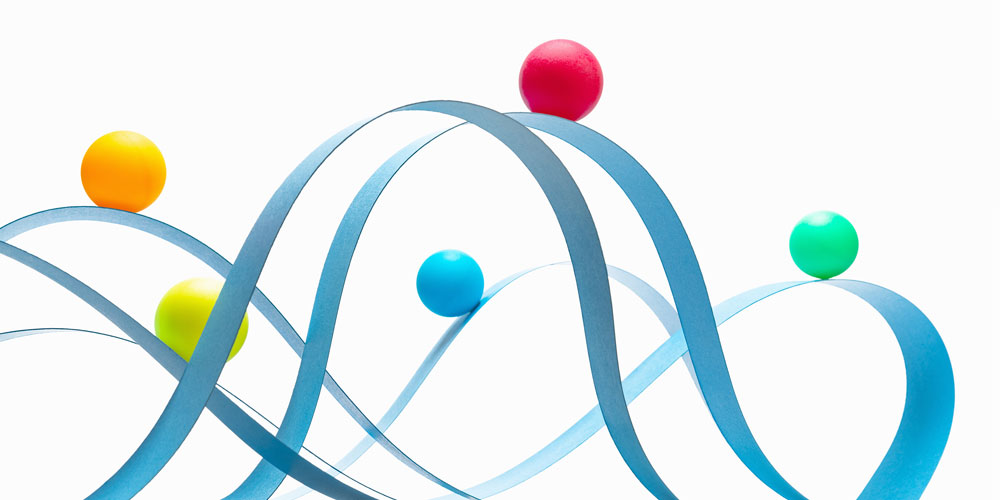
Disruption is not necessarily the crisis it’s frequently considered to be for
incumbents, the researchers stress. Two technologies can often coexist in the
marketplace for a significant period. Thus, it’s important for incumbent
companies not to overreact. They should target dual users and reexamine the
factors that have led to the old technology sticking around for so long. Of
course, the profit implications of cannibalization of the old technology and
leapfrogging depend on which type of firm is trumpeting the new technology.
New entrants will always stand to gain when they introduce a technology that
takes off. But incumbents rolling out a successive technology will also gain
if their competitors would have introduced it anyway or if the 2.0 version has
a higher profit margin than the original. The authors write, “Leapfroggers are
an opportunity loss for incumbents, but switchers are a real loss.” Regardless
of the predictive model they use, marketers should strive to understand how
the various consumer segments identified in this study will grow or shrink
over time and use that information in their forecasts of early sales or market
penetration of successive technologies.
Understanding the AI alignment problem

What’s worse is that machine learning models can’t tell right from wrong and
make moral decisions. Whatever problem exists in a machine learning model’s
training data will be reflected in the model’s behavior, often in nuanced and
inconspicuous ways. For instance, in 2018, Amazon shut down a machine learning
tool used in making hiring decisions because its decisions were biased against
women. Obviously, none of the AI’s creators wanted the model to select
candidates based on their gender. In this case, the model, which was trained
on the company’s historical hiring data, reflected problems within Amazon
itself. This is just one of the several cases where a machine learning model
has picked up biases that existed in its training data and amplified them in
its own unique ways. It is also a warning against trusting machine learning
models that are trained on data we blindly collect from our own past behavior.
“Modeling the world as it is is one thing. But as soon as you begin using that
model, you are changing the world, in ways large and small. There is a broad
assumption underlying many machine-learning models that the model itself will
not change the reality it’s modeling. In almost all cases, this is false,”
Christian writes.
Fixing the cracks in public sector digital infrastructure
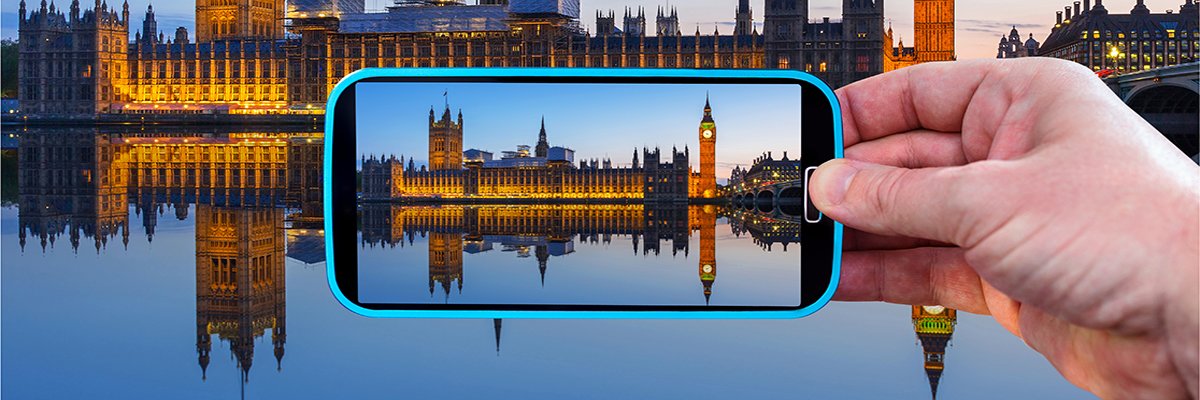
First, there needs to be a government-wide, comprehensive digital skills
strategy. One survey of industry professionals found that 40% of public sector
organisations did not have the right skills to carry out digital
transformation. Every member of the workforce needs to be able to perform
basic tasks online. But to press forward with digital transformation, the
government needs to champion digital leadership in the public sector – and
that includes paying properly for those skills. The Government Digital Service
recently advertised for a head of technology and architecture with a maximum
salary of £70,887 a year. According to Google Jobs, typical pay for this type
of work ranges from £65,000 to £180,000 in the private sector. This puts the
public sector at a unique disadvantage and pay scales should be reviewed. ...
Second, the Cabinet Office needs to address the gap between guidance and
action on the ground. Out-of-date technology is widespread in some areas of
the public sector, despite there being a large volume of information from
central government on maintaining and updating digital
infrastructure. Legacy IT has been holding digital public services back
for years and will continue to do so unless there is a cross-government push
to drive this forward.
Emotion Detection in Tech: It’s Complicated
Emotion detection would be a lot easier if humans expressed themselves in
homogenous ways. However, cultural backgrounds and unique life experiences
influence personal expression. Michelle Niedziela, VP of research and innovation
at market research firm HCD Research, said advertisers and their agencies can
get overly excited about the "happy" responses an ad drives when the response
may have been a natural reflex. "If I smile at you, you innately smile back. So,
one thing is are they really feeling happy or just projecting happy?" said
Niedziela. "But also, how big does a smile have to be in order to be interpreted
as happy?" Even cheap camera sensors are improving, but some of them may not be
able to detect subtle nuances in facial geometry or provide the same degree of
reliability among individuals who represent different races. Also, things that
change an individual's appearance like hats, bangs or facial hair can negatively
impact the accuracy of emotion sensing. "In my mind, the two biggest challenges
are hardware quality and the models," said Capgemini's Simion. "You need to be
very careful when you're talking about emotionality is the dataset you're going
to use because if you're just going to call normal APIs from the cloud
providers, that's not going to help much."
Quote for the day:
"To do great things is difficult; but to command great things is more difficult." -- Friedrich Nietzsche
No comments:
Post a Comment