Are we prepared for ‘Act 2’ of gen AI?

It’s both logical and tempting to design your AI usage around one large model.
You might think you can simply take a giant large language model (LLM) from your
Act 1 initiatives and just get moving. However, the better approach is to
assemble and integrate a mixture of several models. Just as a human’s frontal
cortex handles logic and reasoning while the limbic system deals with fast,
spontaneous responses, a good AI system brings together multiple models in a
heterogeneous architecture. No two LLMs are alike — and no single model can “do
it all.” What’s more, there are cost considerations. The most accurate model
might be more expensive and slower. For instance, a faster model might produce a
concise answer in one second — something ideal for a chatbot. ... Even in
its early days, gen AI quickly presented scenarios and demonstrations that
underscore the critical importance of standards and practices that emphasize
ethics and responsible use. Gen AI should take a people-centric approach that
prioritizes education and integrity by detecting and preventing harmful or
inappropriate content — in both user input and model output. For example,
invisible watermarks can help reduce the spread of disinformation.
Supercharge AIOps Efficiency With LLMs

One of the superpowers LLMs bring to the table is ultra-efficient summarization.
Given a dense information block, generative AI models can extract the main
points and actionable insights. Like with our earlier trials in algorithmic root
cause analysis, we gathered all the data we could surrounding an observed issue,
converted it into text-based prompts, and fed it to an LLM along with guidance
on how it should summarize and prioritize the data. Then, the LLM was able to
leverage its broad training and newfound context to summarize the issues and
hypothesize about root causes. Constricting the scope of the prompt by providing
the LLM the information and context it needs — and nothing more — we were able
to prevent hallucinations and extract valuable insights from the model. ...
Another potential application of LLMs is automatically generating post-mortem
reports after incidents. Documenting issues and resolutions is not only a best
practice but also sometimes a compliance requirement. Rather than scheduling
multiple meetings with different SREs, Developers, and DevOps to collect
information, could LLMs extract the necessary information from the Senser
platform and generate reports automatically?
“AI toothbrushes” are coming for your teeth—and your data
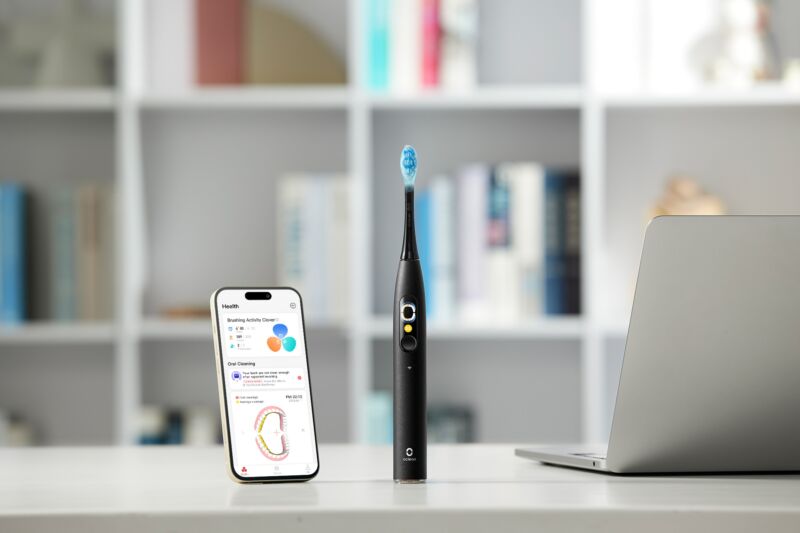
So-called "AI toothbrushes" have become more common since debuting in 2017.
Numerous brands now market AI capabilities for toothbrushes with three-figure
price tags. But there's limited scientific evidence that AI algorithms help
oral health, and companies are becoming more interested in using tech-laden
toothbrushes to source user data. ... Tech-enabled toothbrushes bring privacy
concerns to a product that has historically had zero privacy implications. But
with AI toothbrushes, users are suddenly subject to a company's privacy policy
around data and are also potentially contributing to a corporation's
marketing, R&D, and/or sales tactics. Privacy policies from toothbrush
brands Colgate-Palmolive, Oral-B, Oclean, and Philips all say the companies'
apps may gather personal data, which may be used for advertising and could be
shared with third parties, including ad tech companies and others that may
also use the data for advertising. These companies' policies say users can opt
out of sharing data with third parties or targeted advertising, but it's
likely that many users overlook the importance of reading privacy policies for
a toothbrush.
4 Strategies for Banks and Their Tech Partners that Save Money and Angst
When it comes to technological alignment between banks and tech partners, it’s
about more than ensuring tech stacks are compatible. Cultural alignment on
work styles, development cycles and more go into making things work. Both
partners should be up front about their expectations. For example, banking
institutions have more regulatory and administrative hurdles to jump through
than technology companies. While veteran fintech companies will be aware and
prepared to move in a more conservative way, early-stage technology companies
may be quicker to move and work in more unconventional ways. Prioritization of
projects on both ends should always be noted in order to set realistic
expectations. For example, tech firms typically have a large pipeline of
onboarding ahead. And the financial institution typically has limited tech
resources to allocate towards project management. ... Finally, when tech firms
and financial institutions work together, a strong dose of reality helps. View
upfront costs as a foundation for future returns. Community banking and credit
union leaders should focus on the potential benefits and value generation
expected three to five years after the project begins.
US Army moves closer to fielding next-gen biometrics collection
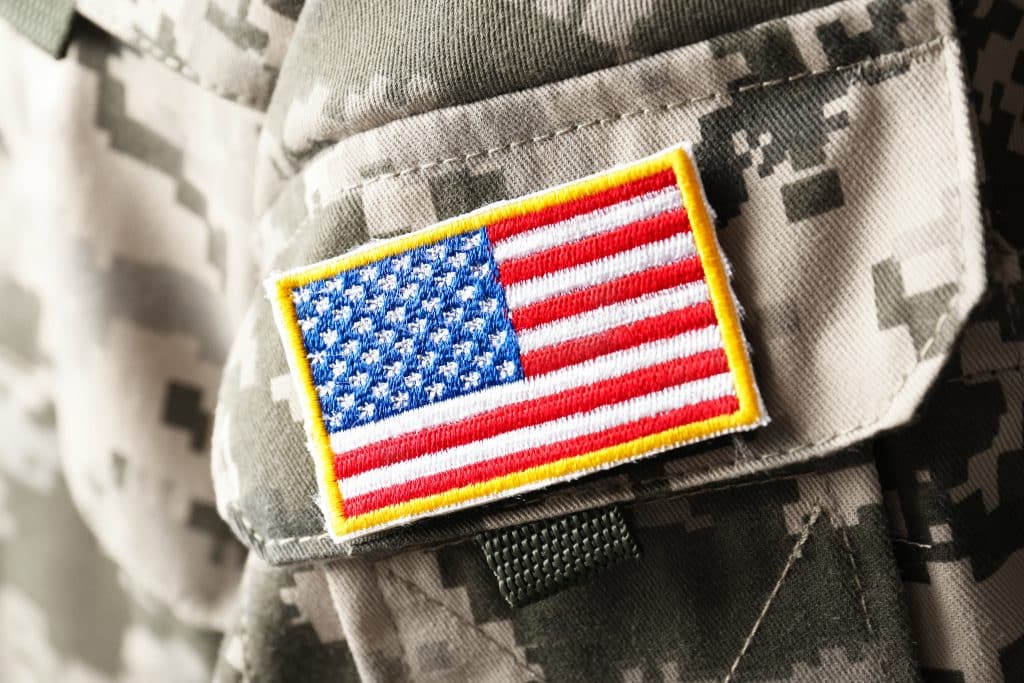
Specifically designed to be the Army’s forward biometrics collection and
matching system, NXGBCC has been designed to support access control, identify
persons of interest, and to provide biometric identities to detainee and
intelligence systems. NXGBCC collects, matches, and stores biometric
identities and is comprised of three components: a mobile collection kit,
static collection kit, and a local trusted source. ... The Army said “NXGBCC
will add to the number of biometric modalities collected, provide matches to
the warfighter in less than three minutes, increase the data sharing
capability, and reduce weight, power, and cost.” NXGBCC will use a Local
Trusted Source that is composed of a distributed database that’s capable of
being used worldwide, data management software, forward biometric matching
software, and an analysis portal. Also, NXGBCC collection kit(s) will be
composed of one or more collection devices, a credential/badge device, and
document scanning device. The NXGBCC system employs an integrated system of
commercial-off-the-shelf hardware and software that is intended to ensure the
end-to-end data flow that’s required to support different technical landscapes
during multiple types of operational missions.
The Future of AI: Edge Computing and Qualcomm’s Vision
AI models are becoming increasingly powerful while also getting smaller and
more efficient. This advancement enables them to run on edge devices without
compromising performance. For instance, Qualcomm’s latest chips are designed
to handle large language models and other AI tasks efficiently. These chips
are not only powerful but also energy-efficient, making them ideal for mobile
devices. One notable example is the Galaxy S24 Ultra, which is equipped with
Qualcomm’s Snapdragon 8 Gen 3 chip. This device can perform various AI tasks
locally, from live translation of phone calls to AI-assisted photography.
Features like live translation and chat assistance, which include tone
adjustment, spell check, and translation, run directly on the device,
showcasing the potential of edge computing. ... The AI community is also
contributing to this trend by developing open-source models that are smaller
yet powerful. Innovations like the Mixture of Agents, which allows multiple
small AI agents to collaborate on tasks, and Route LLM, which orchestrates
which model should handle specific tasks, are making AI more efficient and
accessible.
Software Supply Chain Security: Are You Importing Problems?

In a sense, Software Supply Chain as a strategy, just like Zero Trust, cannot
be bought off-the-shelf. It requires a combination of careful planning,
changing the business processes, improving communications with your suppliers
and customers and, of course, a substantial change in regulations. We are
already seeing the first laws introducing stronger punishment for
organizations involved in critical infrastructure, with their management
facing jail time for heavy violations. Well, perhaps the very definition of
“critical” must be revised to include operating systems, public cloud
infrastructures, and cybersecurity platforms, considering the potential global
impact of these tools on our society. ... To his practical advice I can
only add another bit of philosophical musing: security is impossible without
trust, but too much trust is even more dangerous than too little security.
Start utilizing the Zero Trust approach for every relationship with a
supplier. This can be understood in various ways: from not taking any
marketing claim at its face value and always seeking a neutral 3rd party
opinion to very strict and formal measures like requiring a high Evaluation
Assurance Level of the Common Criteria (ISO 15408) for each IT service or
product you deploy.
A CISO’s Observations on Today’s Rapidly Evolving Cybersecurity Landscape
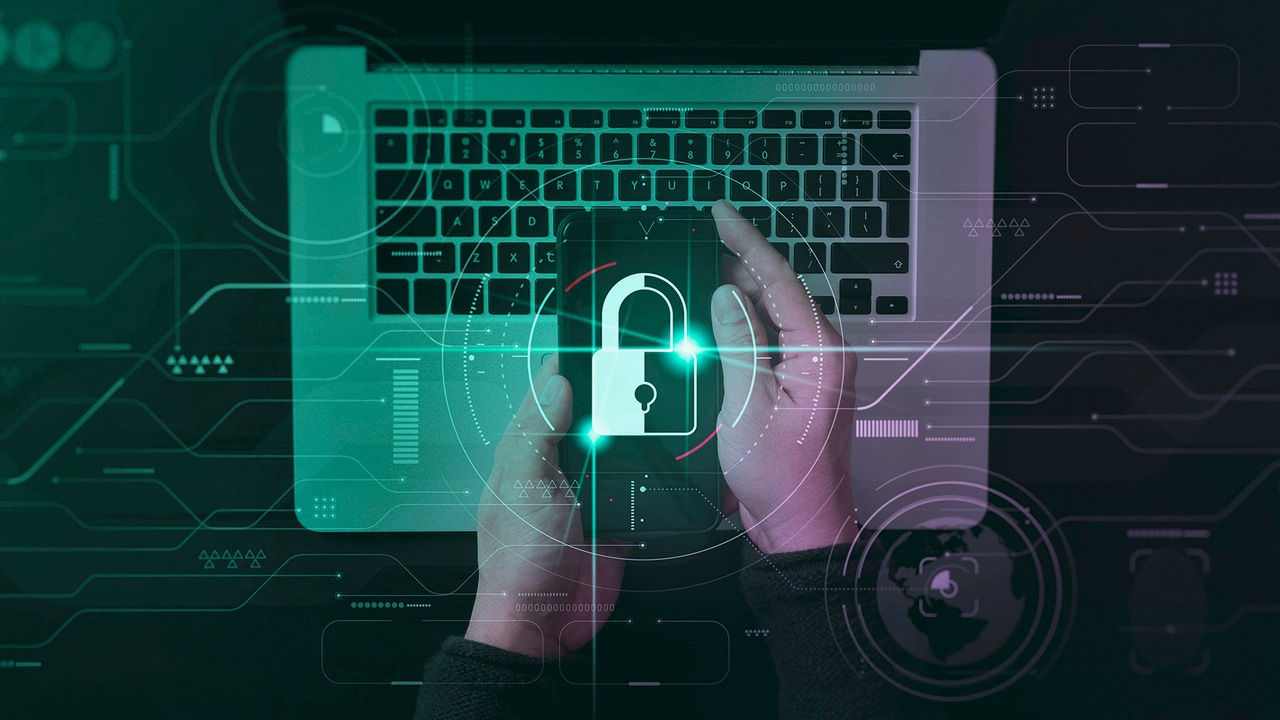
Simply being aware of risks isn’t sufficient. But, role-relevant security
simulations will empower the entire workforce to know what to do and how to
act when they encounter malicious activity. ... Security should be a smooth
process, but it is often complicated. Recall the surge in phishing attacks:
employees know not to click dubious links from unknown senders, but do they
know how to verify if a link is safe or unsafe beyond their gut instinct? Is
the employee aware that there is an official email verification tool? Do they
even know how to use it? ... It is not uncommon for business leaders to rush
technology adoption, delaying security until later as an added feature bolted
on afterward. When companies prioritize speed and scalability at the expense
of security, data becomes more mobile and susceptible to attack, making it
more difficult for security teams to ascertain the natural limitation of a
blast radius. Businesses may also end up in security debt. ... Technology
continues to evolve at breakneck speed, and organizations must adapt their
security strategy appropriately. As such, businesses should adopt a
multifaceted, agile, and ever-evolving cybersecurity approach to managing
risks.
Future AI Progress Might Not Be Linear. Policymakers Should Be Prepared.
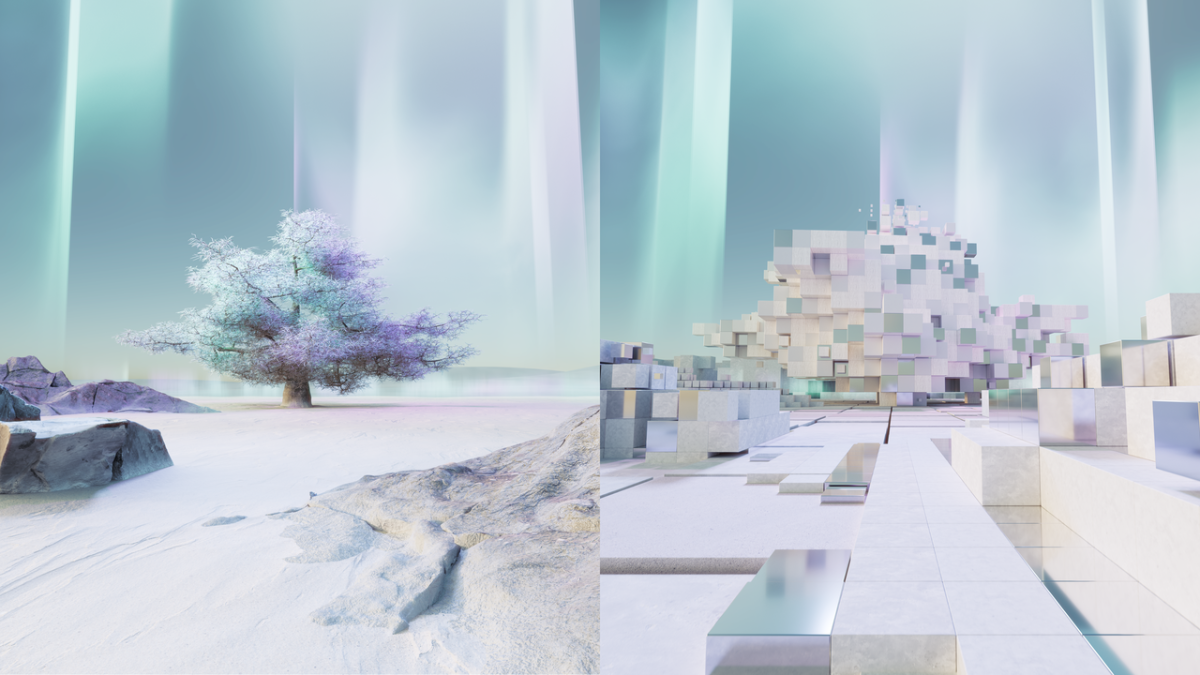
Policymakers and their advisors can act today to address that risk. Firstly,
though it might be politically tempting, they should be mindful of overstating
the likely progress and impact of current AI paradigms and systems. Linear
extrapolations and quickfire predictions make for effective short-term
political communication, but they carry substantial risk: If the next
generation of language models is, in fact, not all that useful for
bioterrorism; if they are not readily adopted to make discriminatory
institutional decisions; or if LLM agents do not arrive in a few years, but we
reach slowing progress or a momentary plateau instead, policymakers and the
public will take note – and be skeptical of warnings in the future. If
nonlinear progress is a realistic option, then policy advocacy on AI should
proactively consider it: hedge on future predictions, conscientiously name the
possibility of plateaus, and adjust policy proposals accordingly. Secondly,
the prospect of plateaus makes reactive and narrow policy-making much more
difficult. Their risk is instead best addressed by focusing on building up
capacity: equip regulators and enforcement with the expertise, access and
tools they need to monitor the state of the field.
Building the data center of the future: Five considerations for IT leaders
Disparate centers of data are, in turn, attracting more data, leading to Data
Gravity. Localization needs and a Hybrid IT infrastructure are creating
problems related to data interconnection. Complex systems require an
abstraction layer to move data around to fulfill fast-changing computing
needs. IT needs interconnection between workflow participants, applications,
multiple clouds, and ecosystems, all from a single interface, without getting
bogged down by the complexity wall. ... Increasing global decarbonization
requirements means data centers must address energy consumption caused by
high-density computing. ... Global variations in data handling and
privacy legislation require that data remain restricted to specific
geographical regions. Such laws aren't the only drivers for data localization.
The increasing use of AI at the edge, the source of the data, is driving
demand for low-latency operations, which in turn requires localized data
storage and processing. Concerns about proprietary algorithms being stored in
the public cloud are also leading companies to move to a Hybrid IT
infrastructure that can harness the best of all worlds.
Quote for the day:
"Perseverance is failing 19 times and
succeding the 20th." -- Julie Andrews
No comments:
Post a Comment