Navigating the Crossroads of Data Confidentiality and AI
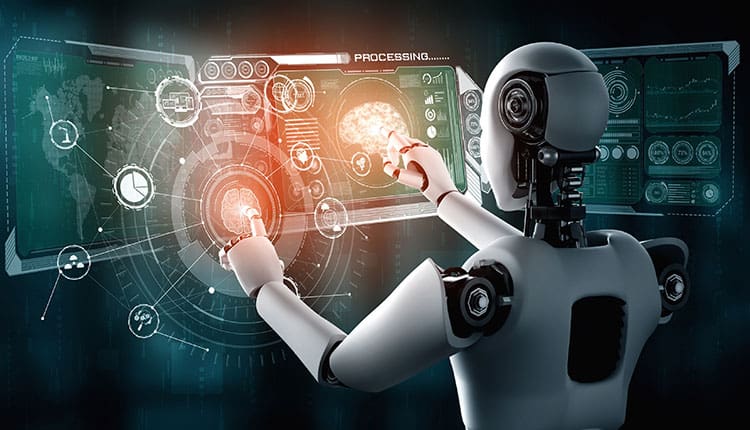
Striking a balance between ensuring data privacy and maximizing the
effectiveness of AI models can be quite complex. The more data we utilize for
training AI systems, the more accurate and powerful they become. However, this
practice often clashes with the need to safeguard privacy rights. Techniques
like federated learning offer a solution by allowing AI models to be trained on
data sources without sharing raw information. For the uninitiated, Federated
Learning leverages the power of edge computing to train local models. These
models use data that never leaves the private environment (like your phone, IoT
devices, corporate terminals, etc.). Once the local models are trained, they are
then leveraged to build a centralized model that can be used for related use
cases. ... Due to the recent acceleration in the adoption of AI, government
regulations play a pivotal role in shaping the future of AI and data
confidentiality. Legislators are increasingly recognizing the significance of
data privacy and are implementing laws such as the General Data Protection
Regulation (GDPR) in Europe and the California Consumer Privacy Act (CCPA).
CISOs vs. developers: A battle over security priorities
“Developers and CISOs juggle numerous security priorities, often conflicting
across organizations,” noted Luke Shoberg, Global CISO at Sequoia Capital. “The
report emphasizes the need for internal assessments, fostering deeper
collaboration, and building trust among teams managing this critical domain.
Recognizing technical and cultural obstacles, organizations have made
significant strides in understanding the importance of securing the software
supply chain for sustained business success.” “The world of software consumption
and security has radically changed. From containers to the explosion of open
source components, every motion has been toward empowering developers to build
faster and better,” said Avon Puri, Global Chief Digital Officer at Sequoia
Capital. “But with that progress, the security paradigm has been challenged to
refocus on better controls and guarantees for the provenance of where software
artifacts come from and that their integrity is being maintained. The survey
shows developers and security teams are wrestling with this new reality in the
wake of major exploits like Log4j and SolarWinds.
Deception technology use to grow in 2024 and proliferate in 2025

It's worth mentioning that all scanning, data collection, processing, and
analysis will be continuous to keep up with changes to the hybrid IT
environment, security defenses, and the threat landscape. When organizations
implement a new SaaS service, deploy a production application, or make changes
to their infrastructure, the deception engine notes these changes and adjusts
its deception techniques accordingly. Unlike traditional honeypots, burgeoning
deception technologies won't require cutting-edge knowledge or complex setup.
While some advanced organizations may customize their deception networks, many
firms will opt for default settings. In most cases, basic configurations will
sufficiently confound adversaries. Remember, too, that deception elements like
decoys and lures remain invisible to legitimate users. Therefore, when someone
goes poking at a breadcrumb or canary token, you are guaranteed that they are up
to no good. In this way, deception technology can also help organizations
improve security operations around threat detection and response.
What Role Will Open-Source Hardware Play in Future Designs?
The extent of open-source hardware’s impact on electronics design is still
uncertain. While it could likely lead to all these benefits, it also faces
several challenges to mainstream adoption. The most significant of these is the
volatility and high costs of the necessary raw materials. Roughly 70% of all
silicon materials come from China. This centralization makes prices prone to
fluctuations from local disruptions in China or throughout the supply chain.
Similarly, long shipping distances raise related prices for U.S. developers.
Even if integrated circuit design becomes more accessible, these costs keep
production inaccessible, slowing open-source devices’ growth. Similarly,
industry giants may be unwilling to accept the open-source movement. While
open-source designs open new revenue streams, these market leaders profit
greatly from their proprietary resources. The semiconductor fabs supporting
these large companies are even more centralized. It may be difficult for
open-source hardware to compete if these organizations don’t embrace the
movement.
How Should Developers Respond to AI?

“Unionizing against AI” wasn’t a specific goal, Quick clarified in an email
interview with The New Stack. He’d meant it as an example of the level of just
how much influence can come from a united community. “My main thought is around
the power that comes with a group of people that are working together.” Quick
noted what happened when the United Auto Workers went on strike. “We are seeing
big changes happening because the people decided collectively they needed more
money, benefits, etc. I can only begin to guess at what an AI-related scenario
would be, but maybe in the future, it takes people coming together to push for
change on regulation, laws, limitations, etc.” Even this remains a concept more
than any tangible movement, Quick stressed in his email. “Honestly, I don’t have
much more specific actions or goals right now. We’re just so early on that all
we can do is guess.” But there is another scenario where Quick thinks community
action would be necessary to push for change: the hot-button issue of “who owns
the code.”
Security, privacy, and generative AI
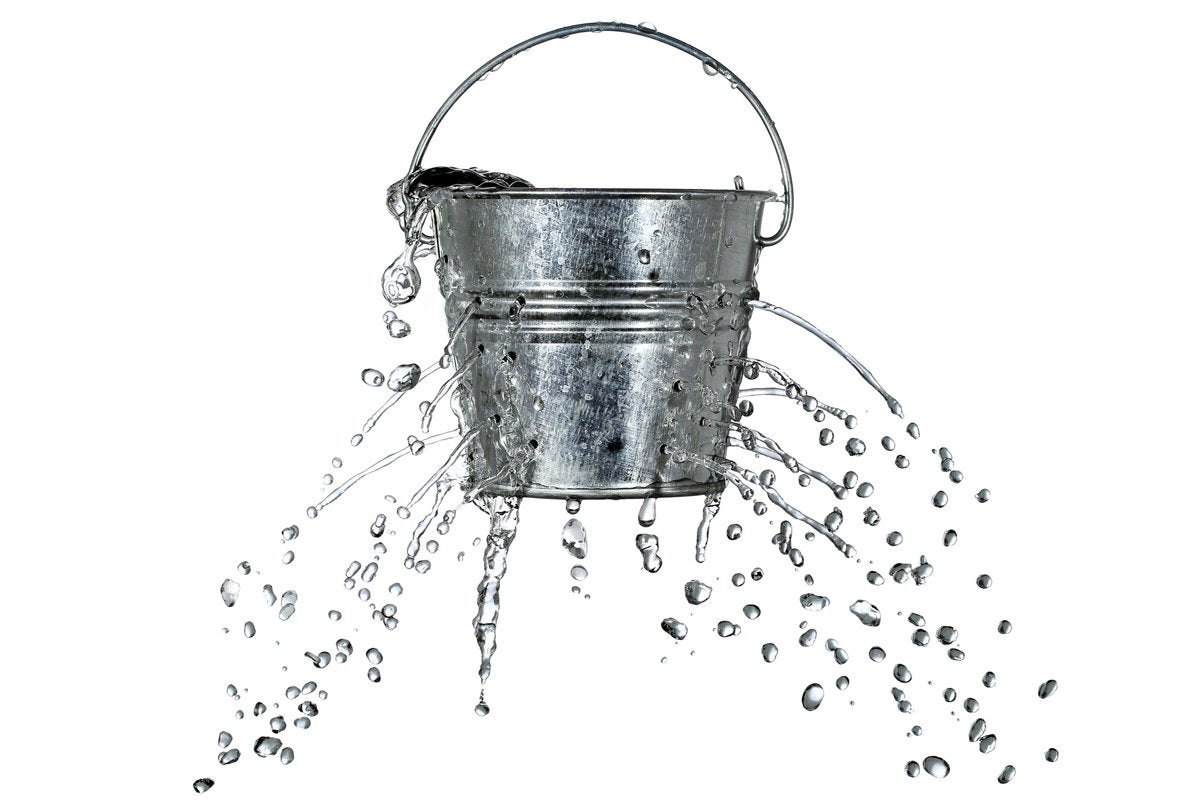
For many of the proposed applications in which LLMs should excel, delivering
false responses can have serious consequences. Luckily, many of the mainstream
LLMs have been trained on numerous sources of data. This allows these models to
speak on a diverse set of topics with some fidelity. However, there is typically
insufficient knowledge around specialized domains in which data is relatively
sparse, such as deep technical topics in medicine, academia, or cybersecurity.
As such, these large base models are typically further refined via a process
called fine-tuning. Fine-tuning allows these models to achieve better alignment
with the desired domain. Fine-tuning has become such a pivotal advantage that
even OpenAI recently released support for this capability to compete with
open-source models. With these considerations in mind, consumers of LLM products
who want the best possible outputs, with minimal errors, must understand the
data in which the LLM is trained (or fine-tuned) to ensure optimal usage and
applicability.
How to keep remote workers connected to company culture
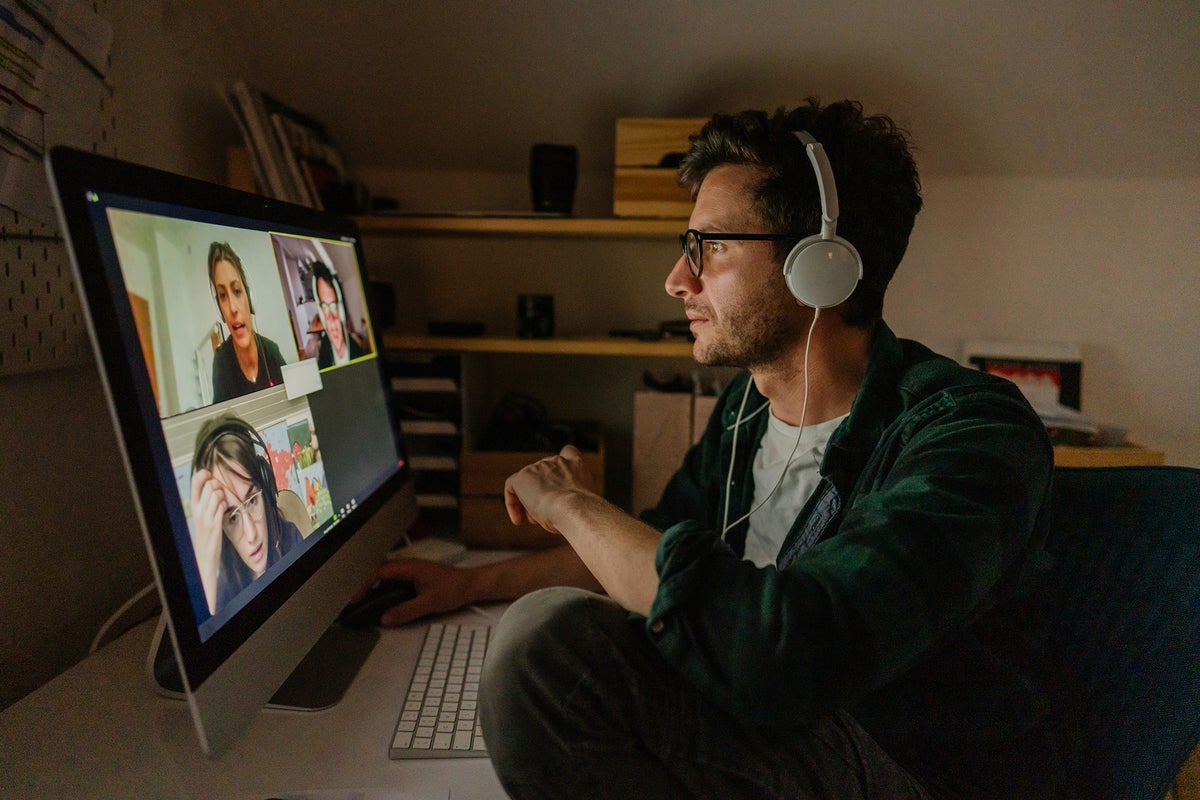
As important as workplace collaboration and communication tools are, technology
alone can’t keep remote workers engaged with business objectives. Before the
pandemic, auto finance firm Credit Acceptance centered its operations around
in-person interactions in its offices, for which it got accolades; after
COVID-19 arrived, the company’s 2,200 employees had to work remotely. “You
didn't work from home at all – [only in] rare circumstances,” said Wendy
Rummler, chief people officer at Credit Acceptance. “We considered our culture
too important, [we believed that] we couldn't maintain it if we had a fully
remote workforce, or even partially for that matter.” Fast forward a couple of
years and the picture is markedly different now, with almost all staffers now
fully remote. Internal pulse surveys have found that employee engagement has
remained as high as before the pandemic, said Rummler. This is no accident, she
said; Credit Acceptance deliberately set out to maintain its work culture
without regular person-to-person interactions.
Should AI Require Societal Informed Consent?
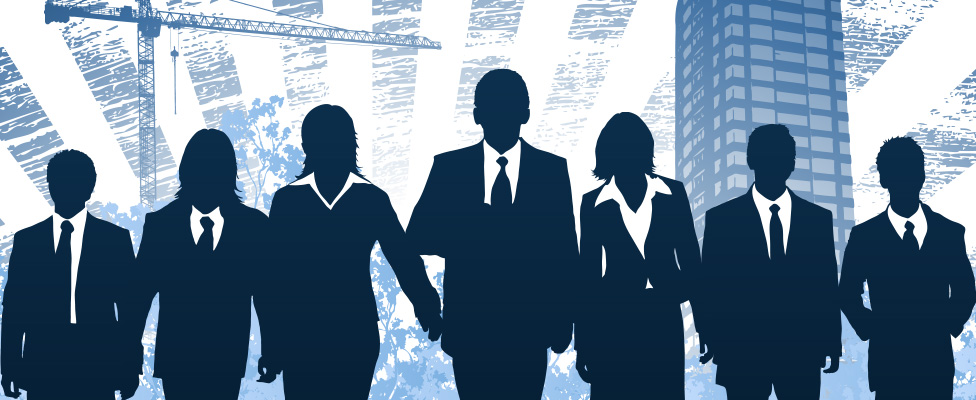
The concept of societal informed consent has been discussed in engineering
ethics literature for more than a decade, and yet the idea has not found its way
into society, where the average person goes about their day assuming that
technology is generally helpful and not too risky. In most cases, technology is
generally helpful and not too risky, but not in all cases. As artificial
intelligence grows more powerful and is applied to more new fields (many of
which may be inappropriate), these cases will multiply. How will technology
producers know when their technologies are not wanted if they never ask the
public? ... One of the characteristics of a representative democracy is that --
at least in theory -- our elected officials are looking out for the well-being
of the public. ... It is time for the government and the public to have a new
conversation, one about technology -- specifically artificial intelligence. In
the past we’ve always given technology the benefit of the doubt; tech was
“innocent until proven guilty” and a long-time familiar phrase in and around
Silicon Valley has been “it’s better to ask forgiveness, not permission.” We no
longer live in that world.
Harnessing the potential of generative AI in marketing

Augmenting human creativity with the power of generative AI holds so much
promise that the use cases we know now are only the tip of the proverbial
iceberg. Companies that are looking to get a head start should, therefore,
ensure that they have laid down the foundations for doing so. An important
consideration in deploying generative AI is the availability of data.
Contextualisation is a key benefit of generative AI and large language models
(LLMs). But for enterprises with legacy, on-premise systems, their data is
usually isolated within silos. Organisations looking to deploy generative AI
solutions for their marketing efforts should leverage cloud data platforms to
unify all their internal data. Aside from breaking down silos, businesses should
also ensure seamless access to all their data. A lot of the data generated by
marketing teams is either unstructured or semi-structured; such as social media
posts, emails, and text documents, to name a few. Marketing teams should ensure
that their cloud data platforms can load, integrate, and analyse all types of
data.
Managing Missing Data in Analytics
Missing at Random (MAR) is a very common missing data situation encountered by
data scientists and machine learning engineers. This is mainly because MCAR and
MNAR-related problems are handled by the IT department, and data issues are
addressed by the data team. MAR data imputation is a method of substituting
missing data with a suitable value. Some commonly used data imputation methods
for MAR are:In hot-deck imputation, a missing value is imputed from a randomly
selected record coming from a pool of similar data records. In hot-deck
imputation, the probabilities of selecting the data are assumed equal due to the
random function used to impute the data. In cold-deck imputation, the random
function is not used to impute the value. Instead, other functions, such as
arithmetic mean, median, and mode, are used. With regression data imputation,
for example, multiple linear regression (MLR), the values of the independent
variables are used to predict the missing values in the dependent variable by
using a regression model. Here, first the regression model is derived, then the
model is validated, and finally the new values, i.e., the missing values, are
predicted and imputed.
Quote for the day:
"Failure isn't fatal, but failure to
change might be" -- John Wooden
No comments:
Post a Comment