How to tell if your cloud finops program is working
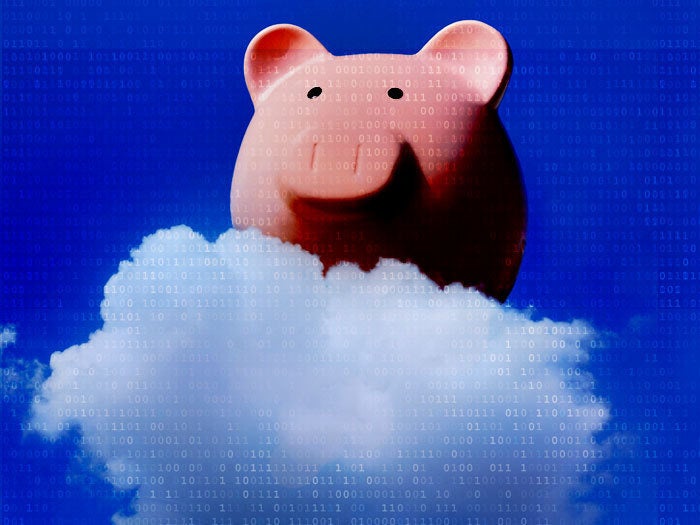
A successful finops program should ensure compliance with applicable financial
regulations and industry standards. These change across industries, but a few
industries, such as finance and health, are more constrained by rules than
others. A good finops program will help your company stay current with relevant
laws, rules, and regulations, such as GAAP (generally accepted accounting
principles) or IFRS (International Financial Reporting Standards). Regular
audits and reviews should be conducted to ensure that financial processes and
practices align with the required standards and laws. These are often overlooked
by cloud engineers and cloud architects building and deploying cloud-based
systems since most of them don’t have a clue about regulations and laws beyond
the basics. If done well, finops should take the stress off those groups and
automate much of what needs to be monitored regarding regulatory compliance. I
was early money on finops, and for good reason. We need to understand the value
of cloud computing right after deployment and monitor its value
continuously.
Why Data Science Teams Should Be Using Pair Programming

Based on what we learn about the data from EDA, we next try to summarize a
pattern we’ve observed, which is useful in delivering value for the story at
hand. In other words, we build or “train” a model that concisely and
sufficiently represents a useful and valuable pattern observed in the data.
Arguably, this part of the development cycle demands the most “science” from
data scientists as we continuously design, analyze and redesign a series of
scientific experiments. We iterate on a cycle of training and validating model
prototypes and make a selection as to which one to publish or deploy for
consumption. Pairing is essential to facilitating lean and productive
experimentation in model training and validation. With so many options of model
forms and algorithms available, balancing simplicity and sufficiency is
necessary to shorten development cycles, increase feedback loops and mitigate
overall risk in the product team. As a data scientist, I sometimes need to
resist the urge to use a sophisticated, stuffy algorithm when a simpler model
fits the bill.
Should IT Reinvent Technical Support for IoT?
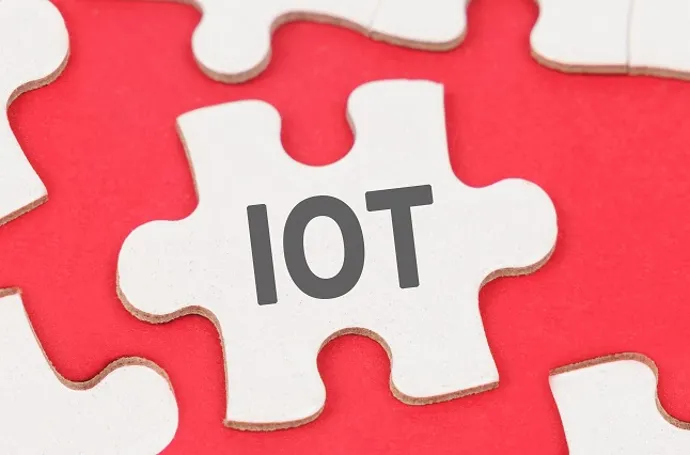
A first step is to advocate for IoT technology purchasing standards and to gain
the support of upper management. The goal should be for the company to not
purchase any IoT technology that fails to meet the company’s security,
reliability, and interoperability standards, which IT must define. None of this
can happen, of course, unless upper management supports it, so educating upper
management on the risks of non-compliant IoT, a job likely to fall to the CIO,
is the first thing that should be done. Next, IT should create a “no exceptions”
policy for IoT deployment that is rigorously followed by IT personnel. This
policy will make it a corporate security requirement to set all IoT equipment to
enterprise security standards before any IoT gets deployed. Finally, IT needs a
way to stretch its support and service capabilities at the edge without hiring
more support personnel, since budgets are tight. If something goes wrong at your
manufacturing plant in Detroit while technical issues arise at your San Diego,
Atlanta, and Singapore facilities, it will be a challenge to resolve all issues
simultaneously with equal force.
Why AI Forces Data Management to Up Its Game
With so much storage growth, organizations never reach the point where storage
is no longer a constant challenge. The combination of massive capacity growth
and democratized AI make it imperative to implement effective data management
from the edge to the cloud. A strong foundation for artificial intelligence
necessitates well-organized data stores and workflows. Many current AI projects
are faltering due to a lack of data availability and poor Data Management.
Skilled Data Management, then, has become a key factor in truly realizing the
potential of AI. But it also plays a vital role in containing storage costs,
hardening data security and cyber resiliency, verifying legal compliance and
enhancing customer experiences, decision-making, and even brand reputation. ...
Using metadata and global namespaces, the Data Management layer makes data
accessible, searchable, and retrievable on whatever storage platform or media it
may reside. It adds automation to facilitate tiering of data to long-term
storage as well as cleansing data and alerting on anomalous conditions.
Hybrid work is entering the 'trough of disillusionment'
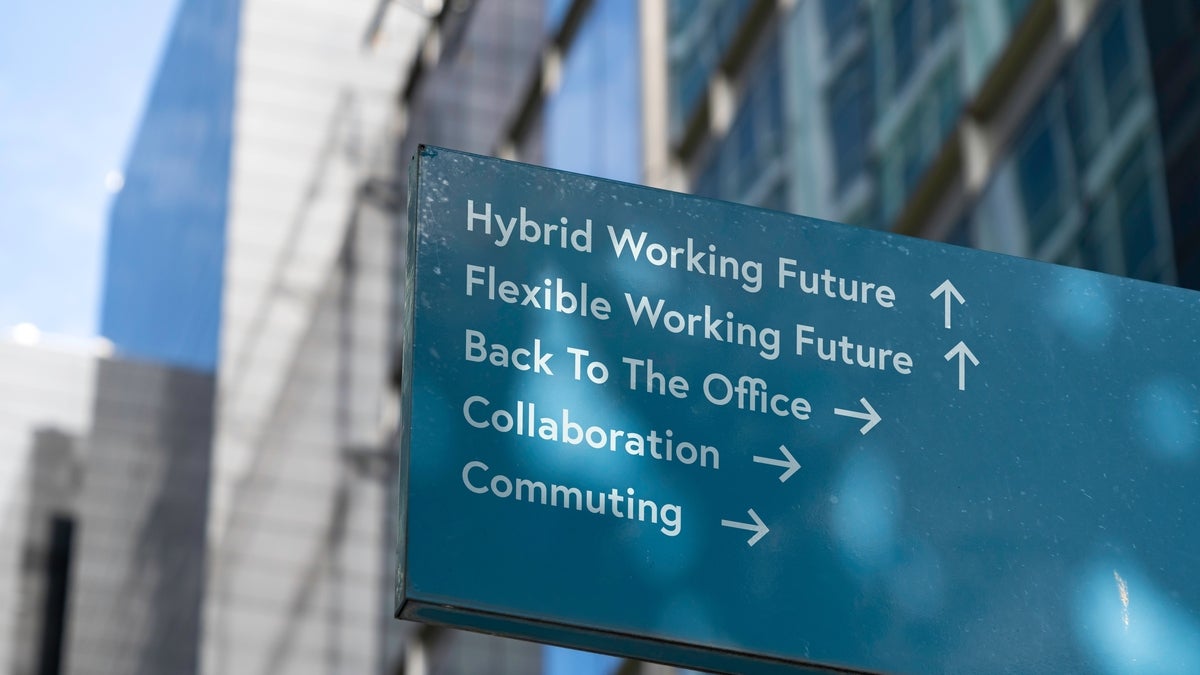
Even though remote and hybrid work practices are in the trough now, that doesn’t
mean they’ll stay there. Some early adopters eventually overcome the initial
hurdles and begin to see the benefits of innovation and best practices emerge.
Until then, the return-to-office edicts continue to roll out. ... Even with an
uptick in return-to-office mandates, office building occupancy continues to
remain below pre-pandemic levels. The average weekly occupancy rate for 10
metropolitan areas in the United States this week was below 50% (48.6%),
according to data tracked by workplace data company Kastle Systems. That
occupancy rate is actually down 0.6% from last week. Office occupancy rates
change substantially, depending on the day of the week. Tuesdays, Wednesdays and
Thursday are the most popular in-office days. Globally and in the US,
organizations have moved from ad hoc hybrid work policies, where employees could
pick their days in the office, to structured schedules.
Cisco: Hybrid work needs to get better
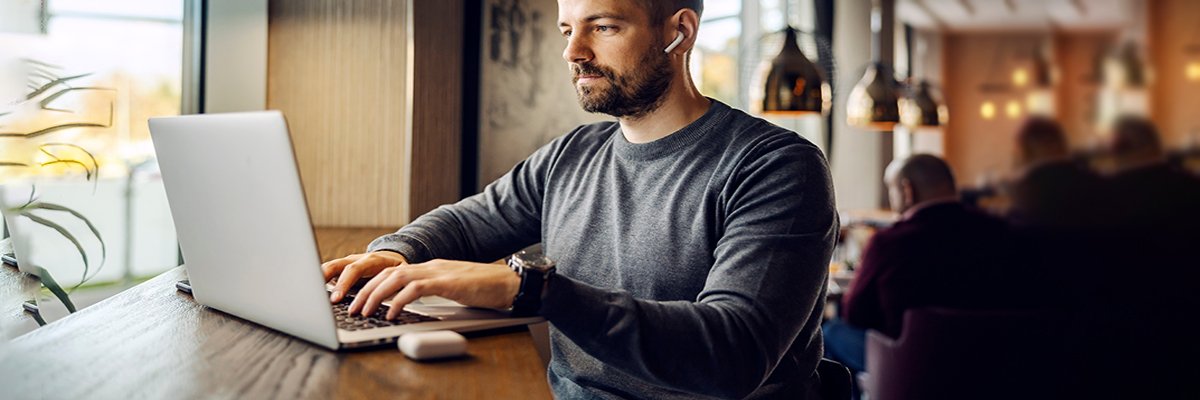
While organisations in APAC have been progressive in adopting hybrid work
arrangements, Patel cautioned them against making the mistake of mandating that
employees work in the office all the time. “It’s much better to create a magnet
than a mandate,” he said. “Give people a reason to come back to the office
because when they collaborate in the office, there’s going to be this X factor
that they don’t get when they are 100% remote.” Patel said adopting hybrid work
would also help organisations recruit the best talent from anywhere in the
world, enabling more people to participate equally in a global economy. “The
opportunity is very unevenly distributed right now, but human potential is
pretty evenly distributed, so it would be nice if anyone in a village in
Bangladesh can have the same economic opportunity as someone in Silicon Valley.
“Most of the time, the mindset is that you are distance-bound, so if you don’t
happen to be in the same geography, then you don’t have access to opportunity.
That’s a very archaic way of thinking and we need to think about this in a much
more progressive manner,” he said.
Rethinking data analytics as a digital-first driver at Dow

The first step in this journey involved bringing our D&A teams under one
roof in the first half of 2022. This team eventually became Enterprise D&A,
with team members based around the world. To develop the strategy, we held
discussions with external partners and interviewed Dow leaders to identify
trends important to business success. Then we looked at where those trends align
with key focus areas like customer engagement, accelerating innovation, market
growth, reliability, sustainability, and the employee experience. Our central
task was to translate our findings into a strategy that creates the most value
for our stakeholders: our customers, our employees, our shareholders, and our
communities. We determined we needed to move to a hub-and-spoke model. To make
this work and achieve our vision of transforming data into a competitive
advantage, we would need to build a strong culture of collaboration around
D&A and support it with talent development within our organization and
across the company.
Why data isn’t the answer to everything
What happens when you disagree with the AI? What are you then going to go and
do? If you’re always going to disagree with it and do what you wanted to do
anyway, then why bother bringing the AI in? Have you maybe mis-written your
requirements and what that AI system is going to go and do for you? A lot of
this is the foundational strategy on organisational design, people design,
decision making. As an executive leader, it’s really easy to stand up on stage
and say, ‘Here’s our 2050 vision or our 2030 vision.’ At the end of the day, an
executive doesn’t do much, they just create the environment for things to
happen. It’s frontline staff that make decisions. There are two reasons why you
wouldn’t make a decision: you don’t have the right data and context or you don’t
have the authority to make that decision. Typically, you only escalate a
decision when you don’t have the data and context. It’s your manager that has
more data and context, which enables that authority. So, with more data and
context, I can push more authority and autonomy down to the frontline to
actually go and drive transformation.
Whirlpool malware rips open old Barracuda wounds

The vulnerability, according to a CISA alert, was used to plant malware payloads
of Seapsy and Whirlpool backdoors on the compromised devices. While Seapsy is a
known, persistent, and passive Barracuda offender masquerading as a legitimate
Barracuda service "BarracudaMailService" that allows the threat actors to
execute arbitrary commands on the ESG appliance, Whirlpool backdooring is a new
offensive used by attackers who established a Transport Layer Security (TLS)
reverse shell to the Command-and-Control (C2) server. "CISA obtained four
malware samples -- including Seapsy and Whirlpool backdoors," the CISA alert
said. "The device was compromised by threat actors exploiting the Barracuda ESG
vulnerability." ... Whirlpool was identified as a 32-bit executable and linkable
format (ELF) that takes two arguments (C2 IP and port number) from a module to
establish a Transport Layer Security (TLS) reverse shell. A TLC reverse shell is
a method used in cyberattacks to establish a secure communication channel
between a compromised system and an attacker-controlled server.
How digital content security stays resilient amid evolving threats
AI technology advancements and the great opportunities it provides have also
motivated business leaders and consumers to reassess the underlying trust models
that have made the internet work for the past 40 years: every major advance in
computing tech has stimulated sympathetic updates in the computer security
industry, and this recent decisive move into a world powered by data, and
auto-generated data, is no different. Provenance will become a key component in
determining the trustworthiness of data. The changes though extend beyond
technology. Rather than continuing to use systems that were built to assume
trust and then verify, businesses and consumers will change and use verify then
trust systems which will also bring mutual accountability into all processes
where data is shared. Standards, open APIs and open-source software have proven
to be adaptable to changing technology previously and will continue prove
adaptable in the age of AI and significantly higher volumes of digital
content.
Quote for the day:
"He who wishes to be obeyed must know
how to command" -- Niccol_ Machiavelli
No comments:
Post a Comment