Quote for the day:
"If a window of opportunity appears, don't pull down the shade." -- Tom Peters
Researchers Revolutionize Fraud Detection with Machine Learning
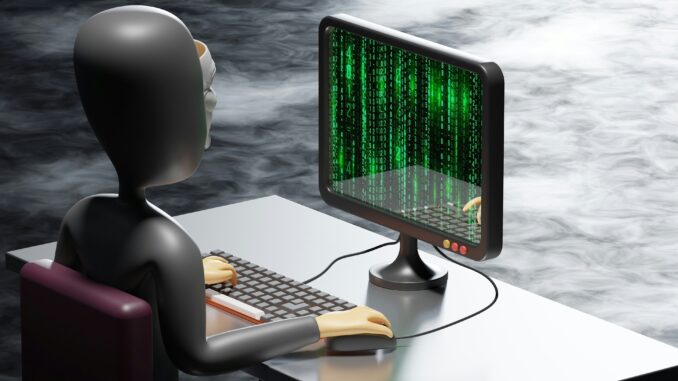
Machine learning plays a critical role in fraud detection by identifying
patterns and anomalies in real-time. It analyzes large datasets to spot normal
behavior and flag significant deviations, such as unusual transactions or
account access. However, fraud detection is challenging because fraud cases
are much rarer than normal ones, and the data is often messy or unlabeled. ...
“The use of machine learning in fraud detection brings many advantages,” said
Taghi Khoshgoftaar, Ph.D., senior author and Motorola Professor in the FAU
Department of Electrical Engineering and Computer Science. “Machine learning
algorithms can label data much faster than human annotation, significantly
improving efficiency. Our method represents a major advancement in fraud
detection, especially in highly imbalanced datasets. It reduces the workload
by minimizing cases that require further inspection, which is crucial in
sectors like Medicare and credit card fraud, where fast data processing is
vital to prevent financial losses and enhance operational efficiency.” ... The
method combines two strategies: an ensemble of three unsupervised learning
techniques using the SciKit-learn library and a percentile-gradient approach.
The goal is to minimize false positives by focusing on the most confidently
identified fraud cases.
Cybersecurity is Not Working: Time to Try Something Else

Many CISOs, changing jobs every 2 years or so, have not learnt to get things
done in large firms; they have not developed the political acumen and the
management experience they would need. Many have simply remained technologists
and firefighters, trapped in an increasingly obsolete mindset, pushing
bottom-up a tools-based, risk-based, tech-driven narrative, disconnected from
what the board wants to hear which has now shifted towards resilience and
execution. This is why we may have to come to the point where we have to
accept that the construction around the role of the CISO, as it was initiated
in the late 90s, has served its purpose and needs to evolve. The first step in
this evolution, in my opinion, is for the board to own cybersecurity as a
business problem, not as a technology problem. It needs to be owned at board
level in business terms, in line with the way other topics are owned at board
level. This is about thinking the protection of the business in business
terms, not in technology terms. Cybersecurity is not a purely technological
matter; it has never been and cannot be. ... There may be a need to amalgamate
it with other matters such as corporate resilience, business continuity or
data privacy to build up a suitable board-level portfolio, but for me this is
the way forward in reversing the long-term dynamics, away from the failed
historical bottom-up constructions, towards a progressive top-down
approach.
How the financial services C-suite are going beyond ‘keeping the lights on’ in 2025
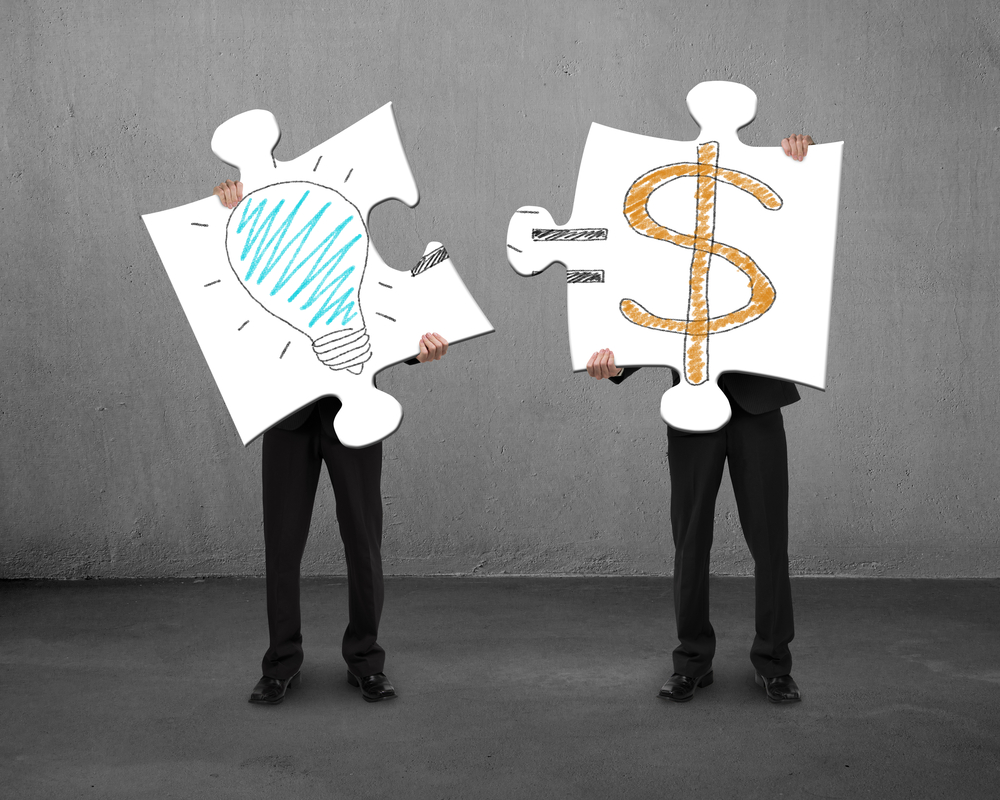
C-suites need to tackle three core areas: Ensure they are getting high value
support for their mission-critical systems; Know how to optimise their
investments; Transform their organisation without disrupting day-to-day
operations. It is certainly possible if they have the time, capabilities and
skill sets in-house. Yet even the most well-resourced enterprises can struggle
to acquire the knowledge base and market expertise required to negotiate with
multiple vendors, unlock investments or run complex change programmes
single-handedly. The reality is that managing the balance needed to save costs
while accelerating innovation is challenging. ... The survey demonstrates a
growing necessity for CIOs and CFOs to speak each other’s language, marking a
shift in organisational strategy, moving IT beyond the traditional ‘keeping the
lights on’ approach, and driving a pivotal transformation in the relationship
between CIOs and CFOs. As they find better ways to collaborate and innovate,
businesses in the financial services space will reap the rewards of emerging
technology, while falling in line with budgetary needs. Emerging technologies
are being introduced thick and fast, and as a result, hard metrics aren’t always
available. Instead of feeling frustrated with a lack of data, CFOs should lean
in as active participants, understanding how emerging technologies like AI and
cybersecurity can drive strategic value, optimise operations and create new
revenue streams.
Threat actors are scanning your environment, even if you’re not
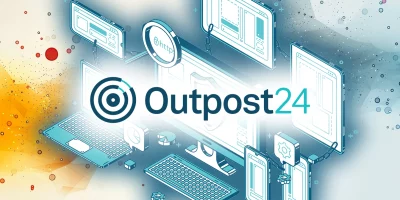
Martin Jartelius, CISO and Product Owner at Outpost24, says that the most common
blind spots that the solution uncovers are exposed management interfaces of
devices, exposed databases in the cloud, misconfigured S3 storage, or just a
range of older infrastructure no longer in use but still connected to the
organization’s domain. All of these can provide an entry point into internal
networks, and some can be used to impersonate organizations in targeted phishing
attacks. But these blind spots are not indicative of poor leadership or IT
security performance: “Most who see a comprehensive report of their attack
surface for the first time are surprised that it is often substantially larger
than they understood. Some react with discomfort and perceive their prior lack
of insight as a failure, but that is not the case. ... Attack surface management
is still a maturing technology field, but having a solution bringing the
information together in a platform gives a more refined and in-depth insight
over time. External attack surface management starts with a continuous detection
of exposed assets – in Sweepatic’s case, that also includes advanced port
scanning to detect all (and not just the most common) ports at risk of
exploitation – then moves on to automated security analysis and then risk-based
reporting.
How to Run a Generative AI Developer Tooling Experiment

The last metric, rework, is a significant consideration with generative AI, as
67% of developers find that they are spending more time debugging AI-generated
code. Devexperts experienced a 200% increase in rework and a 30% increase in
maintenance. On the other hand, while the majority of organizations are seeing
an increase in complexity and lines of code with code generators, these five
engineers saw a surprising 15% decrease in lines of code created. “We can
conclude that, for the live experiment, GitHub Copilot didn’t deliver the
results one could expect after reading their articles,” summarized German
Tebiev, the software engineering process architect who ran the experiment. He
did think the results were persuasive enough to believe speed will be enabled if
the right processes are put in place: “The fact that the PR throughput shows
significant growth tells us that the desired speed increase can be achieved if
the tasks’ flow is handled effectively.” ... Just 17% of developers responded
that they think Copilot helped them save at least an hour a week, versus a
whopping 40% saw no time savings by using the code generator, which is well
below the industry average. Developers were also able to share their own
anecdotal experience, which is very situation-dependent. Copilot seemed to be a
better choice for completing more basic lines of code for new features, less so
when there’s complexity of working with an existing codebase.
Dark Data: Surprising Places for Business Insights
Previously, the biggest problem when dealing with dark data was its messy
nature. Even though AI has been able to analyze structured data for years,
unstructured or semi-structured data proved to be a hard nut to crack.
Unfortunately, unstructured data constitutes the majority of dark data. However,
recent advances in natural language programming (NLP), natural language
understanding (NLU), speech recognition, and ML have enabled AI to deal with
unstructured dark data more effectively. Today, AI can easily analyze raw inputs
like customer reviews, social media comments to identify trends and sentiment.
Advanced sentiment analysis algorithms can come to accurate conclusions when
concerning tone, context, emotional nuances, sarcasm, and urgency, providing
businesses with deeper audience insights. For instance, Amazon uses this
approach to flag fake reviews. In finance and banking, AI-powered data analysis
tools are used to process transaction logs and unstructured customer
communications to identify fraud risks and enhance service and customer
satisfaction. Another industry where dark data mining might have potentially
huge social benefits is healthcare. Currently, this industry generates around
30% of all the data in the world.
Is your AI product actually working? How to develop the right metric system

Not tracking whether your product is working well is like landing a plane
without any instructions from air traffic control. There is absolutely no way
that you can make informed decisions for your customer without knowing what is
going right or wrong. Additionally, if you do not actively define the metrics,
your team will identify their own back-up metrics. The risk of having multiple
flavors of an ‘accuracy’ or ‘quality’ metric is that everyone will develop their
own version, leading to a scenario where you might not all be working toward the
same outcome. ... the complexity of operating an ML product with multiple
customers translates to defining metrics for the model, too. What do I use to
measure whether a model is working well? Measuring the outcome of internal teams
to prioritize launches based on our models would not be quick enough; measuring
whether the customer adopted solutions recommended by our model could risk us
drawing conclusions from a very broad adoption metric ... Most metrics are
gathered at-scale by new instrumentation via data engineering. However, in some
instances (like question 3 above) especially for ML based products, you have the
option of manual or automated evaluations that assess the model outputs.
Security needs to be planned and discussed early, right at the product ideation stage
On the open-source side, where a lot of supply chain risks emerge, we leverage a
state-of-the-art development pipeline. Developers follow strict security
guidelines and use frameworks embedded with security tools that detect risks
associated with third-party libraries—both during development and at runtime. We
also have robust monitoring systems in place to detect vulnerabilities and
active exploits. ... Monitoring technological events is one part, but from a
core product perspective, we also need to monitor risk-based activities, like
transactions that could potentially lead to fraud. For that, we have strong
AI/ML developments already deployed, with a dedicated AI and data science team
constantly building new algorithms to detect fraudulent actions. From a product
standpoint, the system is quite mature. On the technology side, monitoring has
some automation powered by AI, and we’ve also integrated tools like GitHub
Copilot. Our analysts, developers, and security engineers use these technologies
to quickly identify potential issues, reducing manual effort significantly. ...
Security needs to be planned and discussed early—right at the product ideation
stage with product managers—so that it doesn’t become a blocker at the end.
Early involvement makes it much easier and avoids last-minute disruptions.
14 tiny tricks for big cloud savings

Good algorithms can boost the size of your machine when demand peaks. But clouds
don’t always make it easy to shrink all the resources on disk. If your disks
grow, they can be hard to shrink. By monitoring these machines closely, you can
ensure that your cloud instances consume only as much as they need and no more.
... Cloud providers can offer significant discounts for organizations that make
a long-term commitment to using hardware. These are sometimes called reserved
instances, or usage-based discounts. They can be ideal when you know just how
much you’ll need for the next few years. The downside is that the commitment
locks in both sides of the deal. You can’t just shut down machines in slack
times or when a project is canceled. ... Programmers like to keep data around in
case they might ever need it again. That’s a good habit until your app starts
scaling and it’s repeated a bazillion times. If you don’t call the user, do you
really need to store their telephone number? Tossing personal data aside not
only saves storage fees but limits the danger of releasing personally
identifiable information. Stop keeping extra log files or backups of data that
you’ll never use again. ... Cutting back on some services will save money, but
the best way to save cash is to go cold turkey. There’s nothing stopping you
from dumping your data into a hard disk on your desk or down the hall in a local
data center.
Two-thirds of jobs will be impacted by AI

“Most jobs will change dramatically in the next three to four years, at least as
much as the internet has changed jobs over the last 30,” Calhoon said. “Every
job posted on Indeed today, from truck driver to physician to software engineer,
will face some level of exposure to genAI-driven change.” ... What will emerge
is a “symbiotic” relationship with an increasingly “proactive” technology that
will require employees to constantly learn new skills and adapt. “AI can manage
repetitive tasks, or even difficult tasks that are specific in nature, while
humans can focus on innovative and strategic initiatives that drive revenue
growth and improve overall business performance,” Hoffman said in an interview
earlier this year. “AI is also much quicker than humans could possibly be, is
available 24/7, and can be scaled to handle increasing workloads.” As AI takes
over repetitive tasks, workers will shift toward roles that involve overseeing
AI, solving unique problems, and applying creativity and strategy. Teams will
increasingly collaborate with AI—like marketers personalizing content or
developers using AI copilots. Rather than replacing humans, AI will enhance
human strengths such as decision-making and emotional intelligence. Adapting to
this change will require ongoing learning and a fresh approach to how work is
done.
No comments:
Post a Comment