Future in Fintech in 2020: a revolution in financial sectors

Experts foresight 2020 to bring a tremendous change in the future of the
Fintech industry. Goldman Sachs predicts that by the end of 2020, the
worldwide Fintech pie will reckon up $4,7 trillion. Parenthetically, the
interesting fact is that nearly one-third of Goldman Sachs’ employees are
engineers, which makes more than on Twitter or Facebook. We found out the five
main trends in Fintech banking that are going to disrupt the industry and
drive the immense growth. The age of totally digital banking is approaching.
The majority of existing banks already offer global payments and transfers
virtually, and those who don’t yet will join the trend. The ability to trade
currencies along with Bitcoin and Ethereum online will come to a daily basis,
and according to the forecasts, it will lead to a drop in physical bank visits
by 36% by 2020. Though in 2019 the blockchain technology became a widely
discussed topic, its embodiment into financial services was relatively slower,
compared to other spheres. The future of Fintech in 2020 is intimately tied to
the blockchain technology, and the main reasons are transparency and trust it
guarantees, significantly decreasing the time needed for transactions and
improving the cash flow. 77% of surveyed incumbents expect to embrace
blockchain by 2020.
New taskforce to push cyber security standards
It follows earlier reports on Monday that the federal government is crafting
minimum cyber security standards for businesses, including critical
infrastructure, as part of its next cyber security strategy. The
taskforce will focus its efforts on “harmonising baseline standards and
providing clarity for sector specific additional standards and guidance” and
improving interoperability. It also aims to enhance "competitiveness
standards by sector for both supplier and consumers” and support Australian
cyber security companies to seize opportunities globally. ... “We know that
the current plethora of different security standards make it difficult for
government and industry to know what they’re buying when it comes to cyber
security,” he said. “By bringing together industry to identify relevant
standards and provide other practical guidance, we aim to make government more
secure, whilst providing direction for industry to build their cyber
resilience. “This will realise our ambition for NSW to become the leading
cyber security hub in the Southern Hemisphere.”
4 edge computing use cases delivering value in the enterprise
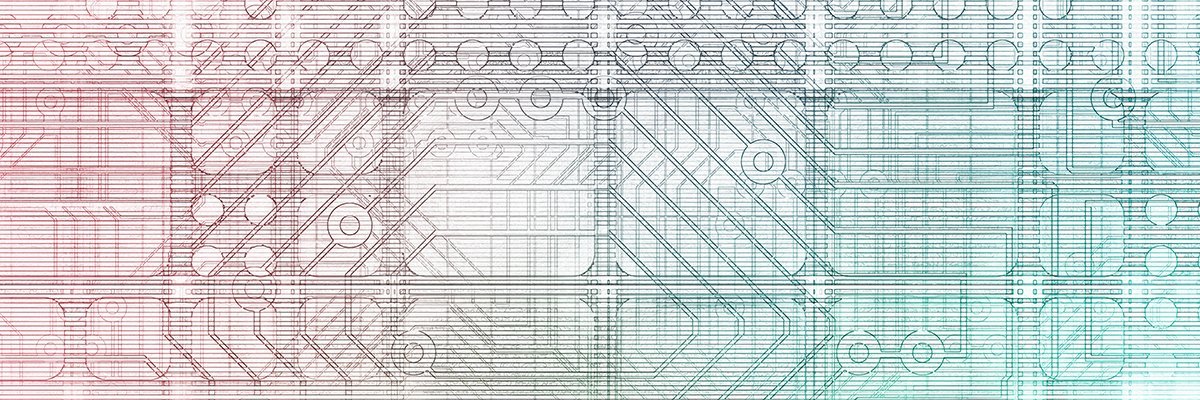
However, all that data doesn't need to be handled in centralized servers;
similar to the healthcare edge computing use cases, every temperature reading
from every connected thermometer, for example, isn't important. Rather, most
organizations only need to bring aggregate data or average readings back to
their central systems, or they only need to know when such readings indicate a
problem, such as a temperature on a unit that's out of normal range. Edge
computing enables organizations to take and understand the data near those
endpoint devices, thereby limiting the cost and complexity of sending reams of
often unneeded data points to central systems, while still gaining the
benefits of understanding the performance of its equipment. The ROI of this is
critical: The insights into the data generated by endpoint devices enable
remote monitoring so organizations can identify performance problems and
safety issues early, even when no one is on-site. Using edge computing with
predictive and prescriptive analytics can deliver even bigger ROI, as they
enable organizations to predict the optimal time to service their equipment.
Why There Are Silos And Gaps In SOCs… And What To Do About It

Detections are an event that looks anomalous or malicious. And the issue today
in a modern security operations center (SOC) is that detections can bubble up
from many siloed tools. For example, you have firewall and network detection
and response (NDR) for your network protection, Endpoint Detection and
Response (EDR) for your endpoints’ protection and Cloud Application Security
Broker (CASB) for your SaaS applications. Correlating those detections to
paint a bigger picture is the issue, since hackers are now using more complex
techniques to access your applications and data with increased attack
surfaces. Your team is either claiming false positives or an inability to see
through these detections and get a sense of what is critical vs. noise. The
main purpose of SIEMs is to collect and aggregate data such as logs from
different tools and applications for activity visibility and incident
investigation. That said there are still a lot of manual tasks needed, like
transforming the data including the data fusion to create context for the
data, i.e., enrichment with threat intelligence, location, asset and/or user
information.
Intel Tiger Lake processors to feature built-in malware protection
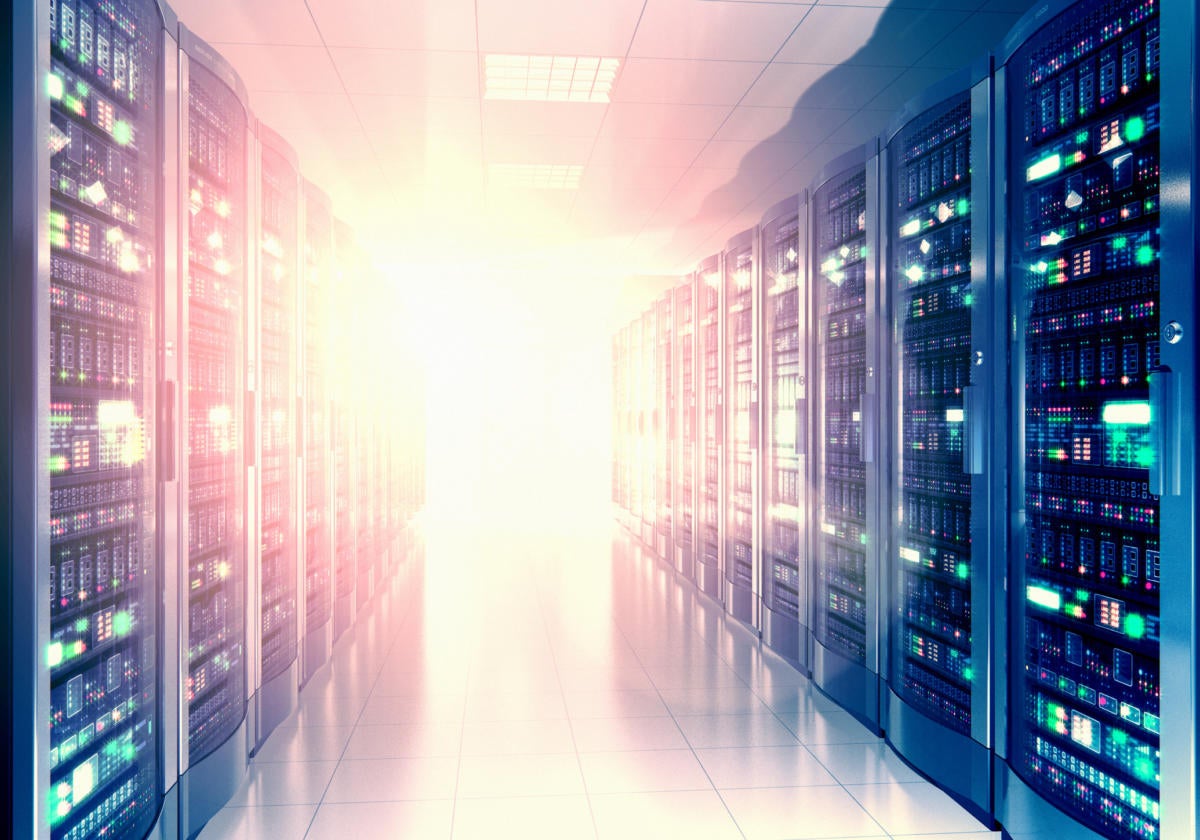
Intel CET deals with the order in which operations are executed inside the
CPU. Malware can use vulnerabilities in other apps to hijack their control
flow and insert malicious code into the app, making it so that the malware
runs as part of a valid application, which makes it very hard for
software-based anti-virus programs to detect. These are in-memory attacks,
rather than writing code to the disk or ransomware. Intel cited TrendMicro’s
Zero Day Initiative (ZDI), which said 63.2% of the 1,097 vulnerabilities
disclosed by ZDI from 2019 to today were related to memory safety. "It takes
deep hardware integration at the foundation to deliver effective security
features with minimal performance impact," wrote Tom Garrison, vice president
of the client computing group and general manager of security strategies and
initiatives at Intel in a blog post announcing the products. "As our work here
shows, hardware is the bedrock of any security solution. Security solutions
rooted in hardware provide the greatest opportunity to provide security
assurance against current and future threats. Intel hardware, and the added
assurance and security innovation it brings, help to harden the layers of the
stack that depend on it," Garrison wrote.
Why We Need DevOps for ML Data

We are now starting to see MLOps bring DevOps principles and tooling to ML
systems. MLOps platforms like Sagemaker and Kubeflow are heading in the right
direction of helping companies productionize ML. They require a fairly
significant upfront investment to set up, but once properly integrated, can
empower data scientists to train, manage, and deploy ML
models. Unfortunately, most tools under the MLOps banner tend to focus
only on workflows around the model itself (training, deployment, management) —
which represents a subset of the challenges for operational ML. ML
applications are defined by code, models, and data4. Their success depends on
the ability to generate high-quality ML data and serve it in production
quickly and reliably… otherwise, it’s just “garbage in, garbage out.” The
following diagram, adapted and borrowed from Google’s paper on technical debt
in ML, illustrates the “data-centric” and “model-centric” elements in ML
systems.
Data Lake vs Data Warehouse
Although data warehouses can handle unstructured data, they cannot do so
efficiently. When you have a large amount of data, storing all your data in a
database or data warehouse can be expensive. In addition, the data that comes
into the data warehouses must be processed before it can be stored in some
shape or structure. In other words, it should have a data model. In response,
businesses began to support Data Lakes, which stores all structured and
unstructured enterprise data on a large scale in the most cost-effective way.
Data Lakes stores raw data and can operate without having to determine the
structure and layout of the data beforehand. In the case of the Data Lake, the
information is structured at the output when you need to extract data and
analyze it. At the same time, the process of analysis does not affect the data
themselves in the lake — they remain unstructured so that they can be
conveniently stored and used for other purposes. This way we get the
flexibility that Data Warehouse hasn't. Thus, the Data Lake differs
significantly from the Data Warehouse. However, LSA's architectural approach
can also be used in the construction of Data Lake(my representation).
Lessons learned: Strategies to adjust IT operations in a crisis
Some IT organizations already had a robust VPN setup, as well as sufficient
laptops for staff to continue their work from home. Others, particularly those
without remote work policies already in place, had to rush to adjust. But even
after all the firefighting, some IT organizations have used the pandemic as an
opportunity to identify potential improvements within their environments --
whether through automation or AI, security updates or a streamlined help desk.
Use the below synopses of five recent SearchITOperations articles by
TechTarget senior news writer Beth Pariseau to explore the adjustments
organizations have made to maintain, manage and even optimize IT operations
during a crisis. With the overwhelming shift back to localized work
environments in the 2010s, many IT organizations in 2020 scrambled to
accommodate new restrictions and complications related to COVID-19. In-person,
impromptu discussions and weekly co-located meetings became impossible with
all staff offsite, which created bottlenecks and, in some cases, a slow-down
in productivity.
Millions of Connected Devices Have Exploitable TCP/IP Flaws
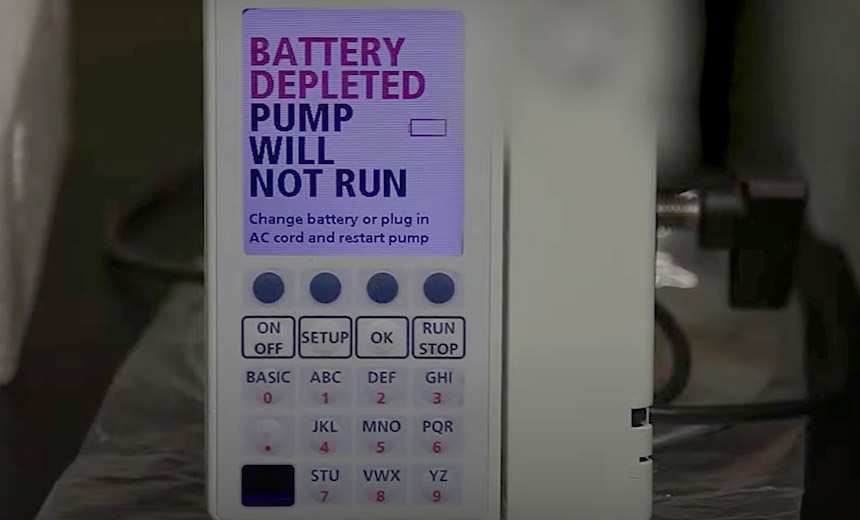
Treck says in a statement that it has updated its TCP/IPv4/v6 software to fix
the issues. JSOF notes that organizations should use Treck's stack version
6.0.1.67 or higher. JSOF dubbed the flaws Ripple20 to reflect how a single
vulnerable component can have a ripple effect on "a wide range of industries,
applications, companies, and people." The company is due to present its
findings at Black Hat 2020, which will be a virtual event. Four of the flaws
are rated critical, and two of them could be exploited to remotely take
control of a device. Others require an attacker to be on the same network as
the targeted device, which makes these flaws more difficult - but not
impossible - to exploit. "The risks inherent in this situation are high," JSOF
says. "Just a few examples: Data could be stolen off of a printer, an infusion
pump behavior changed, or industrial control devices could be made to
malfunction. An attacker could hide malicious code within embedded devices for
years." Simpson of Armis says that patching will be time consuming since
administrators may have to manually update every make and model of vulnerable
device.
Introducing The Fourth Generation of Core Banking System
The cracks began to show in the wake of the global financial crisis as the banks
were faced with a difficult challenge in that they had to both drive the costs
of their IT infrastructure down to allow them to maintain competitive banking
products in the market, as well as having to adapt to shifting consumer
expectations and increasingly stringent regulator demands. A notable example of
the latter being the introduction of the third installation of the Basel accord,
Basel III, which places increasing demands on banks to adapt their core systems
in ways that seem to directly clash with the traditional model of end of day
batch style processing, such as the requirement of intraday liquidity
management. All of a sudden the banks found themselves facing two key challenges
that seemed to conflict with each other. To drive the cost of their
infrastructure down they needed to get rid of their mainframes and run on leaner
infrastructure which would lower the glass ceiling on the amount of processing
power in the system. At the same time, to adapt to regulatory changes they had
to increase the frequency of their batch processing jobs, which would require
more processing power.
Quote for the day:
No comments:
Post a Comment