How to build employee trust as AI gains ground
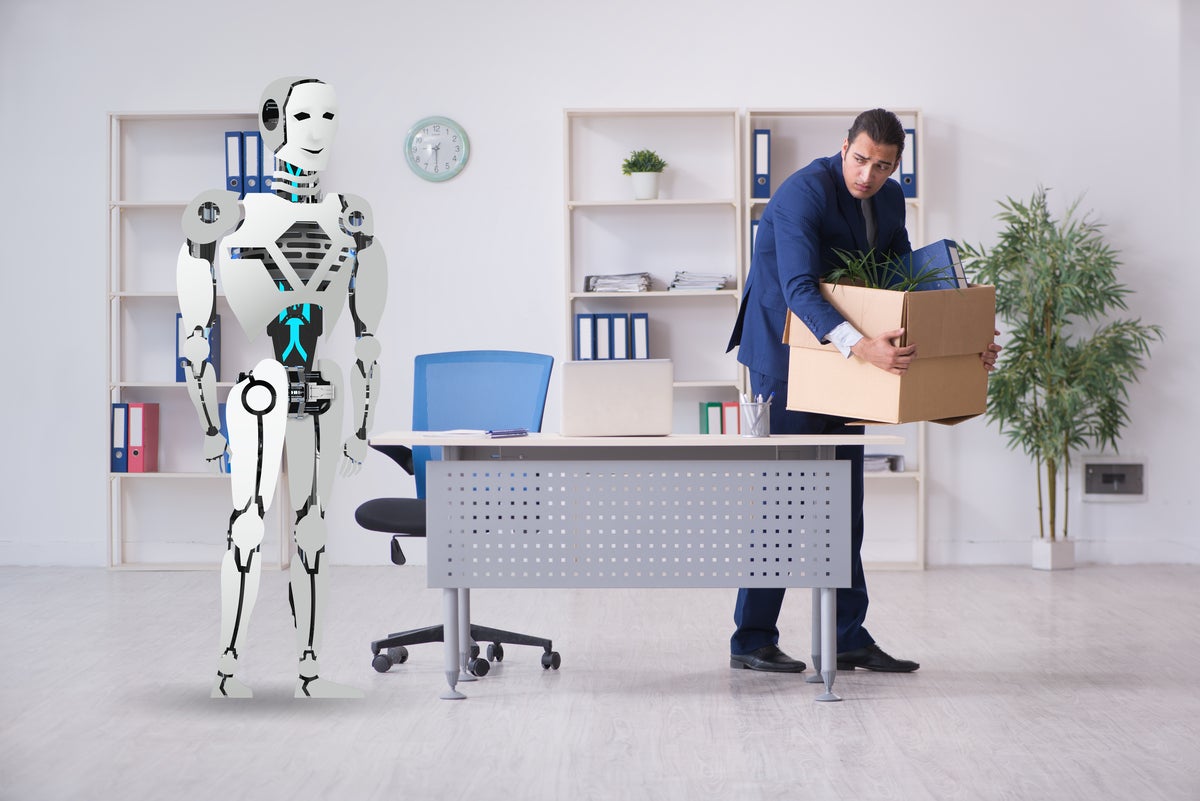
Most experts agree, however, that newer AI tools are less about replacing people
and more about eliminating mundane, manual, or number-crunching tasks that most
employees already hate. In fact, the technology will mostly help free up workers
to tackle more important tasks such as project management, data science research
and, perhaps most importantly, creative thinking and problem solving. "There is
no example today of an AI system that can perform data science totally
independent of people," said Erick Brethenoux, a distinguished vice president
analyst at research firm Gartner. A lot of the uncertainty and fear workers feel
about generative AI tools is based on ignorance, experts say. AI, in its many
forms, has been around for more than 50 years, but many people simply don’t
recognize it’s been beside them all this time. “People have always been afraid
of AI because the vision they have of it is science fiction; it’s a Hollywood
vision of it,” Brethenoux said. “There’s a lot of hype around it."
Red Hat rivals form Open Enterprise Linux Association
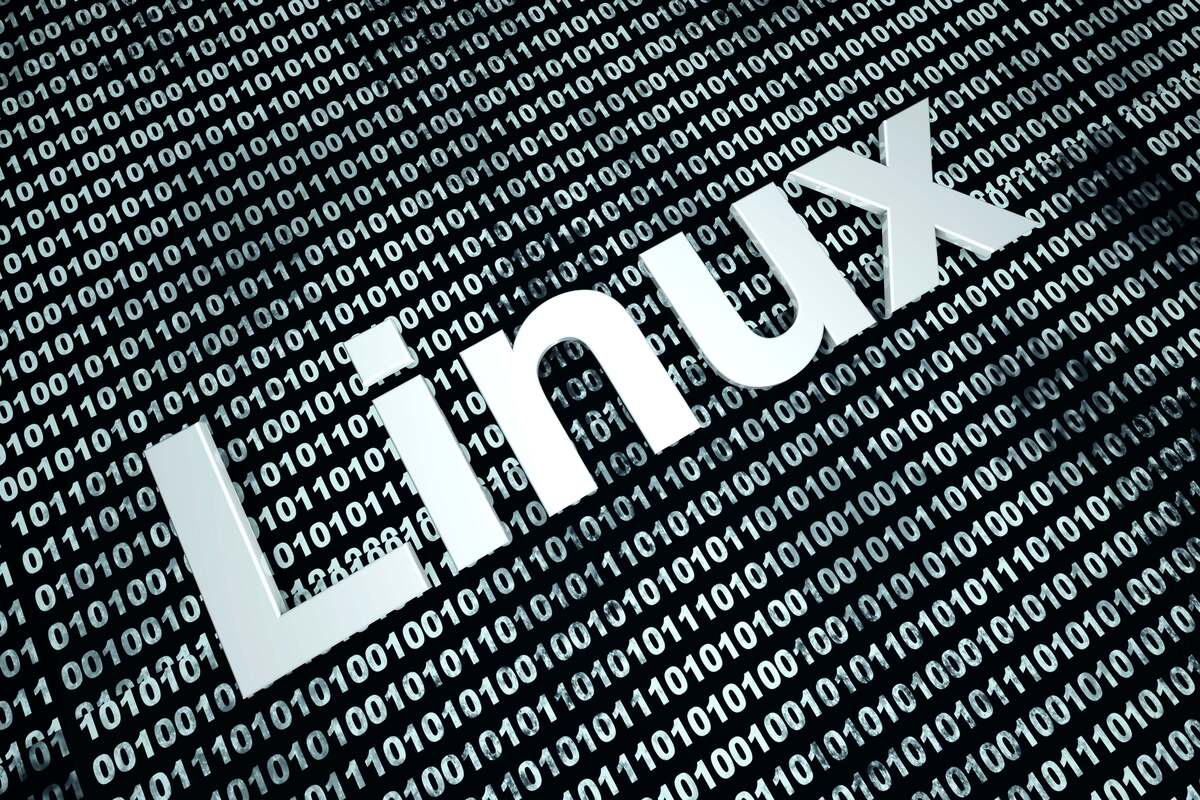
At the heart of the new organization is a disagreement over the way Red Hat,
long the dominant force in enterprise Linux, provides access to its source code.
For years, the company supported the development of a Red Hat Enterprise Linux
clone called CentOS, with the idea of providing a free alternative for testing
and development purposes, given that paid support would be unnecessary for that
purpose. However, increasingly, users began to implement CentOS instead of RHEL
in production environments as well, with other companies, including CIQ,
springing up to provide enterprise support. Accordingly, Red Hat stopped
supporting CentOS in its previous form two years ago, in favor of an alternative
called CentOS Stream. That, however, is an upstream distribution, meaning that
it’s updated much more frequently, making it less suitable for production work.
And earlier this summer, Red Hat made its source code less accessible,
restricting access to paying Red Hat customers and obscuring some details of the
way the code is put together to create the final distribution.
How FraudGPT presages the future of weaponized AI

FraudGPT signals the start of a new, more dangerous and democratized era of
weaponized generative AI tools and apps. The current iteration doesn’t reflect
the advanced tradecraft that nation-state attack teams and large-scale
operations like the North Korean Army’s elite Reconnaissance General Bureau’s
cyberwarfare arm, Department 121, are creating and using. But what FraudGPT and
the like lack in generative AI depth, they more than make up for in ability to
train the next generation of attackers. With its subscription model, in months
FraudGPT could have more users than the most advanced nation-state cyberattack
armies, including the likes of Department 121, which alone has approximately
6,800 cyberwarriors, according to the New York
Times — 1,700 hackers in seven different units and 5,100 technical support
personnel. While FraudGPT may not pose as imminent a threat as the larger, more
sophisticated nation-state groups, its accessibility to novice attackers will
translate into an exponential increase in intrusion and breach attempts,
starting with the softest targets, such as in education, healthcare and
manufacturing.
Application Rationalization: Is Complexity Avoidable?
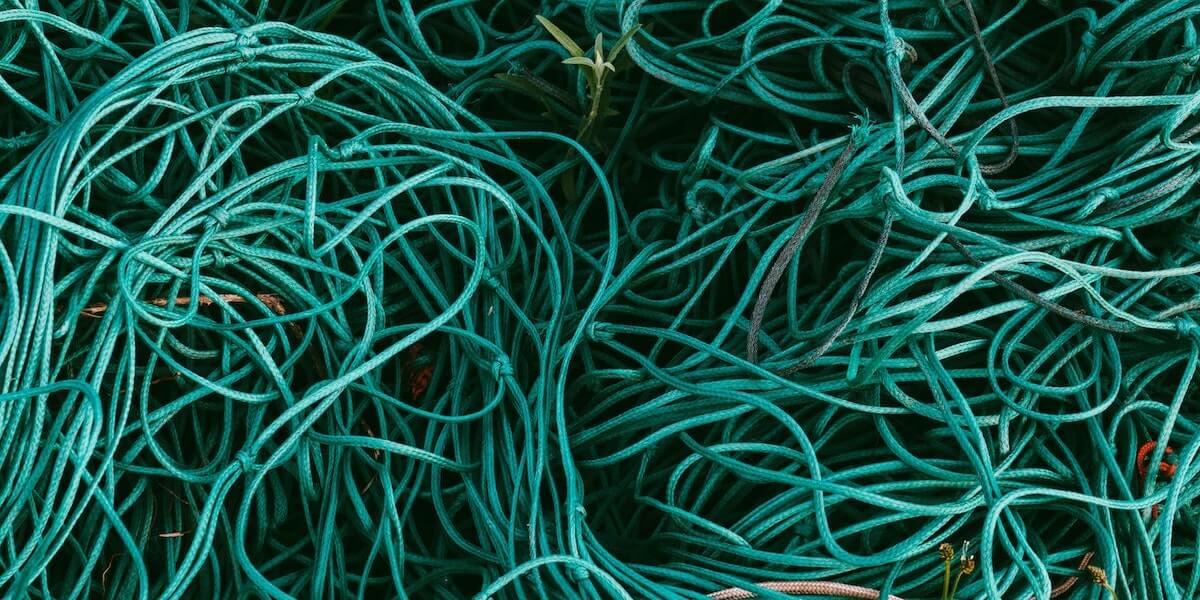
Removing the clutter from your application portfolio is its own reward.
Simplifying your software means: easier maintenance; greater agility; lower
training requirements; reduced costs; faster rationalization in
future. This is, indeed, all possible to achieve. With unlimited budget,
and a willingness to both make tough choices about stripping back applications
and be strict with your colleagues, you could of course remove all complexity
from your portfolio. The question remains, however: should you? Fully optimizing
your application portfolio is costly, time-consuming, and will likely cause a
lot of frustration for software users along the way. True application
rationalization involves a balancing act between technical debt and
optimization, meaning some complexity will likely need to be tolerated. If your
team communicates via Slack, for example, it would be easier to remove email and
Zoom licenses. However, if your external stakeholders don't use Slack Connect,
you could cripple your company's ability to function by doing so.
How to take action against AI bias
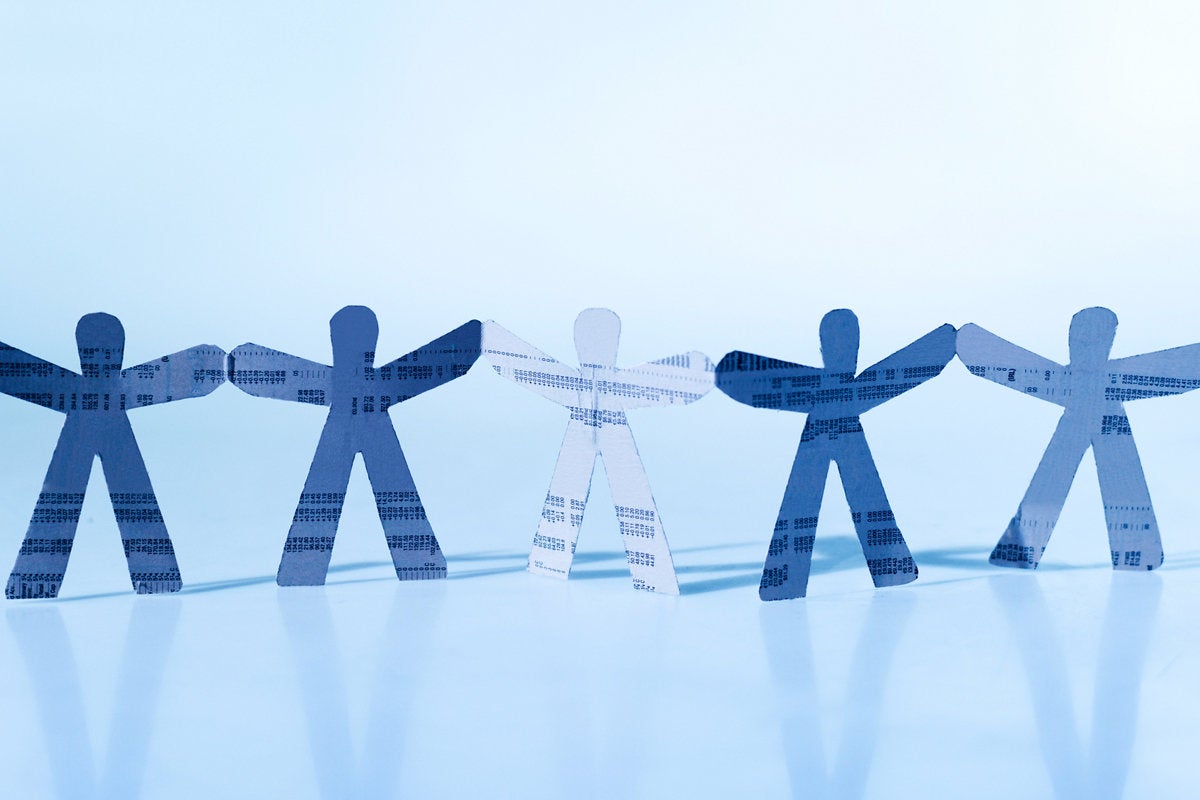
With AI adoption increasing rapidly, it’s critical that guardrails and new
processes be put in place. Such guidelines establish a process for developers,
data scientists, and anyone else involved in the AI production process to avoid
potential harm to businesses and their customers. One practice enterprises can
introduce before releasing any AI-enabled service is the red team versus blue
team exercise used in the security field. For AI, enterprises can pair a red
team and a blue team to expose bias and correct it before bringing a product to
market. It’s important to then make this process an ongoing effort to continue
to work against the inclusion of bias in data and algorithms. Organizations
should be committed to testing the data before deploying any model, and to
testing the model after it is deployed. Data scientists must acknowledge that
the scope of AI biases is vast and there can be unintended consequences, despite
their best intentions. Therefore, they must become greater experts in their
domain and understand their own limitations to help them become more responsible
in their data and algorithm curation.
3 Ways Enterprise Architects Can Bridge the Socio-Technical Gap
Software architecture is often a series of trade-offs. However, for people not
involved in the original decision, it is often no longer clear what the
trade-off was or how that trade-off led to the decision. One approach to
capturing these decisions is Architecture Decision Records (ADRs). Note that
ADRs are not some kind of technical rule, they are essentially a document. But
having such a document can be a useful communication device, as it creates a
history that allows people to keep track of trade-offs made in the past. The
code and architecture themselves can only communicate the current state, but not
how that current state came to be. Note that recording decisions doesn’t make
them permanent or immutable. ... Capturing the rationale behind architectural
decisions through methods like Architecture Decision Records ensures a clear
understanding of trade-offs made over time. Additionally, addressing
architecture incrementally, akin to code-level refinements, offers a practical
way to manage risk and avoid conflicting priorities.
Broken Promises of the Low-Code Approach

The reality is that many low-code solutions present a fundamental
misunderstanding of software development: They conflate the challenge of
understanding a programming language’s syntax with the challenge of designing
effective application logic. Programming languages are just tools; their syntax
is merely a means of expressing solutions. The true heart of software
development lies in problem-solving, in crafting algorithms, data structures and
interfaces that efficiently fulfill the application’s needs. By aiming to
simplify software development through a graphical user interface (GUI), low-code
solutions replace syntax without necessarily simplifying the fundamental
challenge of designing robust applications. This approach can introduce multiple
drawbacks while failing to alleviate the true complexities of software creation,
ultimately having a negative impact on your team’s ability to deliver real
value. ... Low-code solutions frequently grapple with limited customization,
often failing to meet specific, complex or unique business requirements. The
risk of vendor lock-in is another significant downside, potentially leaving
users high and dry if there are changes in pricing, feature offerings or if the
vendor closes shop.
Micro transformation: Driving big business benefit through quick IT wins

While it’s still early days to determine the success of the micro
transformation, the initial customer feedback has been encouraging, Aird says.
“There’s something intrinsically rewarding when you hear directly from customers
about how much they’re enjoying the new tool, how it’s adding value to their
purchasing experience, and how it makes the process of creating their own neon
signs easier and more fun and exciting.” This is critical because Custom Neon
operates in a “highly saturated e-commerce niche,’’ he adds, and micro
transformations such as upgrading the website tool “subtly, but surely redefine
the customer experience, contributing to our continued growth and
competitiveness.” This kind of micro transformation underscores the power of
agile methodology, enabling IT to identify bottlenecks, implement targeted
improvements, and quickly see the effects, Aird says. “Moreover, they allow us
to enhance our KPIs, notably in customer satisfaction and operational
efficiency.”
Cybersecurity hiring gap: Time to rethink who can contribute

Ford sees the "cybersecurity talent shortage" as misidentified, he refers to the
situation as an "experience shortage." As we all know, the only way to garner
experience is by doing. He opened doors to "overlooked" talent, with the
creation of their Cybersecurity Career Reboot Program. The program's key factor
probably broke every HR sorting tool, as they sought out individuals who had
been passed over because the "lack the experience required to land entry-level
jobs." ... They then used their Professional Rotation Experience Program (PREP),
which took recent grads and put them in "two-year rotational program that
includes global exposure to all our cybersecurity functions. PREP participants
gain experience with the foundations of cybersecurity through hands-on project
work, exposure to a variety of experiences, and innovative training and
development, rotating through the different teams within cybersecurity every six
months during the program." While the focus of homegrown talent programs is on
the new and eager employees, CISOs must also keep an eye on retaining and
improving the talent already in place.
Generative AI – What Are the Legal Issues?
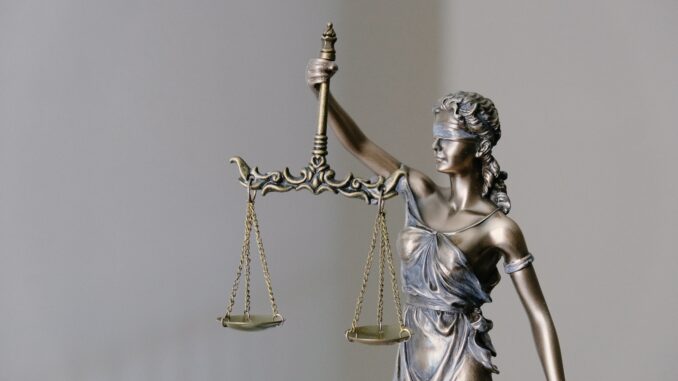
The pace of the development of AI far outstrips the legal, regulatory and
ethical frameworks which need to be put in place to ensure that the benefits
of AI are carefully considered. For anyone looking at adopting or developing
AI technologies, risk assessments should be conducted to identify and mitigate
the impact on individuals. ... Considering the dataset used to teach the
algorithm will potentially identify areas of risk. For example, an AI designed
to sift CVs and provide hiring recommendations might inherit any unconscious
hiring biases from the underlying dataset of ‘successful applicant’ and
‘unsuccessful applicant’ CVs. Not all algorithms are born equal and
consideration should be given to the sophistication and development of any
product before use given the potential impact on individuals. ... As Gen AI
can create new content, who will own the intellectual property in any new
work, media, image or music? There may be IP issues if the Gen AI creator did
not have sufficient rights to the information used in the training dataset and
any contract should clearly set out IP ownership where possible.
Quote for the day:
"It is the responsibility of
leadership to provide opportunity, and the responsibility of individuals to
contribute." -- William Pollard
No comments:
Post a Comment