Generative AI Faces an Existential IP Reckoning of Its Own Making
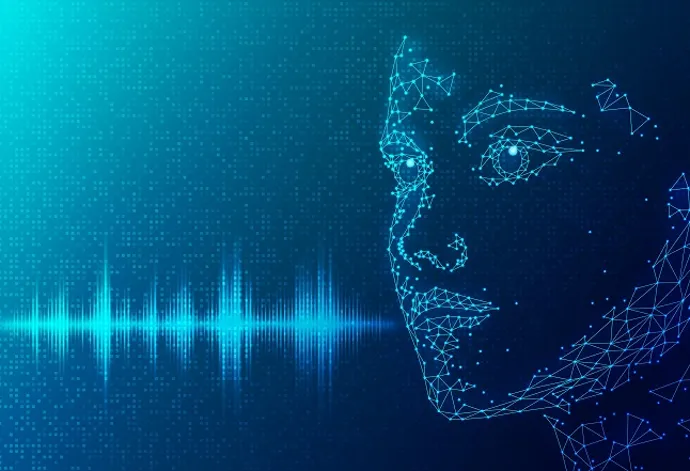
Clearly, this situation is untenable, with a raft of dire consequences already
beginning to emerge. Should the courts determine that generative AI firms aren’t
protected by the fair use doctrine, the still-budding industry could be on the
hook for practically limitless damages. Meanwhile, platforms like Reddit are
beginning to aggressively push back against unchecked data scraping.
... These sorts of unintended externalities will only continue to multiply
unless strong measures are taken to protect copyright holders. Government can
play an important role here by introducing new legislation to bring IP laws into
the 21st century, replacing outdated regulatory frameworks created decades
before anyone could have predicted the rise of generative AI. Government can
also spur the creation of a centralized licensing body to work with national and
international rights organizations to ensure that artists, content creators, and
publishers are being fairly compensated for the use of their content by
generative AI companies.
6 hidden dangers of low code
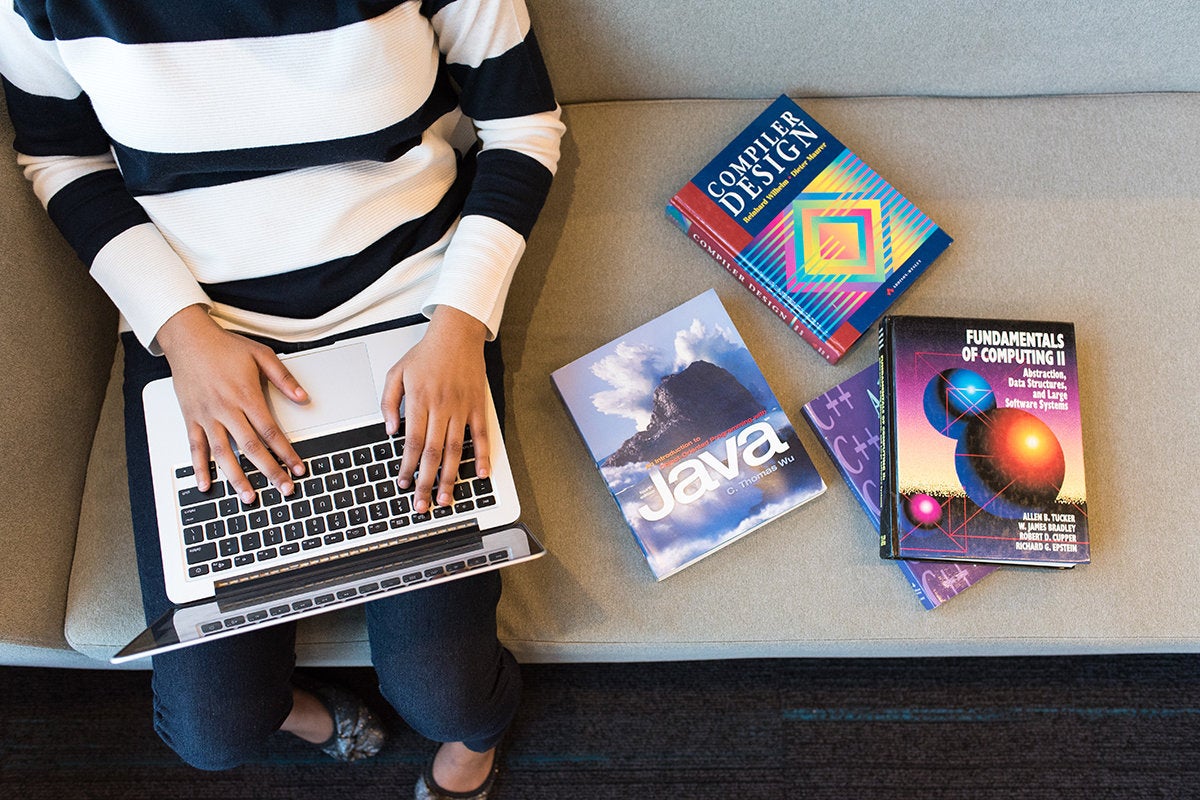
The low-code sales pitch is that computers and automation make humans smarter by
providing a computational lever that multiplies our intelligence. Perhaps. But
you might also notice that, as people grow to trust in machines, we sometimes
stop thinking for ourselves. If the algorithm says it’s the right thing to do,
we'll just go along with it. There are endless examples of the disaster that can
ensue from such thoughtlessness. ... When humans write code, we naturally do the
least amount of work required, which is surprisingly efficient. We're not
cutting corners; we're just not implementing unnecessary features. Low code
solutions don’t have that advantage. They are designed to be one-size-fits-all,
which in computer code means libraries filled with endless if-then-else
statements testing for every contingency in the network. Low code is naturally
less efficient because it’s always testing and retesting itself. This ability to
adjust automatically is the magic that the sales team is selling, after all. But
it’s also going to be that much less efficient than hand-tuned code written by
someone who knows the business.
Applying Reliability Engineering to the Manufacturing IT Environment
To understand exposure to failure, the Reliability Engineers analyzed common
failure modes across manufacturing operations, utilizing the Failure Mode and
Effects Analysis (FMEA) methodology to anticipate potential issues and failures.
Examples of common failure modes include “database purger/archiving failures
leading to performance impact” and “inadequate margin to tolerate typical
hardware outages.” The Reliability Engineers also identified systems that were
most likely to cause factory impact due to risk from these shared failure modes.
This data helped inform a Resiliency Maturity Model (RMM), which scores each
common failure mode on a scale from 1 to 5 based on a system’s resilience to
that failure mode. This structured approach enabled us to not just fix isolated
examples of applications that were causing the most problems, but to instead
broaden our impact and develop a reliability mindset.
5 Skills All Marketing Analytics and Data Science Pros Need Today

Marketing analysts should hone their skills to know who to talk to – and how to
talk to them – to secure the information they have. Trust Insights’ Katie
Robbert says it requires listening and asking questions to understand what they
know that you need to take back to your team, audience, and stakeholders. “You
can teach anyone technical skills. People can follow the standard operating
procedure,” she says. “The skill set that is so hard to teach is communication
and listening.” ... By improving your communication skills, you’ll be
well-positioned to follow Hou’s advice: “Weave a clear story in terms of how
marketing data could and should guide the organization’s marketing team.” She
says you should tell a narrative that connects the dots, explains the how and
where of a return on investment, and details actions possible not yet realized
due to limited lines of sight. ... Securing organization-wide support requires
leaning into what the data can do for the business. “Businesspeople want to see
the business outcomes.
Neural Networks vs. Deep Learning

Neural networks, while powerful in synthesizing AI algorithms, typically require
less resources. In contrast, as deep learning platforms take time to get trained
on complex data sets to be able to analyze them and provide rapid results, they
typically take far longer to develop, set up and get to the point where they
yield accurate results. ... Neural networks are trained on data as a way of
learning and improving their conclusions over time. As with all AI deployments,
the more data it’s trained on the better. Neural networks must be fine-tuned for
accuracy over and over as part of the learning process to transform them into
powerful artificial intelligence tools. Fortunately for many businesses, plenty
of neural networks have been trained for years – far before the current craze
inspired by ChatGPT – and are now powerful business tools. ... Deep learning
systems make use of complex machine learning techniques and can be considered a
subset of machine learning. But in keeping with the multi-layered architecture
of deep learning, these machine learning instances can be of various types and
various strategies throughout a single deep learning application.
Ready or not, IoT is transforming your world
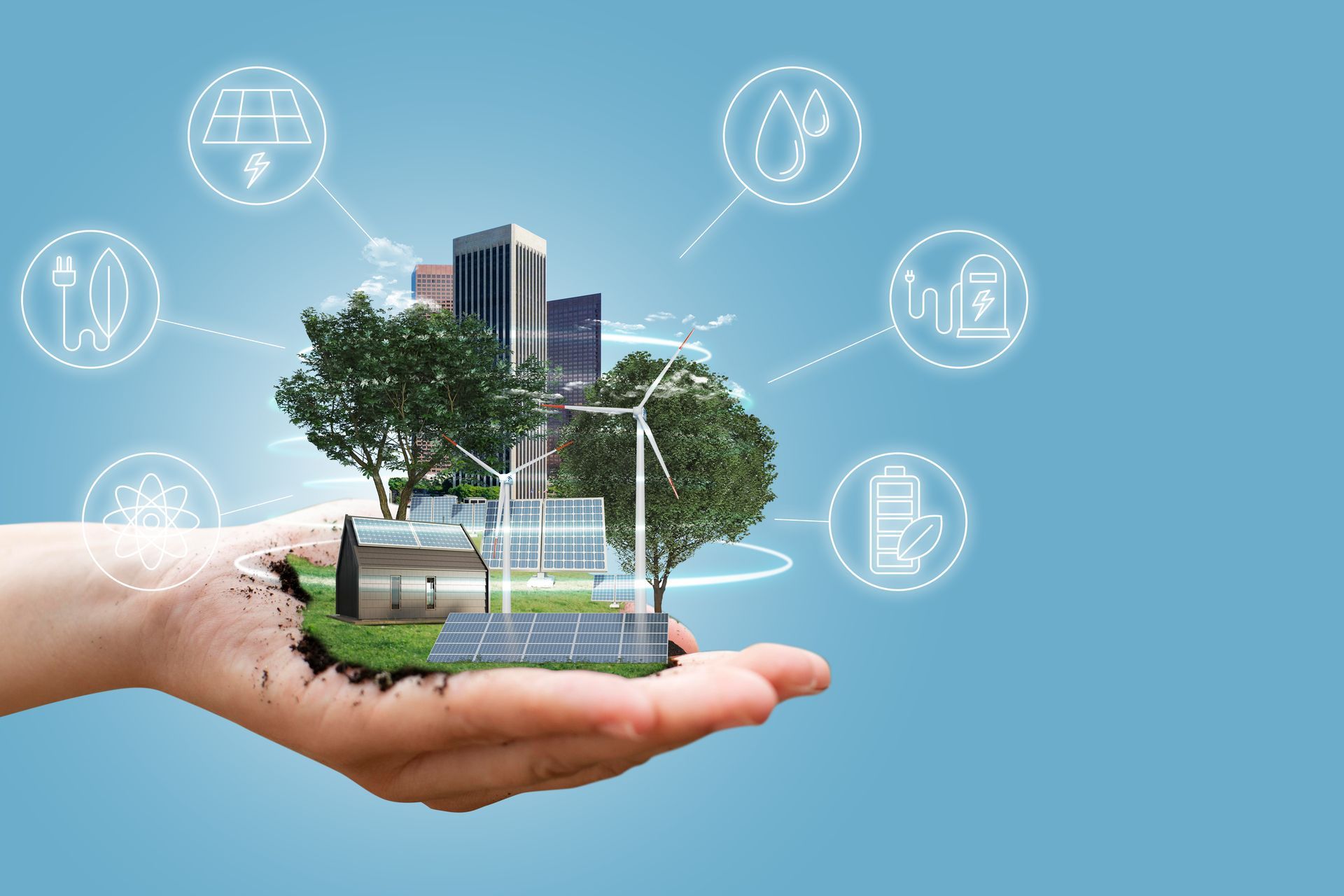
At its core, IoT refers to the interconnection of everyday objects, devices,
and systems through the internet, enabling them to collect, exchange, and
analyze data. This connectivity empowers us to monitor and control various
aspects of our lives remotely, from smart homes and wearable devices to
industrial machinery and city infrastructure. The essence of IoT lies in the
seamless communication between objects, humans, and applications, making our
environments smarter, more efficient, and ultimately, more convenient. ...
Looking ahead, the future of IoT holds remarkable potential. Over the next
five years, we can expect a multitude of advancements that will reshape
industries and lifestyles. Smart cities will continue to evolve, leveraging
IoT to enhance sustainability, security, and quality of life. The healthcare
sector will witness even more personalized and remote patient monitoring,
revolutionizing the way medical care is delivered. AI and automation will play
a pivotal role, in driving efficiency and innovation across various
domains.
What are network assurance tools and why are they important?

Without a network assurance tool at their disposal, many enterprises would be
forced to limit their network reach and capacity. "They would be unable to
take advantage of the latest technological advancements and innovations
because they didn’t have the manpower or tools to manage them," says Christian
Gilby, senior product director, AI-driven enterprise, at Juniper Networks. "At
the same time, enterprises would be left behind by their competitors because
they would still be utilizing manual, trial-and-error procedures to uncover
and repair service issues." The popularity of network assurance technology is
also being driven by a growing enterprise demand for network teams to do more
with less. "Efficiency is needed in order to manage the ever-expanding network
landscape," adds Gilby. New devices and equipment are constantly brought
online and added to networks. Yet enterprises don’t have unlimited IT budgets,
meaning that staffing levels often remain the same, even as workloads
increase.
How tomorrow’s ‘smart cities’ will think for themselves
In the smart cities of the future, technology will be built to respond to
human needs. Sustainability is the biggest problem facing cities – and by far
the biggest contributor is the automobile. Smart cities will enable the move
towards reducing traffic, and towards autonomous vehicles directed efficiently
through the streets. Deliveries which are not successful the first time are
one example. These are a key driver of congestion, as drivers have to return
to the same address repeatedly. In a cognitive city, location data that shows
when a customer is home can be shared anonymously with delivery companies –
with their consent – so that more deliveries arrive on the first attempt.
Smart parking will be another important way to reduce congestion and make the
streets more efficient. Edge computing nodes will sense empty parking spaces
and direct cars there in real-time. They will also be a key enabler for
autonomous driving, delivering more data points to autonomous systems in
cars.
Navigating Your Path to a Career in Cyber Security: Practical Steps and Insights
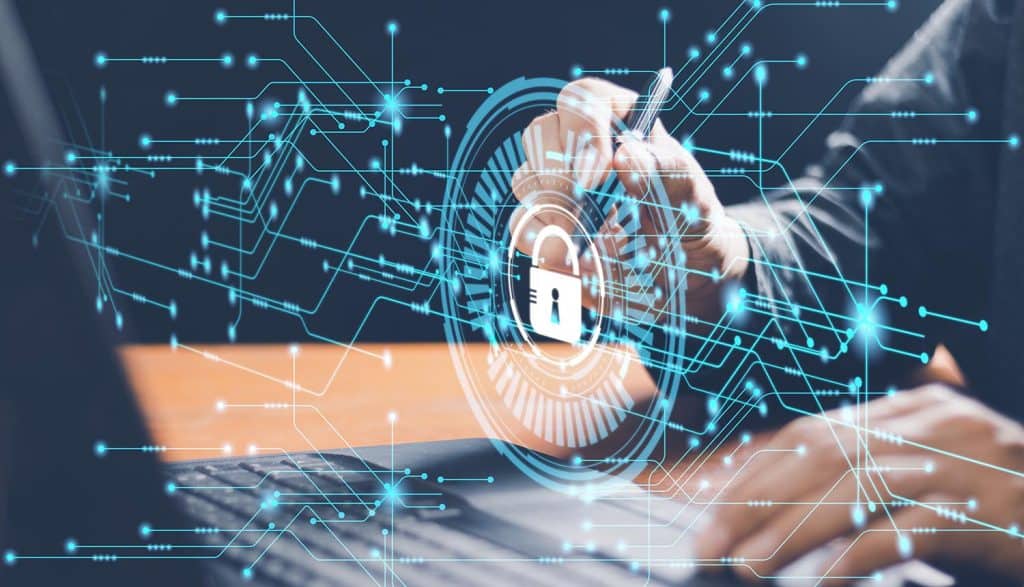
Practical experience is critical in the field of cyber security. Seek
opportunities to apply your knowledge and gain hands-on experience as often as
you can. I recommend looking for internships, part-time jobs, or volunteer
positions that allow you to work on real-world projects and develop practical
skills. I cannot stress how important it is to understand the fundamentals.
... Networking is essential for finding job opportunities in any field,
including cybersecurity. You should attend industry events and conferences
(there are plenty of free ones) and try to meet as many professionals already
working in the field as possible. Their insights will go a long way in your
journey to finding the right role. There are also many online communities and
forums you can join where cyber security experts gather to discuss trends,
share knowledge, and explore job opportunities. Networking will help you gain
insights, discover job openings, and even receive recommendations from
industry professionals.
NCSC warns over possible AI prompt injection attacks
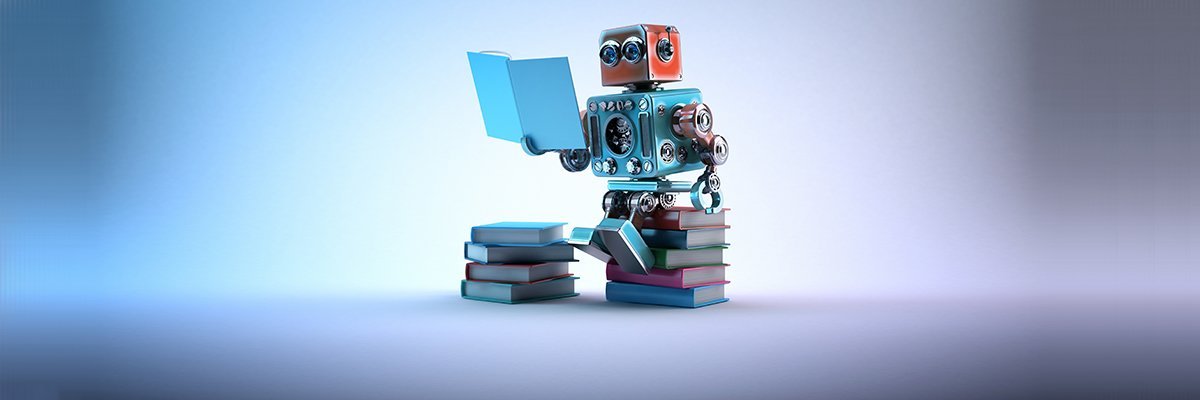
Complex as this may seem, some early developers of LLM-products have already
seen attempted prompt injection attacks against their applications, albeit
generally these have been either rather silly or basically harmless. Research
is continuing into prompt injection attacks, said the NCSC, but there are now
concerns that the problem may be something that is simply inherent to LLMs.
This said, some researchers are working on potential mitigations, and there
are some things that can be done to make prompt injection a tougher
proposition. Probably one of the most important steps developers can take is
to ensure they are architecting the system and its data flows so that they are
happy with the worst-case scenario of what the LLM-powered app is allowed to
do. “The emergence of LLMs is undoubtedly a very exciting time in technology.
This new idea has landed – almost completely unexpectedly – and a lot of
people and organisations (including the NCSC) want to explore and benefit from
it,” wrote the NCSC team.
Quote for the day:
"When you practice leadership, the
evidence of quality of your leadership, is known from the type of leaders
that emerge out of your leadership" -- Sujit Lalwani
No comments:
Post a Comment