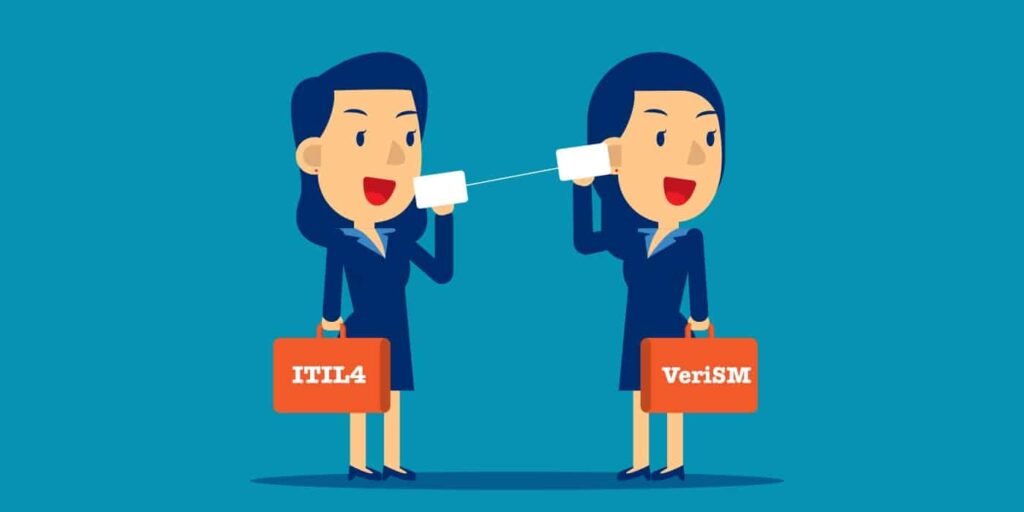
Even though ITIL has been around for many years and is considered the de facto
best practice framework for IT service management (ITSM), VeriSM emerged in 2018
to find its place in the market. And this came before the launch of ITIL 4 from
AXELOS in February 2019. VeriSM’s publication introduced some modern approaches
in service management such as Agile and shift-left among others. ITIL 4, once
released, also incorporated these modern concepts that have conquered the IT
world during the last few years. VeriSM claims not to be a body of service
management best practice but is instead an approach where the key facet of the
model (it’s not a process flow, nor a set of procedures) is the Management Mesh
where all the popular management practices (ITIL, COBIT, ISO/IEC 20000,
CMMI-SVC, DevOps, Agile, Lean, SIAM, etc.) and emerging technologies and trends
(artificial intelligence (AI), containerization, the Internet of Things (IoT),
big data, cloud, shift-left, continuous delivery, CX/UX, etc.) are included.
Maybe there’s some truth in this statement.

Introduced last year, Gloo Mesh Enterprise is an Istio-based Kubernetes-native
solution for multicluster and multimesh service mesh management. New features in
2.0 such as multitenant workspaces enable users to set fine-grained access
control and editing permissions based on roles for shared infrastructure,
enabling teams to collaborate in large environments. Users can manage traffic,
establish workspace dependencies, define cluster namespaces, and control
destinations directly in the UI. And the policies can be re-used and adapted
using labels. Gloo Mesh Enterprise 2.0 also features a new Gloo Mesh API for
Istio management enables developers to configure rules and policies for both
north-south traffic and east-west traffic from a single, unified API. The new
API also simplifies the process of expanding from a single cluster to dozens or
hundreds of clusters. And the new Gloo Mesh UI for observability provides
service topology graphs that highlight network traffic, latency, and speeds
while automatically saving the new state when you move clusters or
nodes.
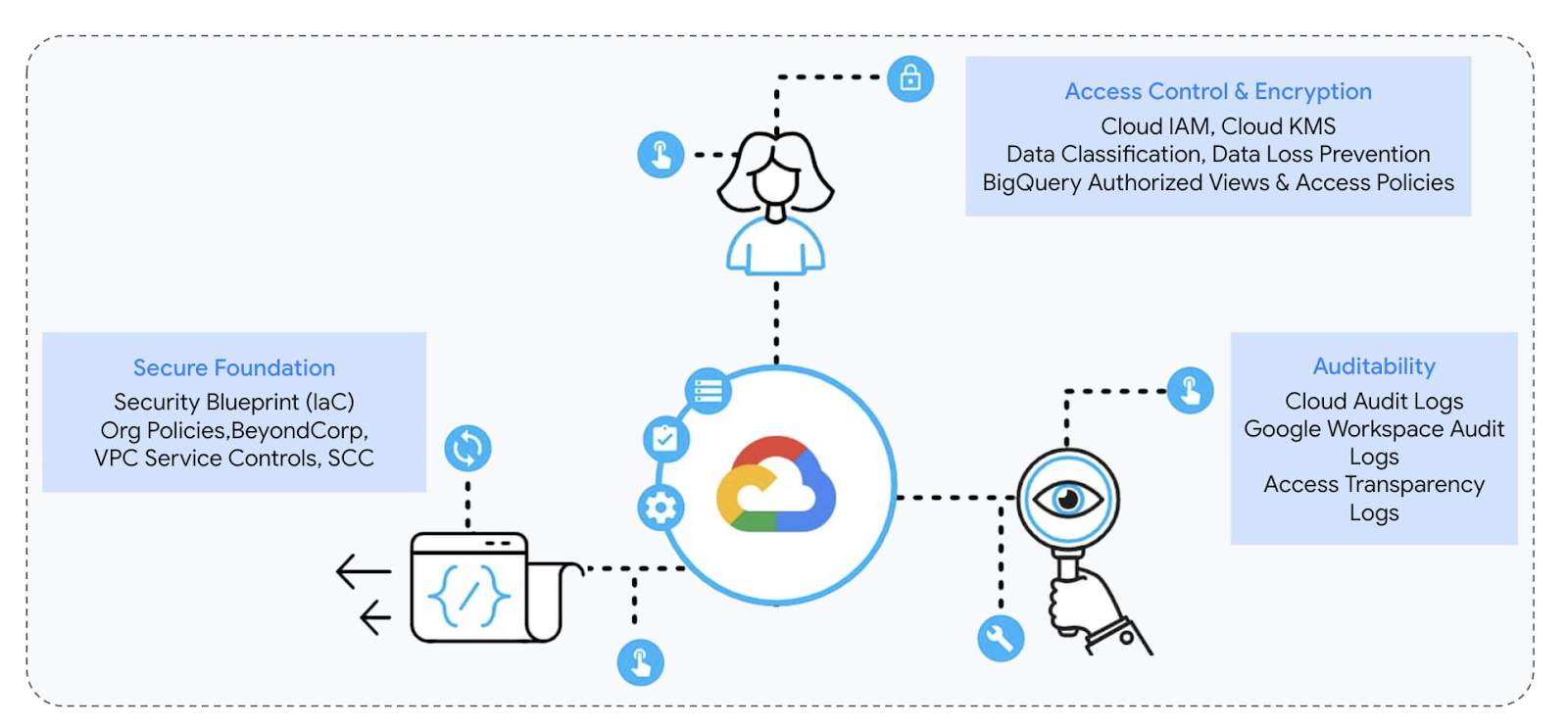
You can use CSA to further investigate high-fidelity security findings from
Security Command Center (SCC) and correlate them with logs for decision-making.
For example, you may use a CSA query to get the list of admin activity performed
by a newly created service account key flagged by Security Command Center in
order to validate any malicious activity. It’s important to note that the
detection queries provided by CSA will be self-managed and you may need to tune
to minimize alert noise. If you’re looking for managed and advanced detections,
take a look at SCC Premium’s growing threat detection suite which provides a
list of regularly-updated managed detectors designed to identify threats within
your systems in near real-time. CSA is not meant to be a comprehensive, managed
set of threat detections, but a collection of community-contributed sample
analytics to give examples of essential detective controls, based on cloud
techniques. Use CSA in conjunction with our threat detection and response
capabilities in conjunction with our threat prevention capabilities.
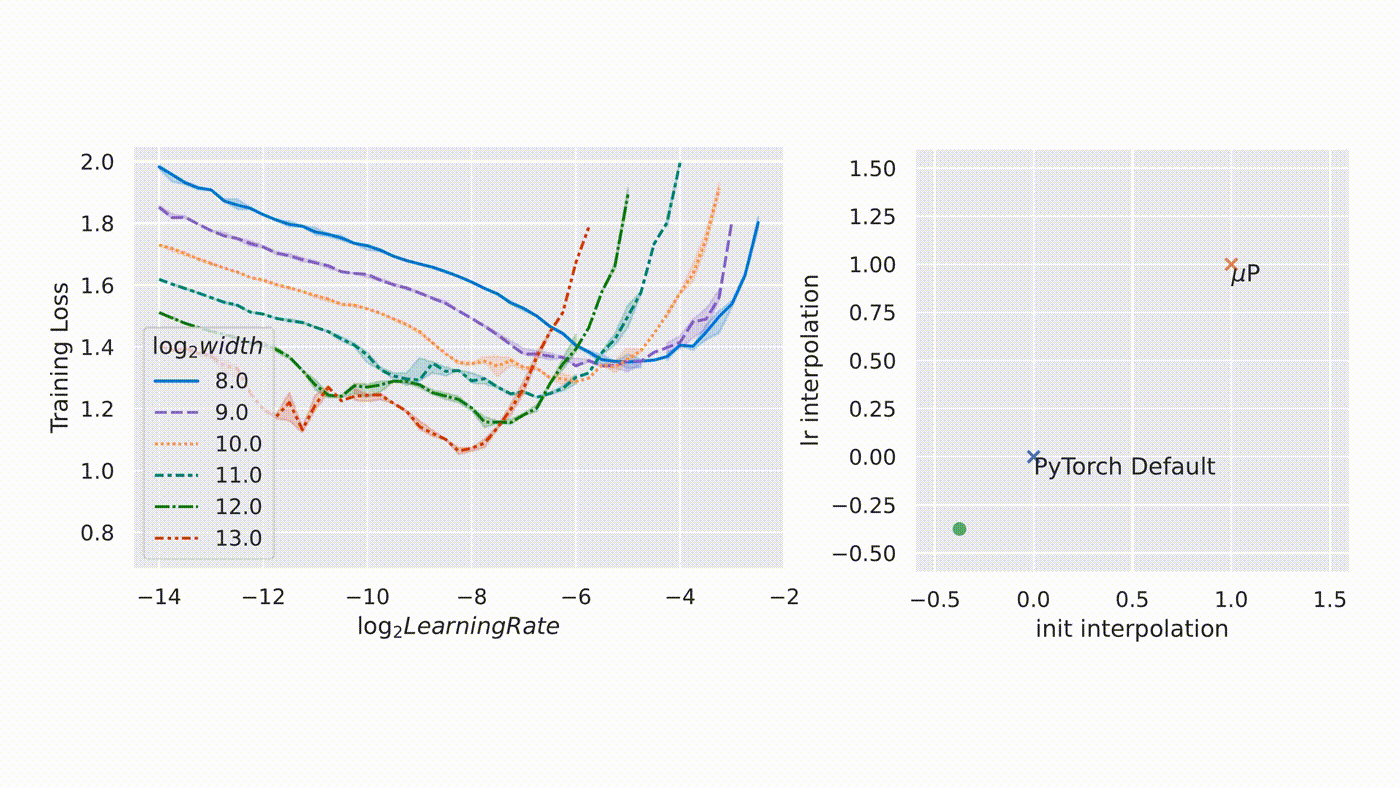
Our theory of scaling enables a procedure to transfer training hyperparameters
across model sizes. If, as discussed above, µP networks of different widths
share similar training dynamics, they likely also share similar optimal
hyperparameters. Consequently, we can simply apply the optimal hyperparameters
of a small model directly onto a scaled-up version. We call this practical
procedure µTransfer. If our hypothesis is correct, the training
loss-hyperparameter curves for µP models of different widths would share a
similar minimum. Conversely, our reasoning suggests that no scaling rule of
initialization and learning rate other than µP can achieve the same result. This
is supported by the animation below. Here, we vary the parameterization by
interpolating the initialization scaling and the learning rate scaling between
PyTorch default and µP. As shown, µP is the only parameterization that preserves
the optimal learning rate across width, achieves the best performance for the
model with width 213 = 8192, and where wider models always do better for a given
learning rate—that is, graphically, the curves don’t intersect.

Transformers quickly became the front-runner for applications like word
recognition that focus on analyzing and predicting text. It led to a wave of
tools, like OpenAI’s Generative Pre-trained Transformer 3 (GPT-3), which trains
on hundreds of billions of words and generates consistent new text to an
unsettling degree. The success of transformers prompted the AI crowd to ask what
else they could do. The answer is unfolding now, as researchers report that
transformers are proving surprisingly versatile. In some vision tasks, like
image classification, neural nets that use transformers have become faster and
more accurate than those that don’t. Emerging work in other AI areas — like
processing multiple kinds of input at once, or planning tasks — suggests
transformers can handle even more. “Transformers seem to really be quite
transformational across many problems in machine learning, including computer
vision,” said Vladimir Haltakov, who works on computer vision related to
self-driving cars at BMW in Munich. Just 10 years ago, disparate subfields of AI
had little to say to each other. But the arrival of transformers suggests the
possibility of a convergence.

In February 2022, an op-ed, titled “Revisiting Bitcoin’s Carbon Footprint,” was
published in the scientific journal “Joule,” authored by four researchers: Alex
de Vries, Ulrich Gallersdörfer, Lena Klaaßen and Christian Stoll. Their written
commentary, which admits limitations in their estimates, states that as bitcoin
miners migrated from China to Kazakhstan and the United States in 2021, the
network’s carbon footprint increased to 0.19% of global emissions. What went
unnoticed by the media was that the researchers have professional motives to
overstate Bitcoin’s relatively tiny environmental impact. The op-ed’s lead
author, Alex de Vries, failed to disclose that he is employed by De
Nederlandsche Bank (DNB), the Dutch central bank. Central banks are no fans of
open, global payment rails, which bypass monopolistic government settlement
layers. De Vries first released his “Bitcoin Energy Consumption Index” in
November 2016, which coincides with his first round of employment with DNB,
giving the appearance that DNB encouraged his critique of Bitcoin’s energy
consumption.

From a DBA perspective (and being a former DBA myself), I always enjoyed working
on more challenging issues. Mundane operations like launching servers and
setting up backups make for a less-than-exciting daily work experience. When
managing large fleets, these operations make up the majority of the work. As
applications grow more complex and data sets grow rapidly, it is much more
interesting to work with the application teams to design and optimize the data
tier. Query tuning, schema design, and workflow analysis are much more
interesting (and often beneficial) when compared to the basic setup. DBAs are
often skilled at quickly identifying issues and understanding design issues
before they become problems. When an enterprise adopts a DBaaS model, this can
free up the DBAs to work on more complex problems. They are also able to better
engage and understand the applications they are supporting. A common comment I
get when discussing complex tickets with clients is: “well, I have no idea what
the application is doing, but we have an issue with XYZ”.
The leadership gap that has been forecasted for the past several years is upon
us. And, it could not have come at a worse time with the Covid-19 pandemic still
underway, impacting each of the multiple generations in the workforce
differently. Many companies are unable to keep pace with their need to fill
leadership openings created by Baby Boomers taking retirement and by companies
expanding, in some cases at rapid rates. Their pipelines are not sufficient to
fill the increasing number of leadership openings promptly. Companies that lack
a focused strategy and drive to close this gap might very well find themselves
struggling to stay in business and maintain their market share. The significant
numbers of Baby Boomers taking retirement for the past ten years have only
exacerbated the leadership gap. Many of them are leaving their leadership roles
for their well-earned leisure lifestyle. In the third quarter of 2020, the
number of Boomers who retired increased by over three million from the same
quarter in 2019.

As construction companies continue to comply with pandemic restrictions,
technology has been essential to the implementation of health and safety
measures. For instance, firms can use wearables and AI sensors to detect when
workers are not maintaining proper physical distance. Some construction projects
are even using contact tracing devices that alert employees when there are too
many personnel at a worksite; these can identify potentially infected
individuals in the event of a confirmed COVID-19 case. These measures not only
prioritize employee safety, but also help companies avoid entire site shutdowns.
Even remotely, technology is a vital asset to construction firms. With fewer
personnel allowed on-site, companies can rely on new cloud-based video platforms
to assist with site monitoring. In the city of Miami, virtual inspections of
construction sites through either a Zoom or a Microsoft Teams video call are now
routine between engineers on site and building control officials. With usage
tripling in 2020 alone, drones are also being used more frequently to improve
mapping and surveying processes.

Leaders often regard purpose in a limited way as either a marketing or human
resources exercise. Companies that go deepest with purpose take a much more
comprehensive approach, treating purpose as an operating system and embedding it
in processes, organizational structures, and culture. Global professional
services firm EY adopted a system of metrics to spur behaviors associated with
its purpose. “Companies really have to be able to show what they’re doing,” EY’s
CEO Carmine Di Sibio told me. “They get into trouble when they talk a lot about
purpose and it’s just talk.” Imagine what it feels like when everything about
your work ties back in clear, even obvious ways to your purpose. That’s what
employees at deep-purpose companies experience on the job. It’s encouraging that
some CEOs—68% of those queried in one survey—are placing “more emphasis” on
purpose, but that’s not enough. For purpose to feel genuine and meaningful, they
must live it in their daily work, hold others accountable for acting in ways
congruent with that purpose, and bring it alive for their workforce.
Quote for the day:
"The essence of leadership is the
willingness to make the tough decisions. Prepared to be lonely." --
Colin Powell
No comments:
Post a Comment