Data Architecture Trends in 2025
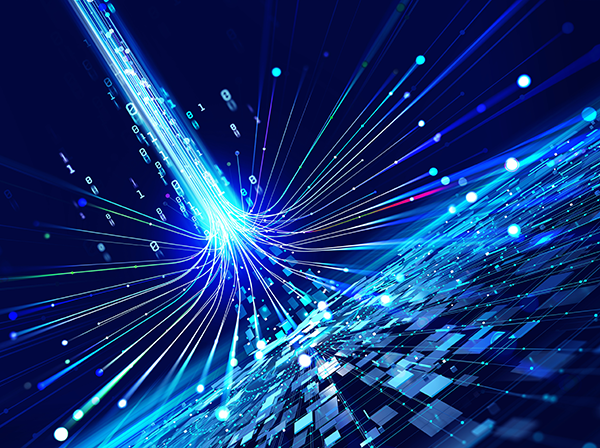
While unstructured data makes up the lion’s share of data in most companies
(typically about 80%), structured data does its part to bulk up business’
storage needs. Sixty-four percent of organizations manage at least one
petabyte of data, and 41% of organizations have at least 500 petabytes of
data, according to the AI & Information Management Report. By 2028, global
data creation is projected to grow to more than 394 zettabytes – and clearly
enterprises will have more than their fair share of that. Time to open the
door to the data lakehouse, which combines the capabilities of data lakes and
data warehouses, simplifying data architecture and analytics with unified
storage and processing of structured, unstructured, and semi-structured data.
“Businesses are increasingly investing in data lakehouses to stay
competitive,” according to MarketResearch, which sees the market growing at a
22.9% CAGR to more than $66 billion by 2033. ... “Through 2026, two-thirds of
enterprises will invest in initiatives to improve trust in data through
automated data observability tools addressing the detection, resolution, and
prevention of data reliability issues,” according to Matt Aslett.
How Does a vCISO Leverage AI?

CISOs design and inform policy that shapes security at a company. They inform
the priorities of their organizations’ cyberdefense deployment and design,
develop, or otherwise acquire the tools needed to achieve the goals they set
up. They implement tools and protections, monitor effectiveness, make
adjustments, and generally ensure that security functions as desired. However,
all that responsibility comes at immense costs, and CISOs are in high demand.
It can be challenging to recruit and retain top-level talent for the role, and
many smaller or growing organizations—and even some larger older ones—do not
employ a traditional, full-time CISO. Instead, they often turn to vCISOs. This
is far from a compromise, as vCISOs offer all of the same functionality as
their traditional counterparts through an entire team of dedicated service
providers rather than a single employee. Since vCISOs are available on a
fractional basis, organizations only pay for specific services they need. ...
As with all technological breakthroughs, AI is not without its risks and
drawbacks. Thankfully, working with a vCISO allows organizations to take
advantage of all the benefits of AI while also minimizing its potential
downsides. A capable vCISO team doesn’t use AI or any other tool just for the
sake of novelty or appearances; their choices are always strategic and
risk-informed.
The Transformative Benefits of Enterprise Architecture

Enterprise Architecture review or development is essential for managing
complexity, particularly when changes involve multiple systems with intricate
interdependencies. ... Enterprise Architecture provides a structured approach
to handle these complexities effectively. Often, key stakeholders, such as
department heads, project managers, or IT leaders, identify areas of change
required to meet new business goals. For example, an IT leader may highlight
the need for system upgrades to support a new product launch or a department
head might identify process inefficiencies impacting customer satisfaction.
These stakeholders are integral to the change process, and the role of the
architect is to: Identify and refine the requirements of the
stakeholders; Develop architectural views that address concerns and
requirements; Highlight trade-offs needed to reconcile conflicting
concerns among stakeholders. Without Enterprise Architecture, it is highly
unlikely that all stakeholder concerns and requirements will be
comprehensively addressed. This can lead to missed opportunities,
unanticipated risks, and inefficiencies, such as misaligned systems, redundant
processes, or overlooked security vulnerabilities, all of which can undermine
business goals and stakeholder trust.
Listen to your technology users — they have led to the most disruptive innovations in history

First, create a culture of open innovation that values insights from outside
the organization. While the technical geniuses in your R&D department are
experts in how to build something new, they aren’t the only authorities on
what it is you should build. Our research suggests that it’s especially
important to seek out user-generated disruption at times when customer needs
are changing rapidly. Talk to your customers and create channels for dialogue
and engagement. Most companies regularly survey users and conduct focus
groups. But to identify truly disruptive ideas, you need to go beyond
reactions to existing products and plumb unmet needs and pain points. Customer
complaints also offer insight into how existing solutions fall short. AI tools
make it easier to monitor user communities online and analyze customer
feedback, reviews, and complaints. Keep your pulse on social media and online
user communities where people share innovative ways to adapt existing products
and wish lists for new functionalities. ... Lastly, explore co-creation
initiatives that foster direct collaboration with user innovators. For
instance, run a contest where customers submit ideas for new products or
features, some of which could turn out to be truly disruptive. Or sponsor
hackathons that bring together users with needs and technical experts to
design solutions.
Guide to Data Observability
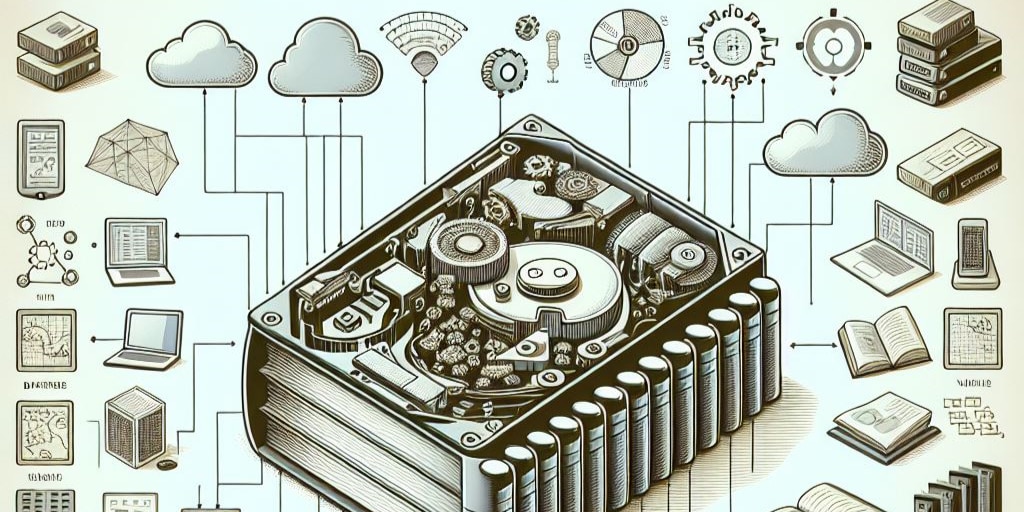
Data observability is critical for modern data operations because it ensures
systems are running efficiently, detecting anomalies, finding root causes, and
actively addressing data issues before they can impact business outcomes.
Unlike traditional monitoring, which focuses only on system health or
performance metrics, observability provides insights into why something is
wrong and allows teams to understand their systems in a more efficient way. In
the digital age, where companies rely heavily on data-driven decisions, data
observability isn’t only an operational concern but a critical business
function. ... When we talk about data observability, we’re focusing on
monitoring the data that flows through systems. This includes ensuring data
integrity, reliability, and freshness across the lifecycle of the data. It’s
distinct from database observability, which focuses more on the health and
performance of the databases themselves. ... On the other hand, database observability is specifically concerned with monitoring the
performance, health, and operations of a database system—for example, an SQL
or MongoDB server. This includes monitoring query performance, connection
pools, memory usage, disk I/O, and other technical aspects, ensuring the
database is running optimally and serving requests efficiently.
Data maturity and the squeezed middle – the challenge of going from good to great
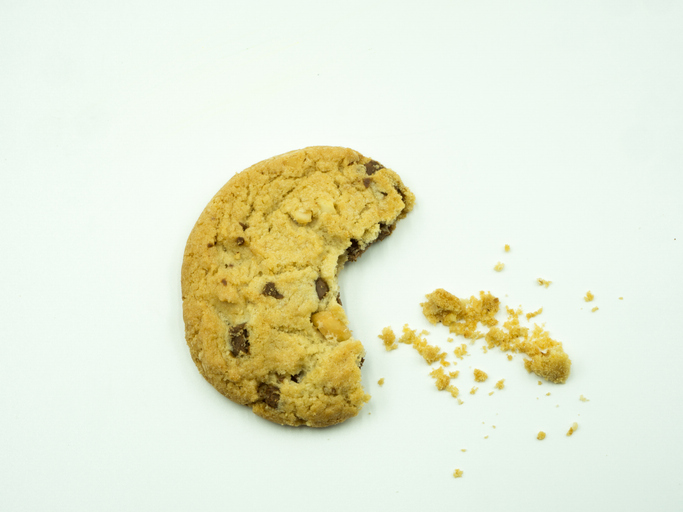
Breaking through this stagnation does not require a complete overhaul.
Instead, businesses can take small but decisive steps. First, they must shift
their mindset from seeing data collection as an end in itself, to viewing it
as a tool for creating meaningful customer interactions. This means moving
beyond static metrics and broad segmentations to dynamic, real-time
personalisation. The use of artificial intelligence (AI) can be transformative
in this regard. Modern AI tools can analyse customer behaviour in real time,
enabling businesses to respond with tailored content, promotions, and
experiences. For instance, rather than relying on broad-brush email campaigns,
companies can use AI-driven insights to craft (truly) hyper-personalised
messages based on individual customer journeys. Such efforts not only improve
conversion rates, but also build deeper customer loyalty. ... It’s
important to never lose sight of the fact that data maturity is about people
and culture as much as tech. Organisations need to foster a culture that
values experimentation, learning, and continuous improvement. Behaviourally,
this can be uncomfortable for slow-moving or cautious businesses and requires
breaking down silos and encouraging cross-functional collaboration.
Finding a Delicate Balance with AI Regulation and Innovation
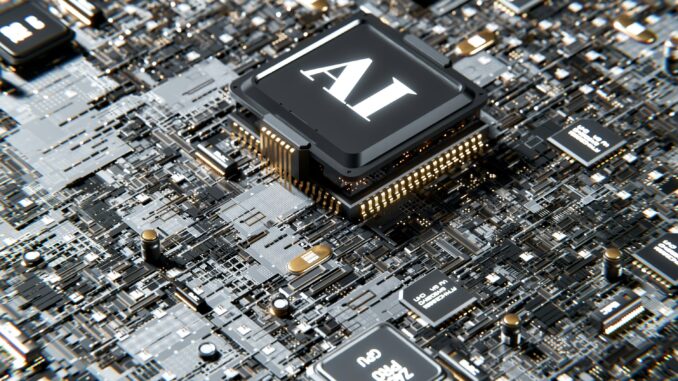
The first focus needs to be on protecting individuals and diverse groups from
the misuse of AI. We need to ensure transparency when AI is used, which in
turn will limit the amount of mistakes and biased outcomes, and when errors
are still made, transparency will help rectify the situation. It is also
essential that regulation tries to prevent AI from being used for illegal
activity, including fraud, discrimination and faking documents and creating
deepfake images and videos. It should be a requirement for companies of a
certain size to have an AI policy in place that is publicly available for
anyone to consult. The second focus should be protecting the environment. Due
to the amount of energy needed to train the AI, store the data and deploy the
technology ones it’s ready for market, AI innovation comes at a great cost for
the environment. It shouldn’t be a zero-sum game and legislation should nudge
companies to create AI that is respectful to the our planet. The third and
final key focus is data protection. Thankfully there is strong regulation
around data privacy and management: the Data Protection Act in the UK and GDPR
in the EU are good examples. AI regulation should work alongside existing data
regulation and protect the huge steps that have already been taken.
Quantum Machine Learning for Large-Scale Data-Intensive Applications
Quantum machine learning (QML) represents a novel interdisciplinary field
that merges principles of quantum computing with machine learning
techniques. The foundation of quantum computing lies in the principles of
quantum mechanics, which govern the behavior of subatomic particles and
introduce phenomena such as superposition and entanglement. These quantum
properties enable quantum computers to perform computations
probabilistically, offering potential advantages over classical systems in
specific computational tasks ... Integrating quantum machine learning (QML)
with traditional machine learning (ML) models is an area of active research,
aiming to leverage the advantages of both quantum and classical systems. One
of the primary challenges in this integration is the necessity for seamless
interaction between quantum algorithms and existing classical
infrastructure, which currently dominates the ML landscape. Despite the
resource-intensive nature of classical machine learning, which necessitates
high-speed computer hardware to train state-of-the-art models, researchers
are increasingly exploring the potential benefits of quantum computing to
optimize and expedite these processes.
Generative Architecture Twins (GAT): The Next Frontier of LLM-Driven Enterprise Architecture

A Generative Architecture Twin (GAT) is a virtual, LLM-coordinated
environment that mirrors — and continuously evolves with — your actual
production architecture. ... Despite the challenges, Generative Architecture
Twins represent an ambitious leap forward. They propose a world
where:Architectural decisions are no longer static but evolve with real-time
feedback loops. Compliance, security, and performance are integrated from
day one rather than tacked on later. EA documentation isn’t a dusty PDF but
a living blueprint that changes as the system scales. Enterprises can
experiment with high-risk changes in a safe, cost-controlled manner, guided
by autonomous AI that learns from every iteration. As we refine these
concepts, expect to see the first prototypes of GAT in innovative startups
or advanced R&D divisions of large tech enterprises. A decade from now,
GAT may well be as ubiquitous as DevOps pipelines are today. Generative
Architecture Twins (GAT) go beyond today’s piecemeal LLM usage and envision
a closed-loop, AI-driven approach to continuous architectural design and
validation. By combining digital twins, neuro-symbolic reasoning, and
ephemeral simulation environments, GAT addresses long-standing EA challenges
like stale documentation, repetitive compliance overhead, and costly rework.
Is 2025 the year of (less cloud) on-premises IT?
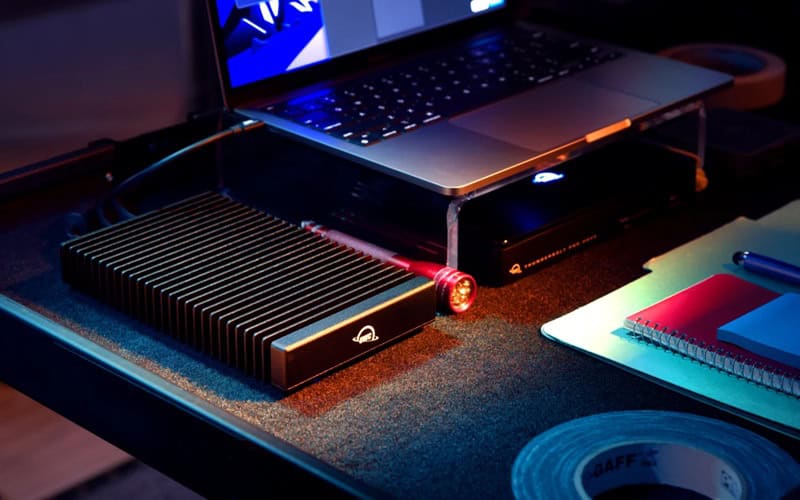
For an external view here outside of OWC, Vadim Tkachenko, technology fellow
and co-founder at Percona thinks that whether or not we’ll see a massive
wave of data repatriation take place in 2025 is still hard to say. “However,
I am confident that it will almost certainly mark a turning point for the
trend. Yes, people have been talking about repatriation off and on and in
various contexts for quite some time. I firmly believe that we are facing a
real inflection point for repatriation where the right combination of
factors will come together to nudge organisations towards bringing their
data back in-house to either on-premises or private cloud environments which
they control, rather than public cloud or as-a-Service options,” he said.
Tkachenko further states that companies across the private sector (and tech
in particular) are tightening their purse strings considerably. “We’re also
seeing more work on enhanced usability, ease of deployment, and of course,
automation. The easier it becomes to deploy and manage databases on your
own, the more organizations will have the confidence and capabilities needed
to reclaim their data and a sizeable chunk of their budgets,” said the
Percona man. It turns out then, cloud is still here and on-premises is still
here and… actually, a hybrid world is typically the most prudent route to go
down.
Quote for the day:
"The greatest leaders mobilize others by coalescing people around a shared
vision." -- Ken Blanchard
No comments:
Post a Comment