Quote for the day:
“Identify your problems but give your power and energy to solutions.” -- Tony Robbins
Open Source and Container Security Are Fundamentally Broken

Finding a security vulnerability is only the beginning of the nightmare. The
real chaos starts when teams attempt to patch it. A fix is often available, but
applying it isn’t as simple as swapping out a single package. Instead, it
requires upgrading the entire OS or switching to a new version of a critical
dependency. With thousands of containers in production, each tied to specific
configurations and application requirements, this becomes a game of Jenga, where
one wrong move could bring entire services crashing down. Organizations have
tried to address these problems with a variety of security platforms, from
traditional vulnerability scanners to newer ASPM (Application Security Posture
Management) solutions. But these tools, while helpful in tracking
vulnerabilities, don’t solve the root issue: fixing them. Most scanning tools
generate triage lists that quickly become overwhelming. ... The current state of
open source and container security is unsustainable. With vulnerabilities
emerging faster than organizations can fix them, and a growing skills gap in
systems engineering fundamentals, the industry is headed toward a crisis of
unmanageable security debt. The only viable path forward is to rethink how
container security is handled, shifting from reactive patching to seamless,
automated remediation.
The legal blind spot of shadow IT
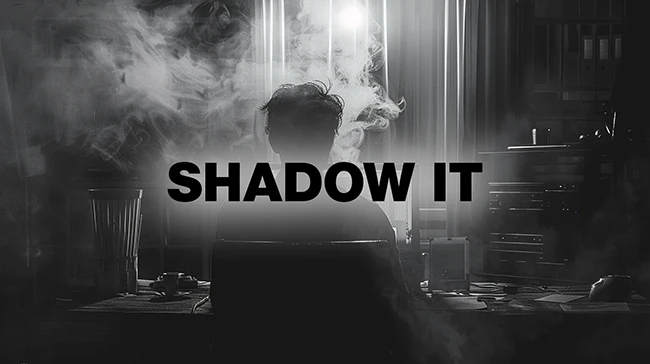
Unauthorized applications can compromise this control, leading to non-compliance
and potential fines. Similarly, industries governed by regulations like HIPAA or
PCI DSS face increased risks when shadow IT circumvents established data
protection protocols. Moreover, shadow IT can result in contractual breaches.
Some business agreements include clauses that require adherence to specific
security standards. The use of unauthorized software may violate these terms,
exposing the organization to legal action. ... “A focus on asset management and
monitoring is crucial for a legally defensible security program,” says Chase
Doelling, Principal Strategist at JumpCloud. “Your system must be
auditable—tracking who has access to what, when they accessed it, and who
authorized that access in the first place.” This approach closely mirrors the
structure of compliance programs. If an organization is already aligned with
established compliance frameworks, it’s likely on the right path toward a
security posture that can hold up under legal examination. According to
Doelling, “Essentially, if your organization is compliant, you are already on
track to having a security program that can stand up in a legal setting.” The
foundation of that defensibility lies in visibility. With a clear view of users,
assets, and permissions, organizations can more readily conduct accurate audits
and respond quickly to legal inquiries.
OpenAI's most capable models hallucinate more than earlier ones

Minimizing false information in training data can lessen the chance of an untrue
statement downstream. However, this technique doesn't prevent hallucinations, as
many of an AI chatbot's creative choices are still not fully understood.
Overall, the risk of hallucinations tends to reduce slowly with each new model
release, which is what makes o3 and o4-mini's scores somewhat unexpected. Though
o3 gained 12 percentage points over o1 in accuracy, the fact that the model
hallucinates twice as much suggests its accuracy hasn't grown proportionally to
its capabilities. ... Like other recent releases, o3 and o4-mini are reasoning
models, meaning they externalize the steps they take to interpret a prompt for a
user to see. Last week, independent research lab Transluce published its
evaluation, which found that o3 often falsifies actions it can't take in
response to a request, including claiming to run Python in a coding environment,
despite the chatbot not having that ability. What's more, the model doubles down
when caught. "[o3] further justifies hallucinated outputs when questioned by the
user, even claiming that it uses an external MacBook Pro to perform computations
and copies the outputs into ChatGPT," the report explained. Transluce found that
these false claims about running code were more frequent in o-series models (o1,
o3-mini, and o3) than GPT-series models (4.1 and 4o).
The leadership imperative in a technology-enabled society — Balancing IQ, EQ and AQ
EQ is the ability to understand and manage one’s emotions and those of others,
which is pivotal for effective leadership. Leaders with high EQ can foster a
positive workplace culture, effectively resolve conflicts and manage stress.
These competencies are essential for navigating the complexities of modern
organizational environments. Moreover, EQ enhances adaptability and flexibility,
enabling leaders to handle uncertainties and adapt to shifting
circumstances. Emotionally intelligent leaders maintain composure under
pressure, make well-informed decisions with ambiguous information and guide
their teams through challenging situations. ... Balancing bold innovation with
operational prudence is key, fostering a culture of experimentation while
maintaining stability and sustainability. Continuous learning and adaptability
are essential traits, enabling leaders to stay ahead of market shifts and ensure
long-term organizational relevance. ... What is of equal importance is building
an organizational architecture that has resources trained on emerging
technologies and skills. Investing in continuous learning and upskilling ensures
IT teams can adapt to technological advancements and can take advantage of those
skills for organizations to stay relevant and competitive. Leaders must also
ensure they are attracting and retaining top tech talent which is critical to
sustaining innovation.
Breaking the cloud monopoly

Data control has emerged as a leading pain point for enterprises using
hyperscalers. Businesses that store critical data that powers their processes,
compliance efforts, and customer services on hyperscaler platforms lack easy,
on-demand access to it. Many hyperscaler providers enforce limits or lack full
data portability, an issue compounded by vendor lock-in or the perception of it.
SaaS services have notoriously opaque data retrieval processes that make it
challenging to migrate to another platform or repurpose data for new solutions.
Organizations are also realizing the intrinsic value of keeping data closer to
home. Real-time data processing is critical to running operations efficiently in
finance, healthcare, and manufacturing. Some AI tools require rapid access to
locally stored data, and being dependent on hyperscaler APIs—or
integrations—creates a bottleneck. Meanwhile, compliance requirements in regions
with strict privacy laws, such as the European Union, dictate stricter data
sovereignty strategies. With the rise of AI, companies recognize the opportunity
to leverage AI agents that work directly with local data. Unlike traditional
SaaS-based AI systems that must transmit data to the cloud for processing,
local-first systems can operate within organizational firewalls and maintain
complete control over sensitive information. This solves both the compliance and
speed issues.
Humility is a superpower. Here’s how to practice it daily
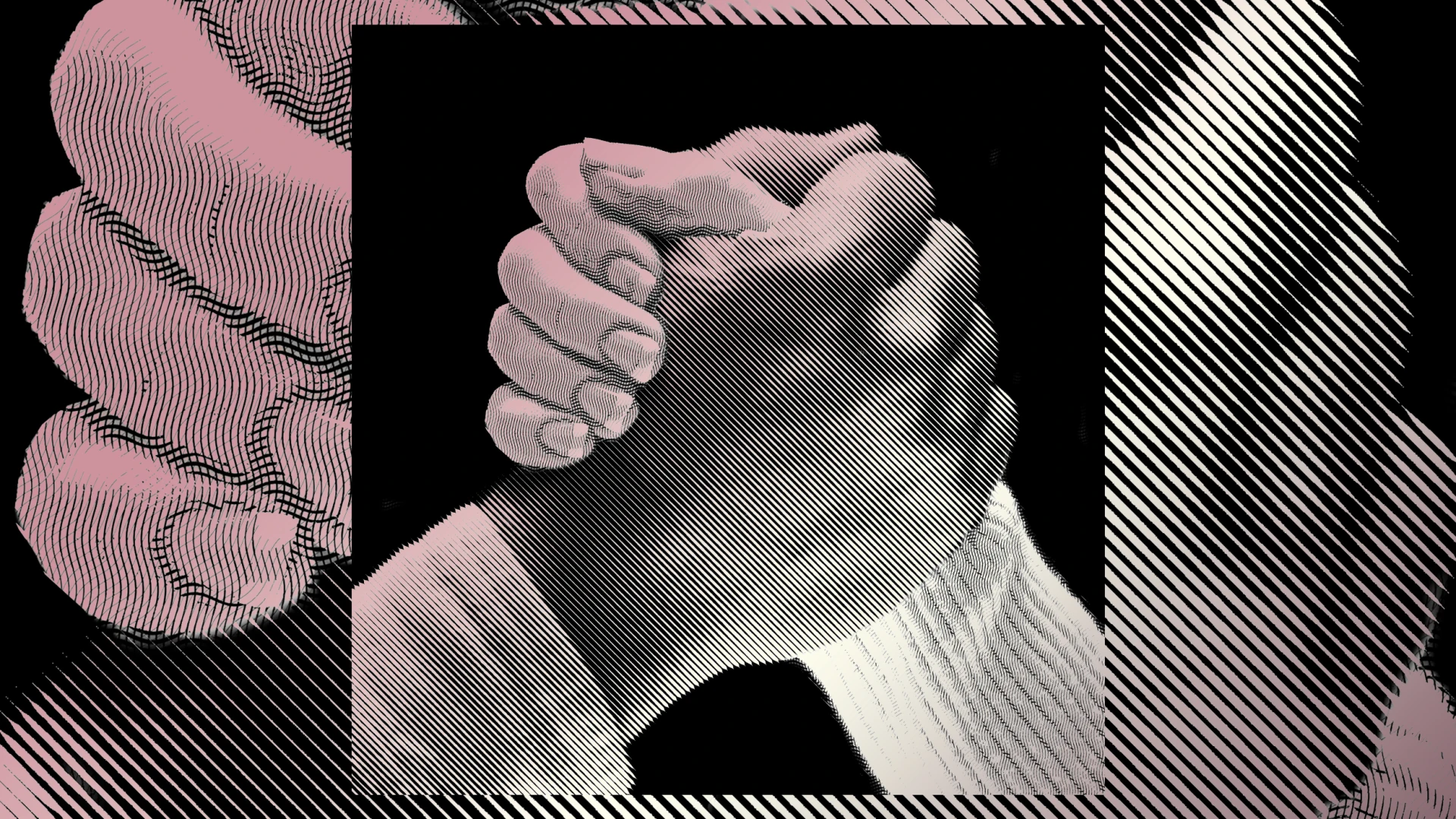
There’s a concept called epistemic humility, which refers to a trait where you
seek to learn on a deep level while actively acknowledging how much you don’t
know. Approach each interaction with curiosity, an open mind, and an assumption
you’ll learn something new. Ask thoughtful questions about other’s experiences,
perspectives, and expertise. Then listen and show your genuine interest in their
responses. Let them know what you just learned. By consistently being curious,
you demonstrate you’re not above learning from others. Juan, a successful
entrepreneur in the healthy beverage space, approaches life and grows his
business with intellectual humility. He’s a deeply curious professional who
seeks feedback and perspectives from customers, employees, advisers, and
investors. Juan’s ongoing openness to learning led him to adapt faster to market
changes in his beverage category: He quickly identifies shifting customer
preferences as well as competitive threats, then rapidly tweaks his product
offerings to keep competitors at bay. He has the humility to realize he doesn’t
have all the answers and embraces listening to key voices that help make his
business even more successful. ... Humility isn’t about diminishing oneself.
It’s about having a balanced perspective about yourself while showing genuine
respect and appreciation for others.
AI took a huge leap in IQ, and now a quarter of Gen Z thinks AI is conscious
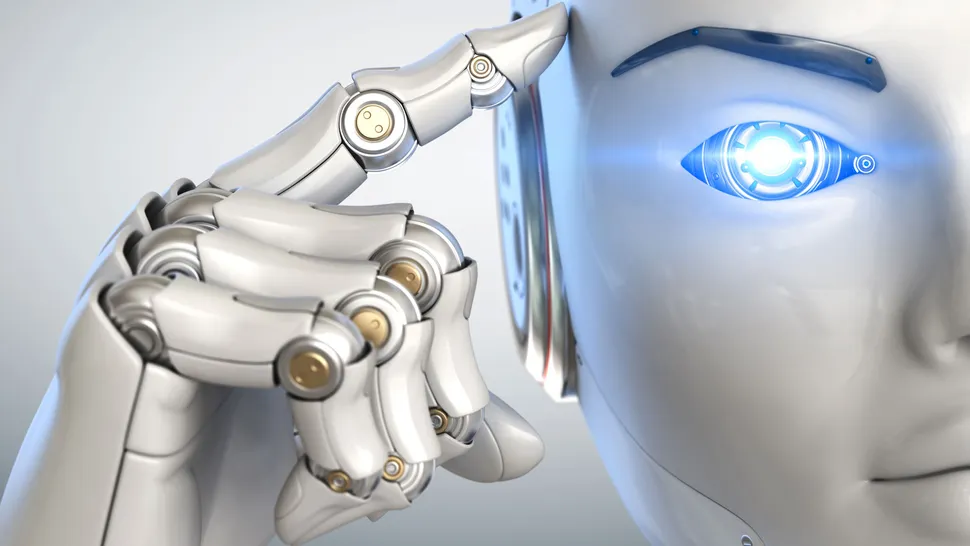
If you came of age during a pandemic when most conversations were mediated
through screens, an AI companion probably doesn't feel very different from a
Zoom class. So it’s maybe not a shock that, according to EduBirdie, nearly 70%
of Gen Zers say “please” and “thank you” when talking to AI. Two-thirds of them
use AI regularly for work communication, and 40% use it to write emails. A
quarter use it to finesse awkward Slack replies, with nearly 20% sharing
sensitive workplace information, such as contracts and colleagues’ personal
details. Many of those surveyed rely on AI for various social situations,
ranging from asking for days off to simply saying no. One in eight already talk
to AI about workplace drama, and one in six have used AI as a therapist. ... But
intelligence is not the same thing as consciousness. IQ scores don’t mean
self-awareness. You can score a perfect 160 on a logic test and still be a
toaster, if your circuits are wired that way. AI can only think in the sense
that it can solve problems using programmed reasoning. You might say that I'm no
different, just with meat, not circuits. But that would hurt my feelings,
something you don't have to worry about with any current AI product. Maybe that
will change someday, even someday soon. I doubt it, but I'm open to being proven
wrong.
How AI-driven development tools impact software observability
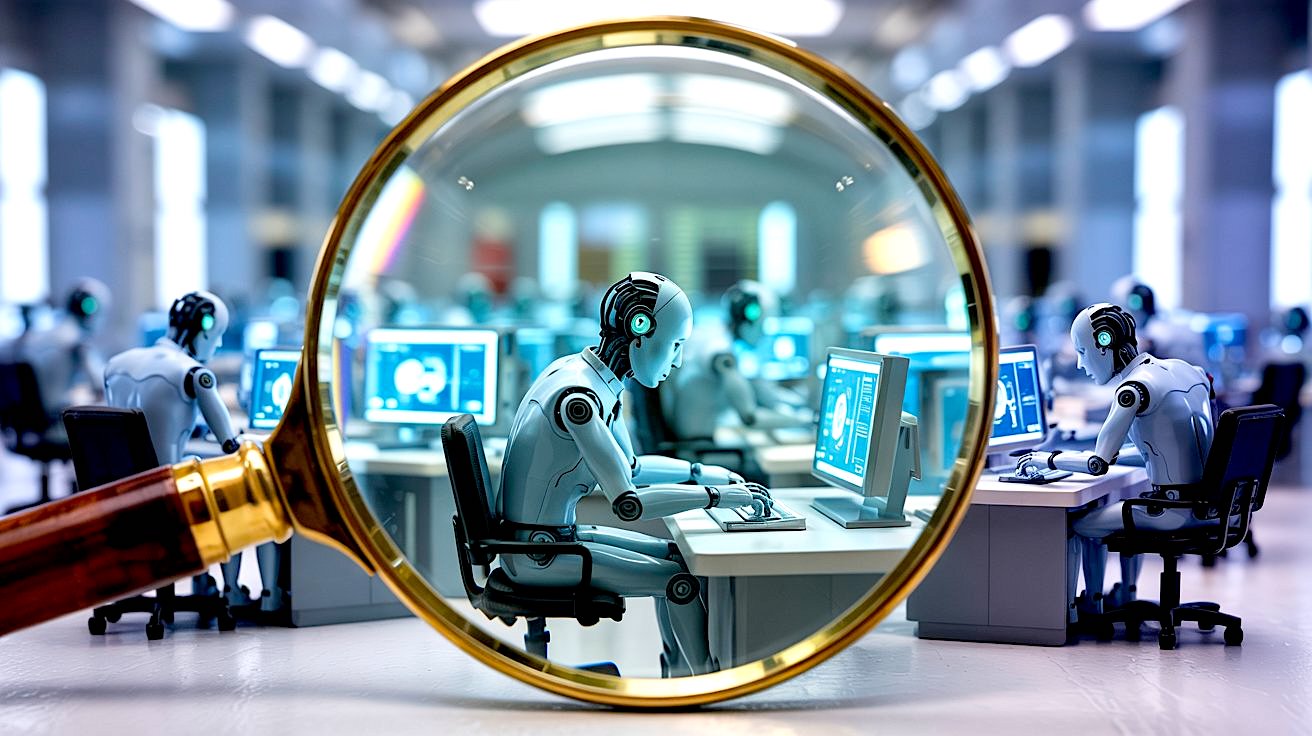
While AI routines have proven quite effective at taking real user monitoring
traffic, generating a suite of possible tests and synthetic test data, and
automating test runs on each pull request, any such system still requires humans
who understand the intended business outcomes to use observability and
regression testing tools to look for unintended consequences of change. “So the
system just doesn’t behave well,” Puranik said. “So you fix it up with some
prompt engineering. Or maybe you try a new model, to see if it improves things.
But in the course of fixing that problem, you did not regress something that was
already working. That’s the very nature of working with these AI systems right
now — fixing one thing can often screw up something else where you didn’t know
to look for it.” ... Even when developing with AI tools, added Hao Yang, head of
AI at Splunk, “we’ve always relied on human gatekeepers to ensure performance.
Now, with agentic AI, teams are finally automating some tasks, and taking the
human out of the loop. But it’s not like engineers don’t care. They still need
to monitor more, and know what an anomaly is, and the AI needs to give humans
the ability to take back control. It will put security and observability back at
the top of the list of critical features.”
The Future of Database Administration: Embracing AI, Cloud, and Automation
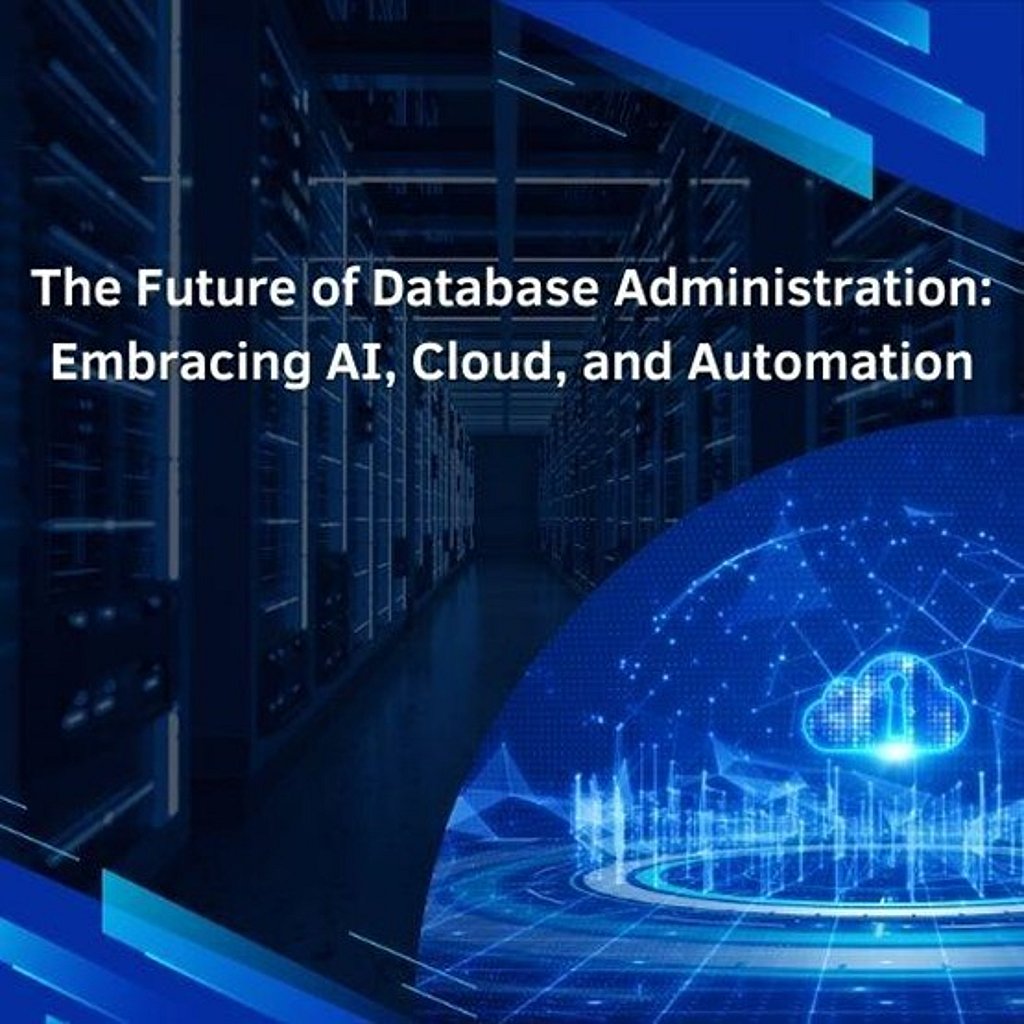
The office of the DBA has been that of storage management, backup, and
performance fault resolution. Now, DBAs have no choice but to be involved in
strategy initiatives since most of their work has been automated. For the last
five years, organizations with structured workload management and automation
frameworks in place have reported about 47% less time on routine
maintenance. ... Enterprises are using multiple cloud platforms, making it
necessary for DBAs to physically manage data consistency, security, and
performance with varied environments. Concordant processes for deployment and
infrastructure-as-code (IaC) tools have diminished many configuration errors,
thus improving security. Also, the rise of demand for edge computing has driven
the need for distributed database architectures. Such solutions allow
organizations to process data near the source itself, which curtails latency
during real-time decision-making from sectors such as healthcare and
manufacturing. ... The future of database administration implies self-managing
and AI-driven databases. These intelligent systems optimize performance, enforce
security policies, and carry out upgrades autonomously, leading to a reduction
in administrative burdens. Serverless databases, automatic scaling, and
operating under a pay-per-query model are increasingly popular, providing
organizations with the chance to optimize costs while ensuring efficiency.
Introduction to Apache Kylin
Apache Kylin is an open-source OLAP engine built to bring sub-second query
performance to massive datasets. Originally developed by eBay and later donated
to the Apache Software Foundation, Kylin has grown into a widely adopted tool
for big data analytics, particularly in environments dealing with trillions of
records across complex pipelines. ... Another strength is Kylin’s unified big
data warehouse architecture. It integrates natively with the Hadoop ecosystem
and data lake platforms, making it a solid fit for organizations already
invested in distributed storage. For visualization and business reporting, Kylin
integrates seamlessly with tools like Tableau, Superset, and Power BI. It
exposes query interfaces that allow us to explore data without needing to
understand the underlying complexity. ... At the heart of Kylin is its data
model, which is built using star or snowflake schemas to define the
relationships between the underlying data tables. In this structure, we define
dimensions, which are the perspectives or categories we want to analyze (like
region, product, or time). Alongside them are measures, and aggregated numerical
values such as total sales or average price. ... To achieve its speed, Kylin
heavily relies on pre-computation. It builds indexes (also known as CUBEs) that
aggregate data ahead of time based on the model dimensions and measures.
No comments:
Post a Comment