Enterprise Architecture – Supporting Resources on Demand
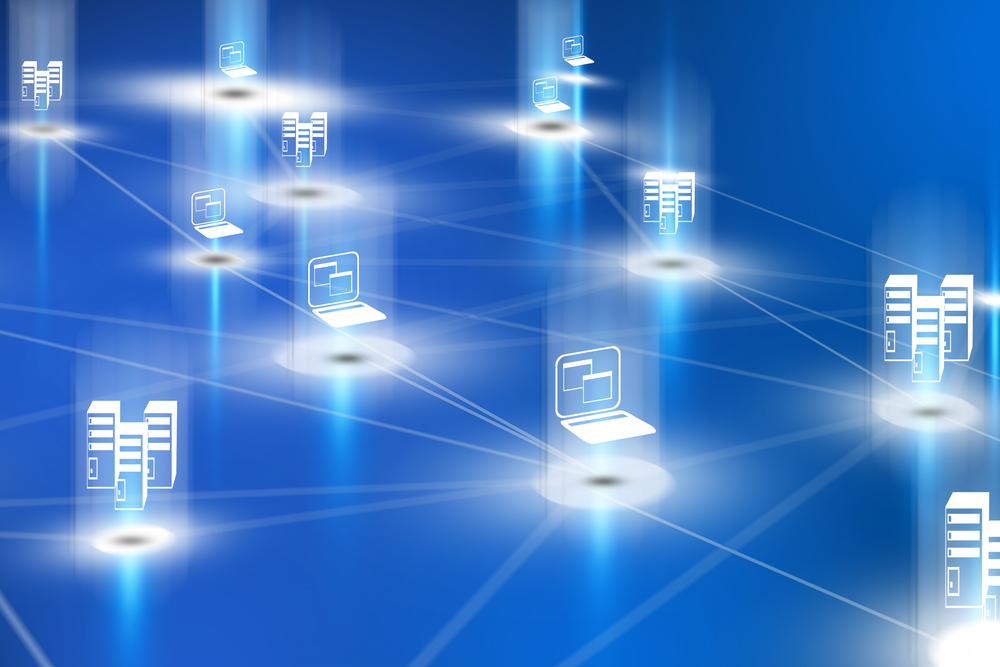
As the subscription economy grows, the market could become saturated with
providers offering varying levels of service quality. Businesses should
carefully evaluate their options, considering factors such as customer support,
scalability, and the sophistication of available resources. The positive impact
of selling EA as a subscription service, however, is clear. With more service
providers offering cloud solutions, there is more competition for your business.
You, as the business customer, have more options, which can lead to better
services and pricing. Business customers of all sizes can get access to advanced
technology and data storage capabilities through a subscription. This can open
economic doors to developing nations, allowing business growth to more players
who would otherwise not be able to participate in a digital transformation
journey. This fosters a more inclusive and diverse tech landscape, where
breakthroughs can emerge from unexpected corners of the business world. You can
focus on growing your core business without the traditional burdens of upfront
investment and the complexity of building and managing infrastructure from
scratch.
Trends in Data Governance and Security: What to Prepare for in 2024
In 2023, many companies turned to do-it-yourself (DIY) data governance to manage
their data. Yet, without seeking the help of data governance experts or
professionals, this proved to be insufficient due to compliance gaps and the
data security errors it leaves in its wake. While do-it-yourself data governance
seemed like a cost-effective solution, it has serious consequences for companies
leaving them exposed to data breaches and other security threats. This is
because DIY data governance often lacks the comprehensive security protocols and
expertise that professional data governance provides leading to both data
breaches and other security threats. Worse, the approach often involves
piecemeal solutions that do not integrate well with each other, creating
security gaps and leaving data vulnerable to attack. As a result, DIY data
governance may not be able to keep up with the constantly evolving data privacy
landscape, including new regulations and compliance requirements. Companies that
rely on do-it-yourself data governance are exposing themselves to significant
risks and will see the repercussions of this in 2024.
Generative AI is off to a rough start

One big problem, among several others that Duckbill Chief Economist Corey Quinn
highlights, is that although AWS felt compelled to position Q as significantly
more secure than competitors like ChatGPT, it’s not. I don’t know that it’s
worse, but it doesn’t help AWS’ cause to position itself as better and then not
actually be better. Quinn argues this comes from AWS going after the application
space, an area in which it hasn’t traditionally demonstrated strength: “As soon
as AWS attempts to move up the stack into the application space, the wheels fall
off in major ways. It requires a competency that AWS does not have and has not
built up since its inception.” Perhaps. But even if we accept that as true, the
larger issue is that there’s so much pressure to deliver on the hype of AI that
great companies like AWS may feel compelled to take shortcuts to get there (or
to appear to get there). The same seems to be true of Google. The company has
spent years doing impressive work with AI yet still felt compelled to take
shortcuts with a demo. As Parmy Olson captures, “Google’s video made it look
like you could show different things to Gemini Ultra in real time and talk to
it. You can’t.”
CIOs grapple with the ethics of implementing AI

Even with a team focused on AI, identifying risks and understanding how the
organization intends to use AI both internally and publicly is challenging,
McIntosh says. Team members must also understand and address the inherent
possibility of AI bias, erroneous claims, and incorrect results, he says.
“Depending on the use cases, the reputation of your company and brand may be at
stake, so it’s imperative that you plan for effective governance.” With that in
mind, McIntosh says it’s critical that CIOs “don’t rush to the finish line.”
Organizations must create a thorough plan and focus on developing a governance
framework and AI policy before implementing and exposing the technology.
Identifying appropriate stakeholders, such as legal, HR, compliance and privacy,
and IT, is where Plexus started its ethical AI process, McIntosh says. “We then
created a draft policy to outline the roles and responsibilities, scope,
context, acceptable use guidelines, risk tolerance and management, and
governance,” he says. “We continue to iterate and evolve our policy, but it is
still in development. We intend to implement it in Q1 2024.”
Accenture takes an industrialized approach to safeguarding its cloud controls

Accenture developed a virtual cloud control factory to support five major,
global cloud infrastructure providers and enable reliable inventory; consistent
log and alert delivery to support security incident detection; and predictable,
stable, and repeatable processes for certifying cloud services and releasing
security controls. The factory features five virtual "departments". There's
research and development, which performs service certification, control
definition, selection, measurement, and continual re-evaluation; the production
floor designs and builds control; quality assurance tests the controls; shipping
and receiving integrates controls with compliance reporting tools; and customer
service provides support to users after a control goes live. "What we decided to
do was centralize that cloud control development, get all the needs into one
place, start organizing them in a way that we could run them through a factory
and get them out there so people can use common controls, common architecture
that had a chance of keeping up with [our engineers'] innovation sitting on top
of the [major cloud platforms'] innovation," Burkhardt says
Pressure on Marketers Will Drive Three Key Data Moves in 2024
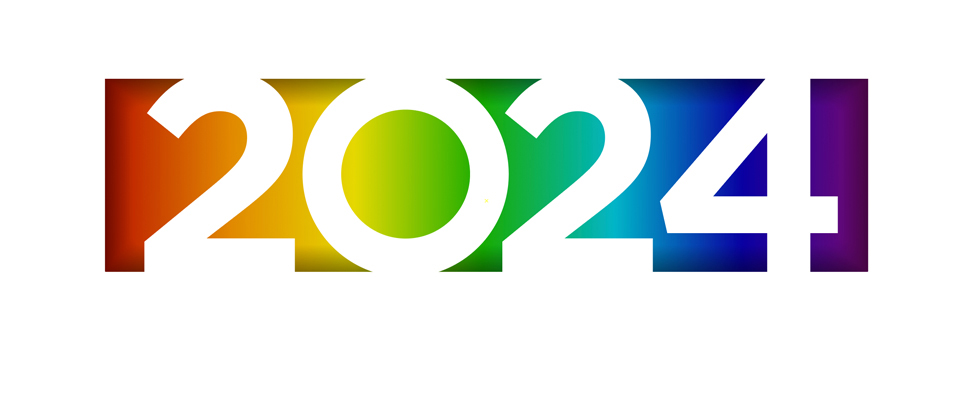
Data clouds help achieve that goal. In both time and expense, organizations can
no longer afford to jump between different systems to try to make sense of what
a customer wants and formulate a real-time response in the moment of
interaction. With a CDP sitting directly on top of a data cloud, it is easier
and less expensive to build a unique customer profile and then activate that
profile across multiple systems. Organizations recognize that first-party data
is a valuable asset and is the foundation for delivering a personalized customer
experience (CX), but for too long business users have been stymied by complex,
unintegrated marketing stacks and time-consuming data transformations. That
approach to making data actionable -- turning data into insight -- is no longer
sustainable when customers expect real-time, personalized experiences that are
consistent across channels. ... Moving to a data cloud and coupling it with a
CDP’s automated data quality and identity resolution addresses these issues
head-on, and that trend will continue -- particularly for customer-facing brands
that see a data cloud with an enterprise-grade CDP as a relatively fast,
inexpensive way to monetize their customer data.
Initial Agile Requirements and Architecture Modeling
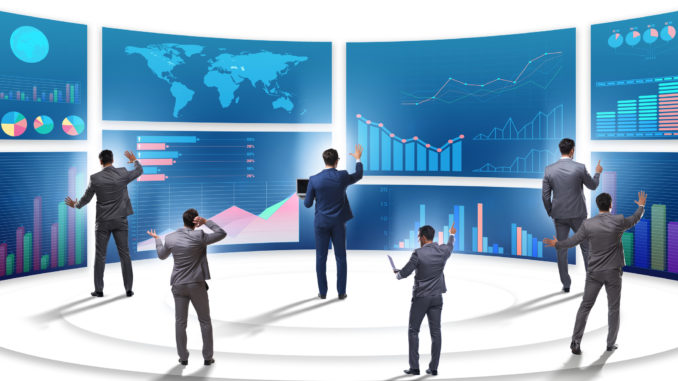
Talk to most agilists, and particularly the purists, and they’ll claim that
they don’t do any modeling up front. This of course is completely false, they
just use different terminology such as “populate the backlog” rather than
initial requirements modeling and “identify a runway” instead of initial
architecture modeling. Sigh. Some of the more fervent agilists may even tell
you about the evils of big modeling up front which is why they choose to
eschew anything that smells like up-front thinking. ... The goal of initial
architecture modeling on an agile team is to identify what the team believes
to be a viable strategy for building the solution. Sufficiency is determined
by your stakeholders – Can you exhibit an understanding of the existing
environment, and the future direction of your organization, and show how your
proposed strategy reflects that? Your initial architecture model should be
JBGE in that it addresses, at a high-level, the business and technical
landscapes that your solution will operate within. This modeling effort is
often led, not dictated, by the architecture owner on your team.
Why are IT professionals not automating?
25% of participants highlighted cost and resource as potential obstacles. They
wonder if they need to create a custom solution and, if so, whether it’s
cost-effective or cheaper to continue with manual maintenance. They are also
concerned about the resources required to maintain an automated solution. 20%
admit that they and their teams lack the knowledge or expertise to choose an
automated solution. They are not familiar with automation in general or the
specific requirements of automating their systems. The survey results clearly
indicate that many IT professionals are not familiar with or don’t see the
value of certificate automation. Or is it that they didn’t think about it
enough? After all, certificates have been part of our IT infrastructure for a
very long time, while they are not exciting, they do work, so why fix
something that is not broken? Unfortunately, when the 90-day Google edict
eventually becomes reality, it will increase the need for renewal/replacement
of SSL/TSL certificates by four times (4X) the current pace. IT professionals
may be underestimating the burden that it will put on their teams.
How Could AI Be a Tool for Workers?
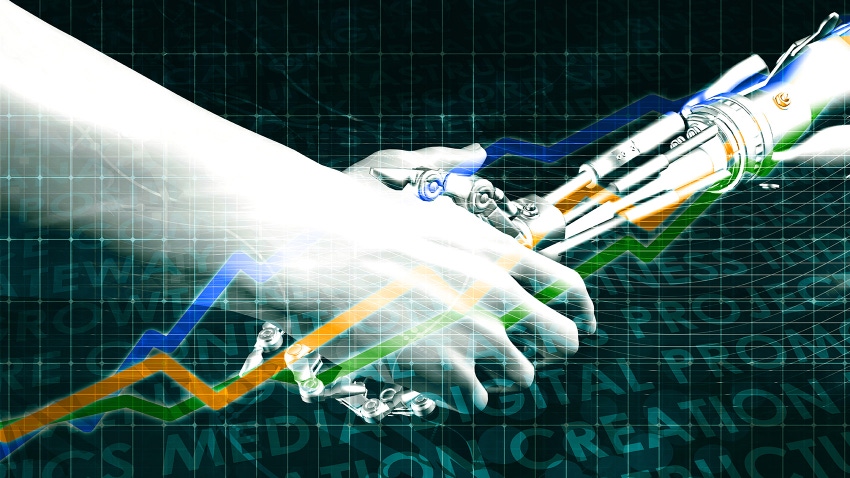
The benefits for companies designing and using AI systems are vast and readily
apparent. Tools that can complete work in a fraction of the time at a fraction
of the cost are a boon for the bottom line. “The main beneficiaries of the
technology are global technology giants primarily based in the United States,”
says Michael Allen, CTO of enterprise content management company Laserfiche.
He points out that these companies have the resources to accrue the massive
amounts of data required to train AI models. Companies that adopt these
powerful AI models can leverage them to cut costs. Allen points out that many
companies will likely use AI to shift away from outsourcing. “A lot of firms
outsource mostly routine clerical work to places like India, and I believe
that's going to be threatened or impacted significantly by AI that will be
able to do that work faster and cheaper,” he says. The way that AI devalues
entry-level work is already being seen. Stephanie Bell is a senior research
scientist at the nonprofit coalition Partnership on AI, which created
guidelines to ensure AI economic benefits are shared. She offers examples in
the digital freelance market.
Bryan Cantrill on AI Doomerism: Intelligence Is Not Enough
Cantrill had titled his talk “Intelligence is not enough: the humanity of
engineering.” Here the audience realizes they’re listening to the proud CTO of
a company that just shipped its own dramatically redesigned server racks. “I
want to focus on what it takes to actually do engineering… I actually do have
a bunch of recent experience building something really big and really hard as
an act of collective engineering…” Importantly, the common thread for these
bugs was “emergent” properties — things not actually designed into the parts,
but emerging when they’re all combined together. “For every single one of
those, there is no piece of documentation. In fact, for several of those, the
documentation was actively incorrect. The documentation would mislead
you ... Cantrill put up a slide saying “Intelligence alone does not solve
problems like this,” presenting his team at Oxide as possessed of something
uniquely human. “Our ability to solve these problems had nothing to do with
our collective intelligence as a team…” he tells his audience. “We had to
summon the elements of our character. Not our intelligence — our
resilience.”
Quote for the day:
“I'd rather be partly great than
entirely useless.” -- Neal Shusterman
No comments:
Post a Comment