GenAI Won’t Work Until You Nail These 4 Fundamentals

Too often, organizations leap into GenAI fueled by excitement rather than
strategic intent. The urgency to appear innovative or keep up with competitors
drives rushed implementations without distinct goals. They see GenAI as the
“shiny new [toy],” as Kevin Collins, CEO of Charli AI, aptly puts it, but the
reality check comes hard and fast: “Getting to that shiny new toy is expensive
and complicated.” This rush is reflected in over 30,000 mentions of AI on
earnings calls in 2023 alone, signaling widespread enthusiasm but often without
the necessary clarity of purpose. ... The shortage of strategic clarity isn’t
the only roadblock. Even when organizations manage to identify a business case,
they often find themselves hamstrung by another pervasive issue: their
data. Messy data hampers organizations’ ability to mature beyond
entry-level use cases. Data silos, inconsistent formats and incomplete records
create bottlenecks that prevent GenAI from delivering its promised value. ...
Weak or nonexistent governance structures expose companies to various ethical,
legal and operational risks that can derail their GenAI ambitions. According to
data from an Info-Tech Research Group survey, only 33% of GenAI adopters have
implemented clear usage policies.
Inside the AI Data Cycle: Understanding Storage Strategies for Optimised Performance
The AI Data Cycle is a six-stage framework, beginning with the gathering and
storing of raw data. In this initial phase, data is collected from multiple
sources, with a focus on assessing its quality and diversity, which establishes
a strong foundation for the stages that follow. For this phase, high-capacity
enterprise hard disk drives (eHDDs) are recommended, as they provide high
storage capacity and cost-effectiveness per drive. In the next stage, data is
prepared for ingestion, and this is where insight from the initial data
collection phase is processed, cleaned and transformed for model training. To
support this phase, data centers are upgrading their storage infrastructure –
such as implementing fast data lakes – to streamline data preparation and
intake. At this point, high-capacity SSDs play a critical role, either
augmenting existing HDD storage or enabling the creation of all-flash storage
systems for faster, more efficient data handling. Next is the model training
phase, where AI algorithms learn to make accurate predictions using the prepared
training data. This stage is executed on high-performance supercomputers, which
require specialised, high-performing storage to function optimally.
Buy or Build: Commercial Versus DIY Network Automation
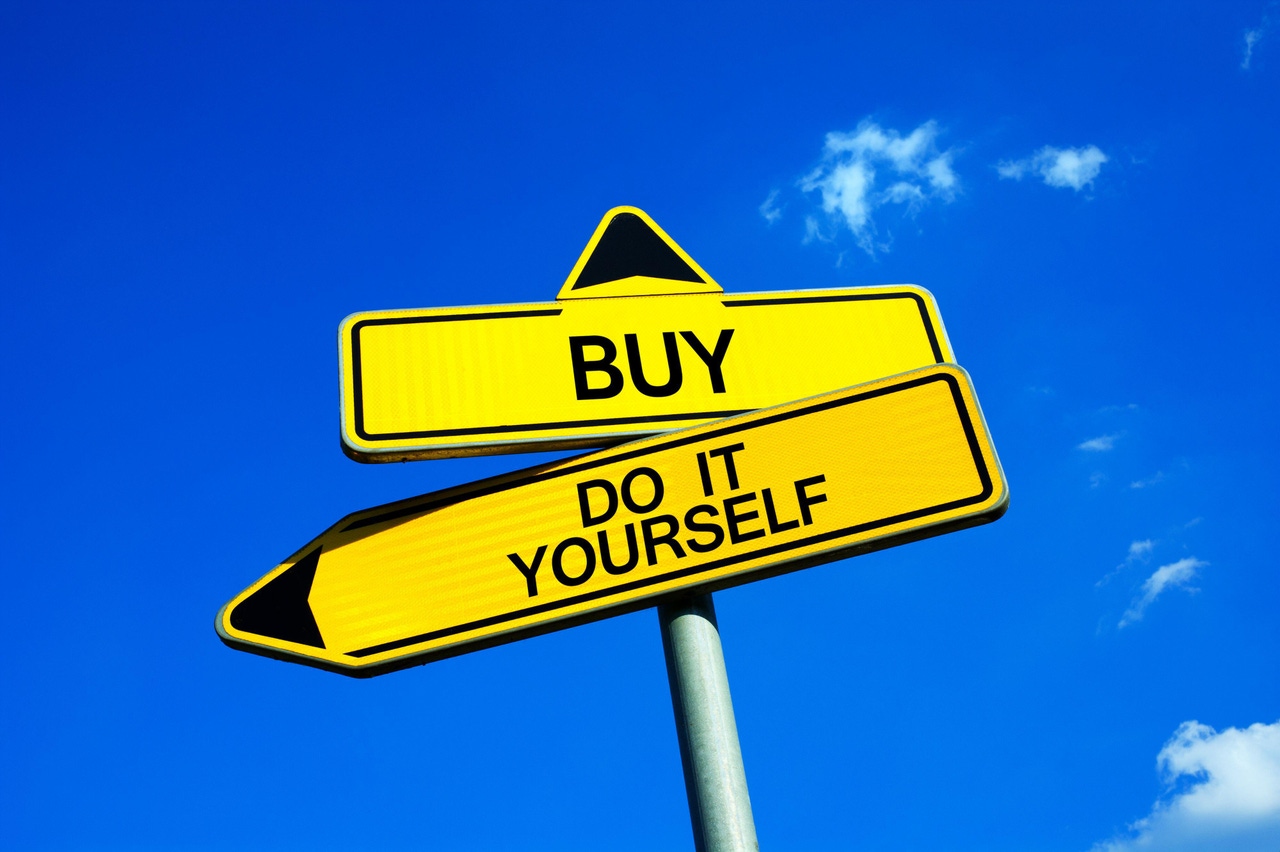
DIY automation can be tailored to your specific network and, in some cases, to meet security or compliance requirements more easily than vendor products. And they come at a great price: free! The cost of a commercial tool is sometimes higher than the value it creates, especially if you have unusual use cases. But DIY tools take time to build and support. Over 50% of organizations in EMA’s survey spend 6-20 hours per week debugging and supporting homegrown tools. Cultural preferences also come into play. While engineers love to grumble about vendors and their products, that doesn’t mean they prefer DIY. In my experience, NetOps teams are often set in their ways, preferring manual processes that do not scale up to match the complexity of modern networks. Many network engineers do not have the coding skills to build good automation, and most don't think about how to tackle problems with automation broadly. The first and most obvious fix for the issues holding back automation is simply for automation tools to get better. They must have broad integrations and be vendor neutral. Deep network mapping capabilities help resolve the issue of legacy networks and reduce the use cases that require DIY. Low or no-code tools help ease budget, staffing, and skills issues.
How HR can lead the way in embracing AI as a catalyst for growth
Common workplace concerns include job displacement, redundancy, bias in AI
decision-making, output accuracy, and the handling of sensitive data. Tracy
notes that these are legitimate worries that HR must address proactively. “Clear
policies are essential. These should outline how AI tools can be used,
especially with sensitive data, and safeguards must be in place to protect
proprietary information,” she explains. At New Relic, open communication about
AI integration has built trust. AI is viewed as a tool to eliminate repetitive
tasks, freeing time for employees to focus on strategic initiatives. For
instance, their internally developed AI tools support content drafting and
research, enabling leaders like Tracy to prioritize high-value activities, such
as driving organizational strategy. “By integrating AI thoughtfully and
transparently, we’ve created an environment where it’s seen as a partner, not a
threat,” Tracy says. This approach fosters trust and positions AI as an ally in
smarter, more secure work practices. The key is to highlight how AI can help
everyone excel in their roles and elevate the work they do every day. While it’s
realistic to acknowledge that some aspects of our jobs—or even certain roles—may
evolve with AI, the focus should be on how we integrate it into our workflow and
use it to amplify our impact and efficiency,” notes Tracy.
Cloud providers are running out of ‘next big things’

Yes, every cloud provider is now “an AI company,” but let’s be honest —
they’re primarily engineering someone else’s innovations into cloud-consumable
services. GPT-4 through Microsoft Azure? That’s OpenAI’s innovation. Vector
databases? They came from the open source community. Cloud providers are
becoming AI implementation platforms rather than AI innovators. ... The root
causes of the slowdown in innovation are clear. Market maturity indicates that
the foundational issues in cloud computing have mostly been resolved. What’s
left are increasingly specialized niche cases. Second, AWS, Azure, and Google
Cloud are no longer the disruptors — they’re the defenders of market share.
Their focus has shifted from innovation to optimization and retention. A
defender’s mindset manifests itself in product strategies. Rather than
introducing revolutionary new services, cloud providers are fine-tuning
existing offerings. They’re also expanding geographically, with the
hyperscalers expected to announce 30 new regions in 2025. However, these
expansions are driven more by data sovereignty requirements than innovative
new capabilities. This innovation slowdown has profound implications for
enterprises. Many organizations bet their digital transformation on
cloud-native architectures with continuous innovation.
Historical Warfare’s Parallels with Cyber Warfare
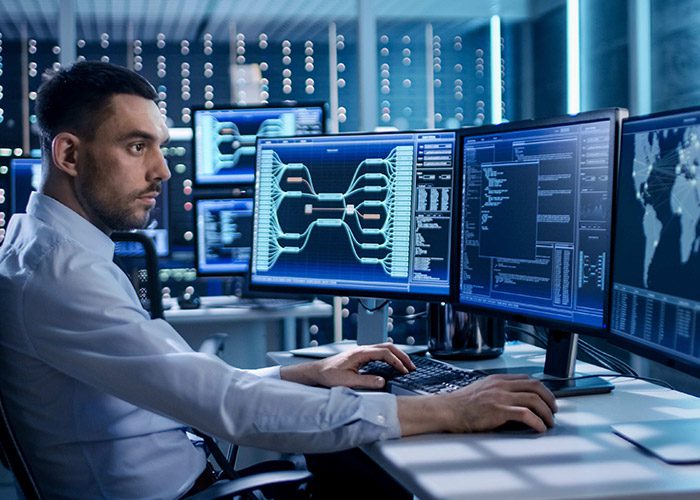
In 1942, the British considered Singapore nearly impregnable. They fortified
its coast heavily, believing any attack would come from the sea. Instead, the
Japanese stunned the defenders by advancing overland through dense jungle
terrain the British deemed impassable. This unorthodox approach using bicycles
in great numbers and small tracks through the jungle enabled the Japanese
forces to hit the defences at the weakest point and well ahead of the
projected time catching the British defences off guard. In cybersecurity, this
corresponds to zero-day vulnerabilities and unconventional attack vectors.
Hackers exploit flaws that defenders never saw coming, turning supposedly
secure systems into easy marks. The key lesson is to never to grow complacent
because you never know what you can be hit with and when. ... Cyber
attackers also use psychology against their targets. Phishing emails appeal to
curiosity, trust, greed, or fear thus luring victims into clicking malicious
links or revealing passwords. Social engineering exploits human nature rather
than code and defenders must recognise that people, not just machines, are the
frontline. Regular training, clear policies, and an ingrained culture of
healthy scepticism which is present in most IT staff can thwart even the most
artful psychological ploys.
Insider Threat: Tackling the Complex Challenges of the Enemy Within
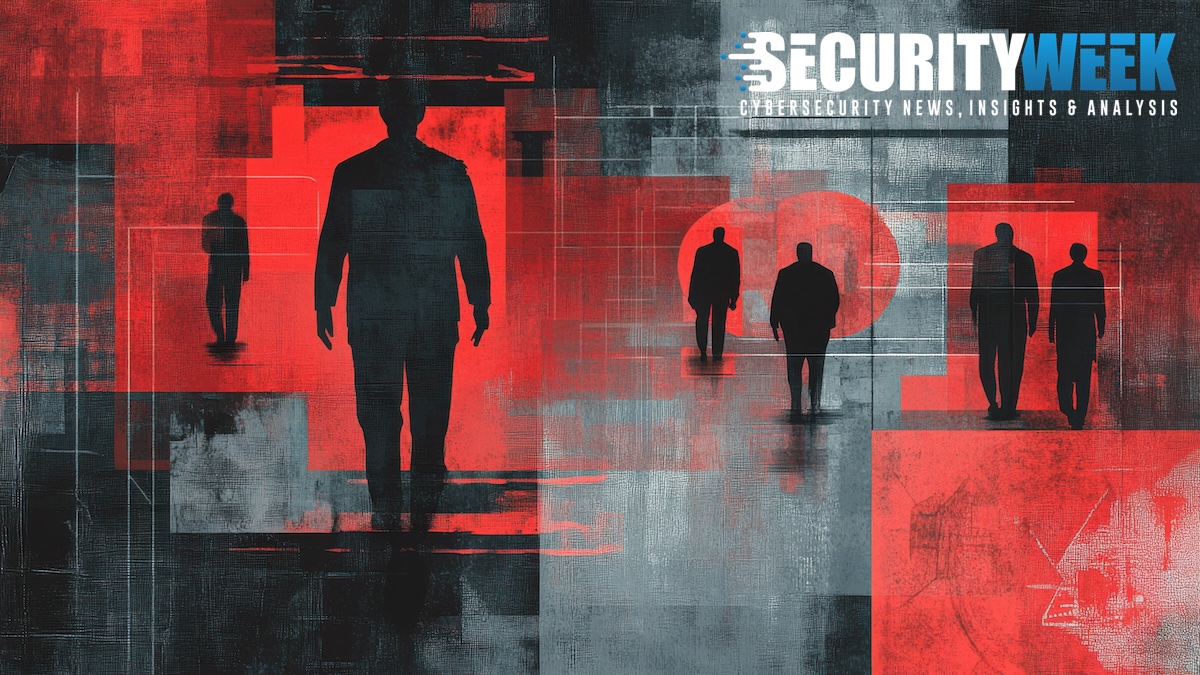
Third-party background checking can only go so far. It must be supported by
old fashioned and experienced interview techniques. Omri Weinberg,
co-founder and CRO at DoControl, explains his methodology “We’re primarily
concerned with two types of bad actors. First, there are those looking to
use the company’s data for nefarious purposes. These individuals typically
have the skills to do the job and then some – they’re often overqualified.
They pose a severe threat because they can potentially access and exploit
sensitive data or systems.” The second type includes those who oversell
their skills and are actually under or way underqualified. “While they might
not have malicious intent, they can still cause significant damage through
incompetence or by introducing vulnerabilities due to their lack of
expertise. For the overqualified potential bad actors, we’re wary of
candidates whose skills far exceed the role’s requirements without a clear
explanation. For the underqualified group, we look for discrepancies between
claimed skills and actual experience or knowledge during interviews.” This
means it is important to probe the candidate during the interview to gauge
the true skill level of the candidate. “it’s essential that the person
evaluating the hire has the technical expertise to make these
determinations,” he added.
Raise your data center automation game with easy ecosystem integration
If integrations are the key, then the things you look for to understand
whether a product is flashy or meaningful should change. The UI matters, but
the way tools are integrated is the truly telling characteristic. What APIs
exist? How is data normalized? Are interfaces versioned and maintained
across different releases? Can you create complex dashboards that pull
things together from different sources using no-code models that don't
require source access to contextualize your environment? How are workflows
strung together into more complex operations? By changing your focus, you
can start to evaluate these platforms based on how well they integrate
rather than on how snazzy the time series database interface is. Of course,
things like look and feel matter, but anyone who wants to scale their
operations will realize that the UI might not even be the dominant
consumption model over time. Is your team looking to click their way through
to completion? ... Wherever you are in this discovery process, let me offer
some simple advice: Expand your purview from the network to the ecosystem
and evaluate your options in the context of that ecosystem. When you do that
effectively, you should know which solutions are attractive but incremental
and which are likely to create more durable value for you and your
organization.
Why Scrum Masters Should Grow Their Agile Coaching Skills
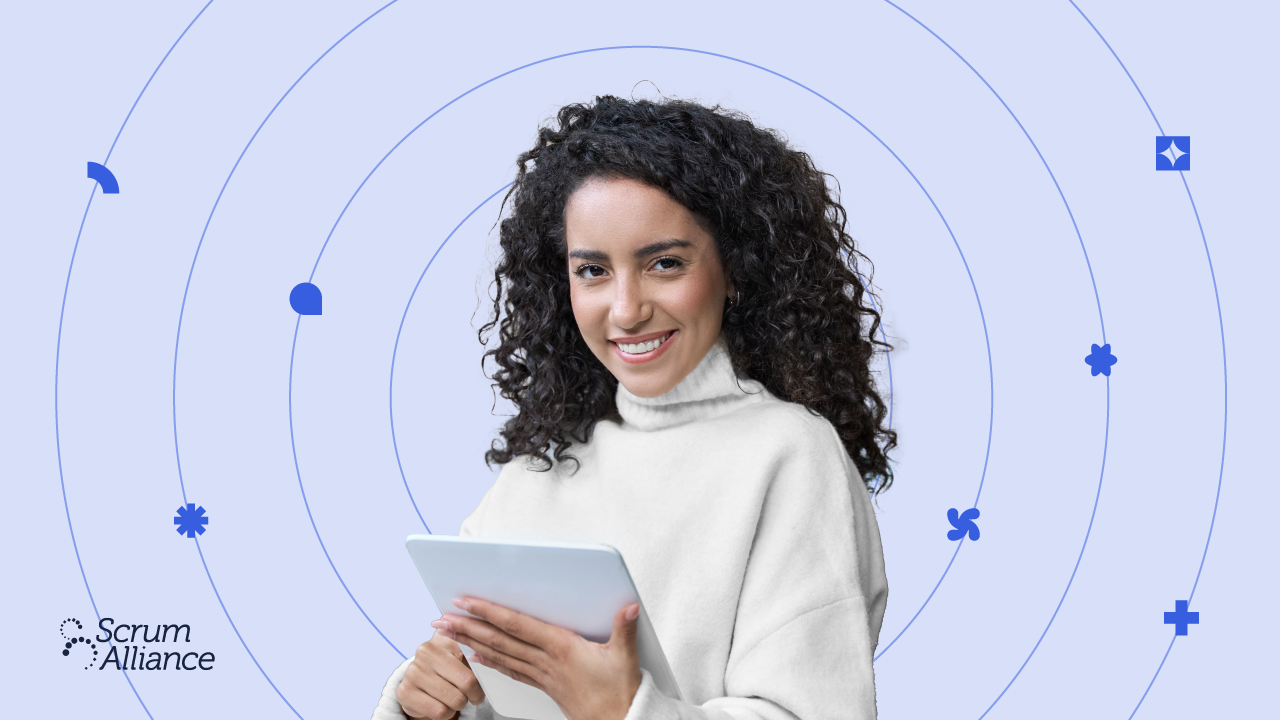
More than half of the organizations surveyed report that finding scrum
masters with the right combination of skills to meet their evolving demands
is very challenging. Notably, 93% of companies seek candidates with strong
coaching skills but state that it’s one of the skills hardest to find.
Building strong coaching and facilitation skills can help you stand out in
the job market and open doors to new career opportunities. As scrum masters
are expected to take on increasingly strategic roles, your skills become
even more valuable. Senior scrum masters, in particular, are called upon to
handle politically sensitive and technically complex situations, bridging
gaps between development teams and upper management. Coaching and
facilitation skills are requested nearly three times more often for senior
scrum master roles than for other positions. Growing these coaching
competencies can give you an edge and help you make a bigger impact in your
career. ... Who wouldn’t want to move up in their career into roles with
greater responsibilities and bigger impact? Regardless of the area of the
company you’re in—product, sales, marketing, IT, operations—you’ll need
leadership skills to guide people and enable change within the
organization.
Scaling penetration testing through smart automation
Automation undoubtedly has tremendous potential to streamline the
penetration testing lifecycle for MSSPs. The most promising areas are the
repetitive, data-intensive, and time-consuming aspects of the process. For
instance, automated tools can cross-reference vulnerabilities against known
exploit databases like CVE, significantly reducing manual research time.
They can enhance accuracy by minimizing human error in tasks like
calculating CVSS scores. Automation can also drastically reduce the time
required to compile, format, and standardize pen-testing reports, which can
otherwise take hours or even days depending on the scope of the project. For
MSSPs handling multiple client engagements, this could translate into faster
project delivery cycles and improved operational efficiency. For their
clients – it enables near real-time responses to vulnerabilities, reducing
the window of exposure and bolstering their overall security posture.
However – and this is crucial – automation should not be treated as a silver
bullet. Human expertise remains absolutely indispensable in the testing
itself. The human ability to think creatively, to understand complex system
interactions, to develop unique attack scenarios that an algorithm might
miss—these are irreplaceable.
Quote for the day:
"Don't judge each day by the harvest
you reap but by the seeds that you plant." --
Robert Louis Stevenson
No comments:
Post a Comment