Is it too soon for AI in the education landscape?
Even if schools did have enough money, not only is their choice of software limited, but many heads and teachers are neither trained nor qualified to either select or use even basic educational technology, let alone AI tools. There is also a widespread fear of the unknown, part of which includes the much-discussed issue of jobs being automated out. Another major concern relates to ethics, believes Elena Sinel, who is a member of the All Parliamentary Group on AI and also founder of Acorn Aspirations and Teens in AI, which provide various forums for young people to learn tech skills. A key challenge in this context is in ensuring AI does not end up doing “more harm than good”, she says. “So it’s about looking at who is accountable if things go wrong – for example, what happens if there’s a data leak and who is ultimately in charge of the data? Or what happens if AI doesn’t assess students fairly or accurately in exams, for instance?” says Sinel. Such questions also fit into a wider debate around whether schools are currently set up to provide young people with the skills required for the workplace of the future, or whether fundamental change is required.
Prepare Now for Next-Generation Cyber Threats
Impacts will be felt across a range of industries. Malicious attacks may result in automated vehicles changing direction unexpectedly, high-frequency trading applications making poor financial decisions, and airport facial recognition software failing to recognize terrorists. Where machine learning systems are compromised, organizations will face significant financial, regulatory, and reputational damage, and lives will be put at risk. Nation states, terrorists, hacking groups, hacktivists, and even rogue competitors will turn their attention to manipulating machine learning systems that underpin products and services. Attacks that are undetectable by humans will target the integrity of information. Widespread chaos will ensue for those dependent on services powered primarily by machine learning. Companies should assess their offerings and dependency on machine learning systems before attackers exploit related vulnerabilities.
Rethinking reskilling: How to find key hidden talent within your organization
To overcome the talent gap and foster adaptive workforces able to keep up with ongoing transformations in tech and industry, there is a clear need to shift from traditional L&D techniques like seminars and online training sessions, to leveraging existing experts within the organization so that we harness the collective intelligence of individuals and teams. These are employees who often already have the skills and knowledge that others need and follow the development of those fields closely. As a result, they can curate and contextualize that knowledge better than any external teacher, hence making it easier to for others to absorb it. Companies also need to tap what can be a hidden resource of knowledge, identifying “invisible” go-to resources; i.e., knowledgeable employees who may be currently unrecognized or perhaps are not even hierarchically high in the company structure, but seem to be go-to people for large networks of employees. Organizations can consider practices similar to Genpact’s Genome reskilling initiative, which uses advanced human network analysis techniques to identify these invaluable knowledge leaders outside of the usual suspects of widely known company subject matter experts.
A Framework for High-Value Big Data
More and more companies are achieving the monetization of data by improving efficiencies, developing new products, growing new markets, and by reducing risks. Saxena talked about Netflix's original series like Orange is the New Black that are a direct result of data-driven innovation. She elaborated on the big data framework elements. Organization maturity is about hard assets in an organization, like its strategy, data, quality etc. Every organization should have a business strategy, as well as a data strategy. The internal competencies are about people, and focus on soft assets like leadership, engagement, and adaptability. Health care organizations in the field of precision health like Geisinger are taking advantage of big data and genomic sequencing to transform healthcare practices, in order to prevent people from becoming sick and to treat people more as individuals (customers), rather than just patients. Data governance initiatives should include aspects of data integration, quality, accessibility and data security.
Leading DevOps program Chef goes all in with open source
What does that mean for Chef's customers? Jacob said, "Chef Software produces only open-source software projects, in the commons. It distributes that software as an enterprise product. For current Chef Software customers, nothing changes. For enterprise users of Chef products who are not customers, they can decide to either pay for Chef's distribution, or they can make or consume an alternative." Going deeper in the new Chef FAQ, Chef stated: "We will begin to attach commercial license terms to our software distribution (binaries) with the next major release." So, if you download and compile the code yourself, you're welcome to use it. But, if you download the binaries, you'll must pay for them. If that sounds familiar, it should. It's a variation of how Red Hat and SUSE, for example, release their enterprise Linux distributions. . . . For existing commercial customers there will be no immediate changes until their next renewal when they will get licensed onto new SKU's representing the same core products."
Bitcoin, BlackRock And The Rise Of Alternatives
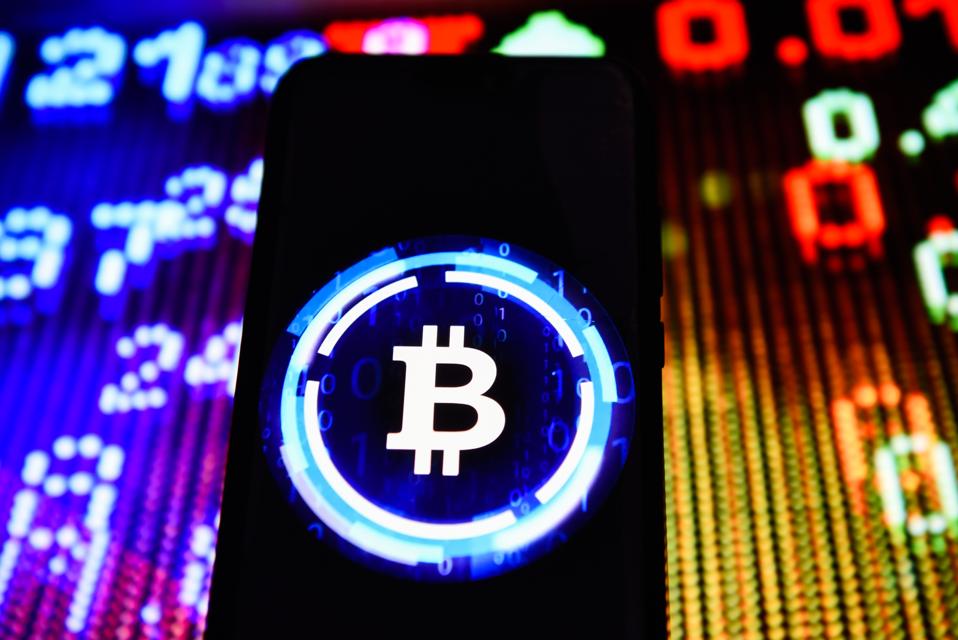
As an alternative asset, the appeal of crypto is that its movements are uncorrelated with the rest of the market, says Mark Yusko, CEO of Morgan Creek Capital Management, which oversees $1.5 billion in assets, including a $40 million blockchain-focused VC fund. “Stocks or bonds derive their value from factors like GDP growth, profitability and interest rates. A cryptocurrency network derives its value from usage growth, adoption, regulation and technology. All of those things are uncorrelated with traditional measures of stocks and bonds.” ... Yusko claims inbound interest from institutional investors is growing. This week, he’s meeting with a California municipal pension fund. He adds that more institutional-investor conferences are including talks on cryptocurrencies. Teddy Fusaro, chief operating officer of Bitwise, a San Francisco digital asset manager and creator of the first crypto index fund, says institutional investors are showing increasing sophistication. “A year ago,” he says, “the conversation might have been, ‘How do we know bitcoin is going to survive?’ Or ‘Who is the CEO of bitcoin?’
6 Essential Skills Cybersecurity Pros Need to Develop in 2019
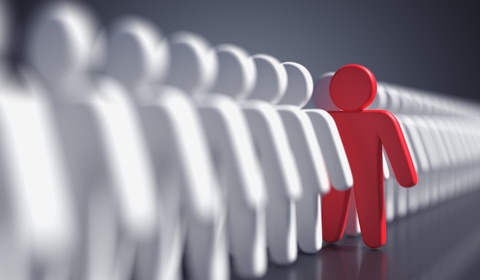
On their face, these stats may engender a bit of complacency from cybersecurity professionals. It would only be natural to figure that anybody with a pulse and some security experience has got it made. But here's the rub. Many disruptive forces are at play that are set to drastically change the way security duties are carried out in the coming years. New security automation platforms, new architectures, and complex hybrid cloud implementations require major shifts in bread-and-butter security technical knowledge. Not only is security technology changing rapidly, but so are many of the fundamental roles held by cybersecurity professionals. Tons of emerging technologies and pervasive use of the Internet of Things are touching every aspect of business operating models, and software delivery is becoming more agile and embedded into lines of business. As a result, security pros are tasked to take positions requiring more consultative leadership and more enablement of democratized security across the organization.
What Is a Scaleup Company and How Is It Different from a Startup?

From a venture capital and entrepreneurial perspective, a scaleup company is considered to be in a later growth phase, after successfully maneuvering through the period of being a startup and having established a sustainable business model with a positive outlook on organizational growth and improvements of the profitability. For additional information on this aspect, you can also have a look at “How to Upscale Like a Boss“. It does not take much to “found” a startup company. Anybody with an interesting idea can register a company which then could be considered to be a startup. It then either fails or becomes successful after a lot of hard work. The question is more on… When does a company stop being a startup? As soon as the startup company has finished an MVP (minimum viable product) and has a stable monthly income, which is hopefully more than the company’s expenses, the organization ceases to be a startup. And that’s a good thing. Being a startup company is nothing good and nothing aspirational. To read more about the exit of this phase, you can also read our article “When Does a Company Stop Being a Startup?“.
Joining Human And Artificial Intelligence

Although the aim of AI is to imitate HI to the point where both are indistinguishable, AI and HI are fundamentally different. Human intelligence learns via the senses and past experiences. They are also emotionally intelligent, which is something that AI is yet to crack. But AI is analytical and logical in a way that humans aren’t, and with this, it is capable of formulating and processing in ways that humans can’t. AI can take huge datasets and whittle them down to snippets of relevant information quickly. It can complete tasks in minutes as opposed to days, and it can identify data discrepancies that humans would never spot. Artificial and human intelligence is a match made in business heaven. The AI-HI model is already in practice across a number of sectors. In healthcare, clinical decisions are aided by artificially intelligent systems that search through historical data at a pace that human professionals never could. But, that said, getting a diagnosis direct from AI would be a very different experience to getting it from a doctor or nurse. Naturally you need both – AI augmenting human intelligence can lead to increased efficiency and accuracy.
How the data mining of failure could teach us the secrets of success
Since learning should reduce the number of attempts required before achieving success, it should lead to a narrower distribution of failure streaks than the exponential form predicted by the chance model. But to the surprise of Yin and co, failure streaks do not follow this pattern either. In fact, they have a much fatter-tailed distribution. “These observations demonstrate that neither chance nor learning alone can explain the empirical patterns underlying failures,” the researchers say. So what other factors are important? To find out, Yin and co modeled the way people learn from experience and how this influences their next attempt. In particular, they modeled whether people take into account all their previous experiences or just some of them. The resulting model considers a complete range of learning—from agents who take all their past experience into account to those who do not take any of their past experience into account, and everything in between. The team say the model predicts a phase change in the behavior that matches the empirical data.
Quote for the day:
"Coaching isn't an addition to a leader's job, it's an integral part of it." -- George S. Odiorne
No comments:
Post a Comment