The Important Difference Between Generative AI And AGI

Here are the key differences: Capability: Generative AI excels at replication
and is adept at producing content based on learned patterns and datasets. It can
generate impressive results within its specific scope but doesn't venture beyond
its programming. AGI, on the other hand, aims to be a powerhouse of innovation,
capable of understanding and creatively solving problems across various fields,
much like a human would. Understanding: Generative AI operates without any real
comprehension of its output; it uses statistical models and algorithms to
predict and generate results based on previous data. AGI, by contrast, would
need to develop a genuine understanding of the world around it, making
connections and having insights that are currently beyond the reach of any AI
system. Application: Today, Generative AI is widely used across industries to
enhance human productivity and foster creativity, performing tasks ranging from
simple data processing to complex content creation. AGI, however, remains a
conceptual goal.
Top strategies for ensuring data center reliability and uptime in 2024
Robust security measures constitute another cornerstone of data center
reliability, safeguarding against both cyber threats and physical intrusions.
Cybersecurity protocols should encompass multifaceted defense strategies,
including perimeter security, network segmentation, encryption, and intrusion
detection systems. Regular vulnerability assessments and penetration testing
help identify and remediate potential weaknesses before they can be exploited by
malicious actors. Physical security measures, such as access controls,
surveillance systems, and environmental monitoring, bolster protection against
unauthorized access and environmental hazards. Additionally, robust disaster
recovery and business continuity plans should be in place to ensure swift
recovery in the event of a security breach or natural disaster. Automation and
orchestration technologies offer further avenues for enhancing data center
reliability by streamlining operations and reducing the risk of human errors.
Reassessing Agile Software Development: Is It Dead or Can It Be Revived?

Why, exactly, do so many folks seem to dislike — and in some cases loathe —
agile software development? There's no simple answer, but common themes
include:Lack of specificity: A lot of complaints about agile emphasize that the
concept is too high-level. As a result, actually implementing agile practices
can be confusing because it's rarely clear exactly how to put agile into
practice. Plus, the practices tend to vary significantly from one organization
to another. Unrealistic expectations: Some agile critics suggest that the
concept leads to unrealistic expectations — particularly from managers who think
that as long as a development team embraces agile, it should be able to release
features very quickly. In reality, even the best-planned agile practices can't
guarantee that software projects will always stick to intended timelines. Misuse
of the term "agile": In some cases, developers complain that team leads or
managers slap the "agile" label on software projects even though few practices
actually align with the agile concept. In other words, the term has ended up
being used very broadly, in ways that make it hard to define what even counts as
agile.
An Architect’s Competing Narratives
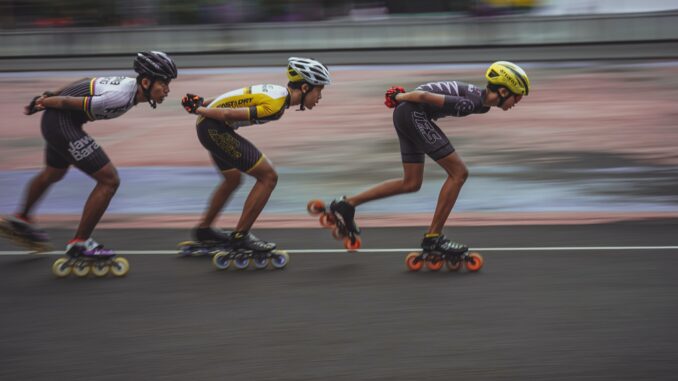
The biggest objection to basing architecture on the traditional EA narrative is
that it is not transformation focused. The ivory-tower analogy has stuck
permanently in this space. The other architects in a practice are often quite
vocal in their difficulty with top-down control concepts that are necessary in
the enterprise architect mindset. There are not enough of them to cover all the
places they need to be. Their skills atrophy and theirfore they lose the ability
to critic others work. They often feel connected to their scope as if it is
seniority or authority. That connection with ‘Enterprise’ or ‘Domain’ sometimes
causes conflict especially if they have not personally delivered a solution or
outcome in a long time. In addition the scope based titles seem to interact
poorly with other leadership roles both in IT and business as there is not clear
ownership. Another type of challenge has emerged in the last ten to fifteen
years. You can think of this as the ‘pure EA’ or ‘whole EA’ challenge.
Effectively a group of practitioners and writers are regularly pointing to
technology skilled EAs and calling them IT EAs and using that to minimize the
value of those practitioners.
Exploring generative AI's impact on ethics, data privacy and collaboration

Implementing GenAI presents organizations with multifaceted challenges,
particularly data privacy and security. The accuracy of GenAI outputs and the
responsibility of organizations and employees to ensure that the outputs are
representative and accurate – are also significant challenges. Governance,
transparency, and the presence of unexpected biases are additional hurdles.
Concerns range from accidentally breaching intellectual property and copyright
by sharing data in an unlicensed or unvetted tool to the potential for privacy
breaches and cybersecurity threats that GenAI can exacerbate. This data may
contain private information about people, sensitive business use cases, or
health care data. Unauthorized access to or inappropriate disclosure of these
types of data can cause harm to individuals or organizations. While privacy
and security were previously associated with intellectual property (IP) and
cybersecurity, the definition and scope have expanded in recent years.to
encompass data access management, data localization and the rights of data
subjects.
AI chip shortages continue, but there may be an end in sight

The breakneck pace of AI adoption over the past two years has strained the
industry’s ability to supply the special high-performance chips needed to run
the process-intensive operations of genAI and AI in general. Most of the focus
on processor shortages has been on the exploding demand for Nvidia GPUs and
alternatives from various chip designers such as AMD, Intel, and the
hyperscale datacenter operators, according to Benjamin Lee ... Nvidia is
tackling the GPU supply shortage by increasing its CoWoS and HBM production
capacities, according to TrendForce. “This proactive approach is expected to
cut the current average delivery time of 40 weeks in half by the second
quarter [of 2024], as new capacities start to come online,” TrendForce report
said in its report. ... On the software side of the equation, LLM creators are
also developing smaller models tailored for specific tasks; they require fewer
processing resources and rely on local, proprietary data — unlike the massive,
amorphous algorithms that boast hundreds of billions or even more than a
trillion parameters.
CDOs’ biggest problem? Getting colleagues to understand their role

One reason the role may be misunderstood, the report says, is because it’s
relatively new. The CDO position first gained momentum around 2008, to ensure
data quality and transparency to comply with regulations following the housing
credit crisis of that era. The CDO role also lacks a standard list of
responsibilities, potentially adding to the confusion, note the report’s
authors Thomas H. Davenport, Randy Bean, and Richard Wang. One possible
definition of the CDO is the organization’s leader responsible for data
governance and use, including data analysis, mining, and processing. In many
cases, CDOs focus on business objectives, but in other cases, they have equal
business and technology remits, according to the authors. ... “The role runs
the gamut from being a very traditional IT-focused role that is oriented to
the management of data, to one that resides in the business and is focused on
the application of data to create value,” he says. “I anticipate that we will
see the role solidify over the coming years, with a bias to value
creation.”
Open Source Is at a Crossroads

Struggles in open source communities undoubtedly stem from the greater economic
climate. The start of the current decade saw a low interest rate environment,
which Lorenc credits as ushering in a massive boom in the number of open source
companies and projects. But now, we are experiencing significant realignment.
“Time and money are even more scarce, making it harder for contributors or
companies to allocate resources,” he said. “Many, but not all, open source
businesses are at a crossroads,” said Fermyon CEO Matt Butcher. For ages, the
theory was that you built an open source tool, established a community and then
figured out how to monetize it. But now, the companies in that final stage are
under immense pressure to increase profit, he said. “For some, that means
abandoning the open source model.” A lack of resources to justify open source
may also stem from a “plethora of riches” problem, explains Chris Aniszczyk, the
chief technology officer of CNCF. With so many projects vying for attention,
it’s easier than ever for innovative projects to lose out on the resources they
require.
Can NIS2 and DORA improve firms’ cybersecurity
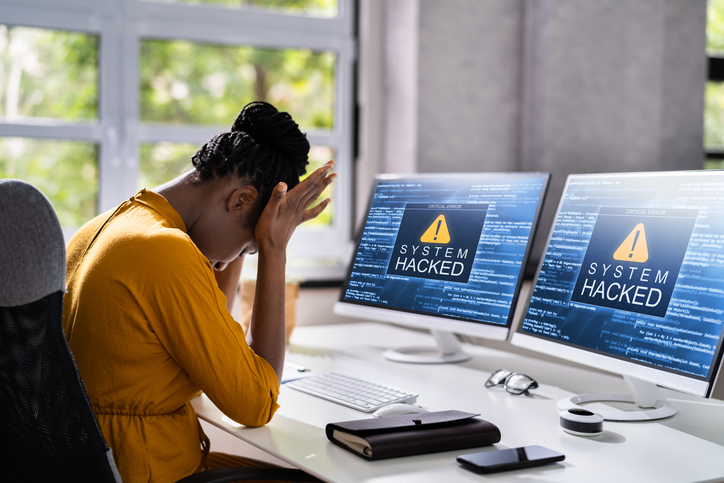
One of the biggest issues with both NIS2 and DORA is the fact that they overly
focus on promoting security and resilience without providing end-users with a
blueprint for success. In paying too much attention to the outcomes that
enterprises should be working towards, they fail to offer clear step-by-step
guidance on the actions that businesses should take to reach those end goals.
This is in part due to a recognition that every business is different. With each
individual organisation having a better understanding of its own unique digital
footprints, the belief is that it makes more sense for enterprises to interpret
the guidelines in a way that makes sense for them. This is very much the case
with DORA, where enterprises shoulder the responsibility of not only defining
what qualifies as a business-critical service but also pinpointing its
interconnected dependencies. Unfortunately, allowing regulations to remain open
to interpretation in this manner can lead to confusion and inconsistencies,
creating complexity to the environment for both organisations and auditors.
Trusted Data Access and Sharing — Why Automation Is the Key to Achieving Value from Data Democratization
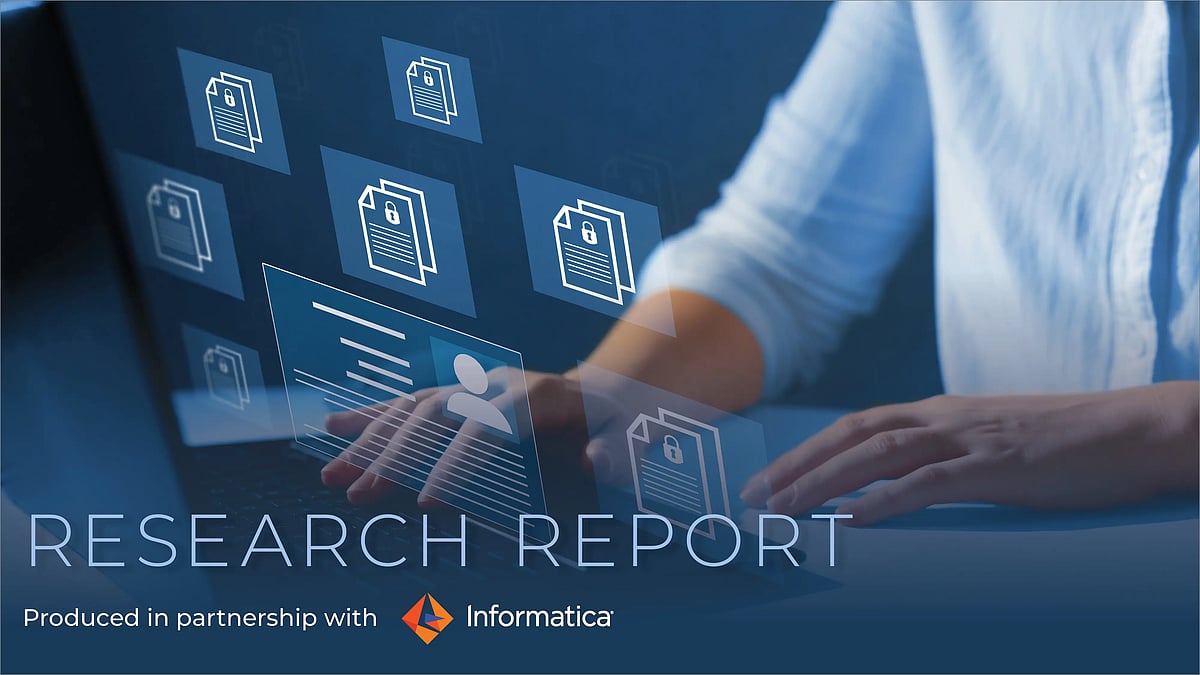
As organizations endeavor to democratize data access and empower individuals
across the enterprise, the last mile of data delivery emerges as the critical
phase in the journey. This final stretch represents an opportunity to share data
and data products responsibly, helping to ensure that insights reach the right
users when needed and with appropriate context. However, achieving
individualized data access poses significant challenges and concerns that need
addressing to realize the potential of data democratization. The last mile of
data delivery is where organizations must contend with a wide range of
variables, including specific user requirements, use cases, rules, and
contextual nuances that inform policies for granting conditional entitlements
to access the data responsibly. ... The controls on sharing data need
to be as granular as possible about who is requesting access and under what
conditions to justify the data types for provisioning. However, the traditional
manual approach to last-mile data delivery that requires negotiating with data
consumers to understand their needs is a significant roadblock to
democratization.
Quote for the day:
"Leadership is a matter of having
people look at you and gain confidence, seeing how you react. If you're in
control, they're in control." -- Tom Landry
No comments:
Post a Comment