HPE bets on hybrid IT to combat public cloud
In a note covering the meeting, financial analyst Berenberg wrote that HPE’s strategy remains focused on three key areas. The first is simplifying hybrid IT using its datacentre technologies, systems software, and private and public cloud partnerships. The second area of focus is to support the so-called intelligent edge. This encompasses its offerings from Aruba in campus and branch networking, and the industrial internet of things (IoT) with products such as Edgeline and the Universal IoT software platform, said Berenberg. The third area of focus is HPE’s advisory, professional and operational services, which Berenberg said will include consumption-based pricing. The analyst said HPE’s decision to stop selling custom-designed commodity servers to the tier-one service providers, while continuing to sell them higher-margin products, was a risky business strategy.
GDPR Requirements for US Companies

An organization may decide not to do business with EU citizens to avoid having to comply with GDPR, but even that decision must be implemented correctly. If you maintain a website that uses cookies, and it can be accessed by EU citizens, GDPR applies. GDPR also applies to organizations of all sizes. It doesn’t matter if you are a small one-person practice or a large organization with thousands of employees. If you collect or process data on EU citizens, GDPR compliance is not optional. GDPR replaces the EU Data Protection Act of 1998, which placed responsibility only on the data controller, not processors of data. If you processed data for another company (the controller) it would be that company that had to comply with past regulations. GDPR applies to both processors and controllers – Both parties are now responsible for protecting the privacy rights of EU citizens.
Data: Lifeblood of the Internet of Things
As IoT matures and we gain more confidence in the technology, we will increasingly use it on more critical applications – like self-driving cars— where errors could lead to serious injury or disruption. This requires us to ensure critical and hypercritical data is prioritised – and in turn it will drive an enormous shift in how systems capture, manage, store, secure, and process information. Analytics, for instance, will increasingly need to happen in real-time and superior analytics will become a competitive advantage. IDC estimates that by 2025, about 20 percent of all data will be critical—meaning necessary for the continuity of daily life--and nearly 10 percent of that will be hypercritical, or directly impacting the health and wellbeing of users. Not all data is equally important, but the amount of hypercritical data generated by IoT is accelerating dramatically.
GetStream.io: Why We Switched from Python to Go

Go is extremely fast. The performance is similar to that of Java or C++. For our use case, Go is typically 30 times faster than Python. ... For many applications, the programming language is simply the glue between the app and the database. The performance of the language itself usually doesn’t matter much. Stream, however, is an API provider powering the feed infrastructure for 500 companies and more than 200 million end users. We’ve been optimizing Cassandra, PostgreSQL, Redis, etc. for years, but eventually, you reach the limits of the language you’re using. Python is a great language but its performance is pretty sluggish for use cases such as serialization / deserialization, ranking and aggregation. We frequently ran into performance issues where Cassandra would take 1ms to retrieve the data and Python would spend the next 10ms turning it into objects.
Survey shows most workers misunderstand cybersecurity
The report concluded by advocating employee education programs in order to create a culture of cybersecurity. However, keeping users informed about the current threat landscape isn't the difficult part of establishing appropriate cybersecurity. That award goes to keeping them focused on their behavior so they can understand how to make the right choices. As a system administrator I'm a big proponent of automation wherever possible and this includes patching/securing systems and devices. Proper automation can apply updates and restart systems automatically without establishing a reliance on the end users, run in the background in the form of anti-spam filters and antimalware software, or enforce policies intended to protect devices such as by mandating passwords or utilizing encryption.
How AI can help you stay ahead of cybersecurity threats
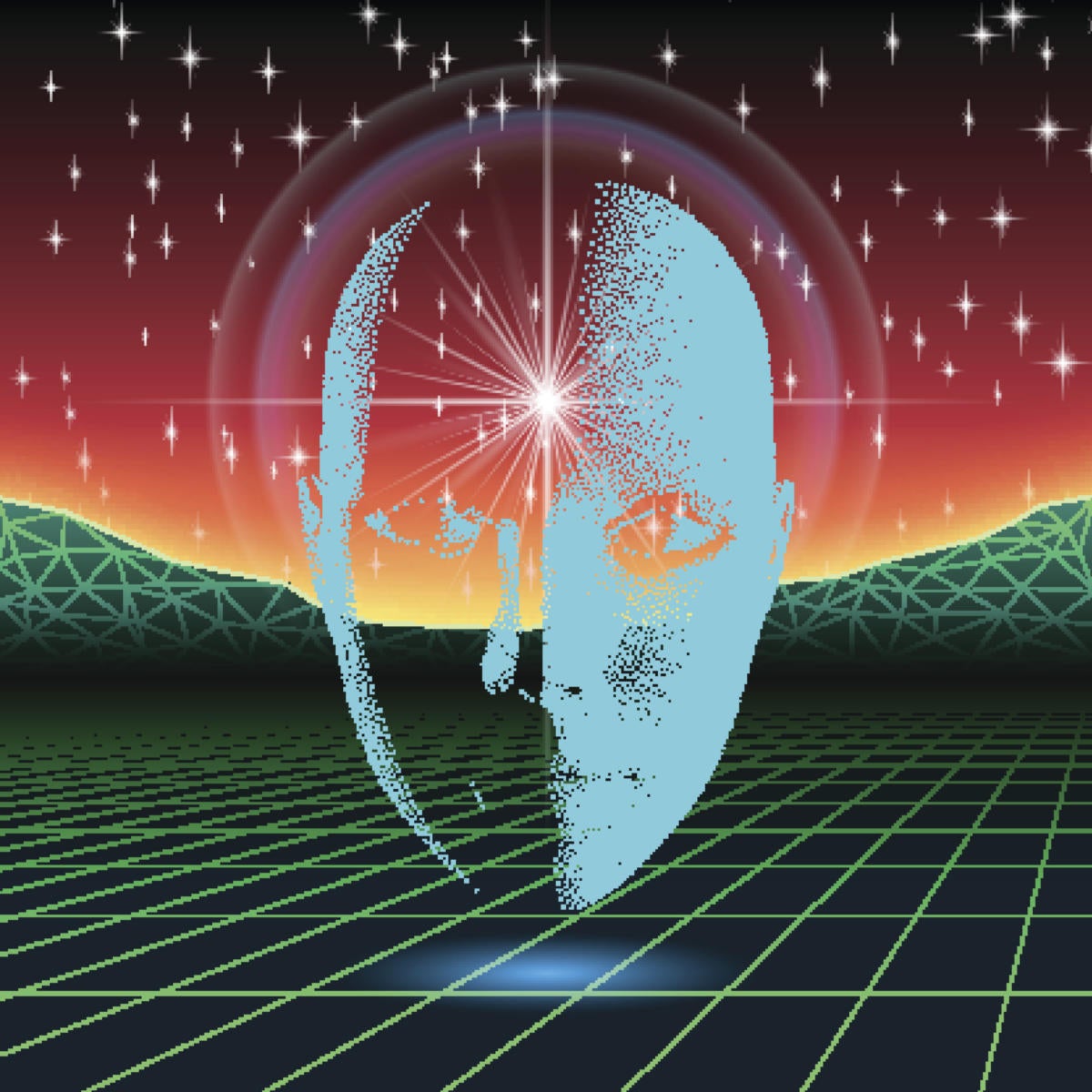
Barclays Africa is beginning to use AI and machine learning to both detect cybersecurity threats and respond to them. “There are powerful tools available, but one must know how to incorporate them into the broader cybersecurity strategy,” says Kirsten Davies, group CSO at Barclays Africa. ... AI and machine learning also lets her deploy her people for the most valuable human-led tasks. “There is an enormous shortage of the critical skills that we need globally,” she says. “We've been aware of that coming for quite some time, and boy, is it ever upon us right now. We cannot continue to do things in a manual way.” ... San Jose-based engineering services company Cadence Design Systems, Inc., continually monitors threats to defend its intellectual property. Between 250 and 500 gigabits of security-related data flows in daily from more than 30,000 endpoint devices and 8,200 users
Data Science, IT And Your Digital Transformation
As data science becomes more commonplace in enterprise environments, businesses are gaining a better understanding of how to deliver the experiences customers crave. Even so, when my company, DataScience.com, commissioned Forrester to survey more than 200 businesses last year, it found that only 22% were leveraging big data well enough to get ahead of their competition. Standing in the way of these companies on the road to a digital-first approach are challenges inherent to large-scale data management, governance and access. If handled incorrectly, data management and infrastructure problems can cripple a digital transformation; however, a great data management strategy will clear the way for data science success. Imagine a scenario in which your data science team predicts, with a high level of accuracy, how much money your customers are likely to spend with your business in the next three months and then delivers that information to you in the dashboard of a tool you already use.
Apache Kafka and the four challenges of production machine learning systems

The nature of machine learning and its core difference from traditional analytics is that it allows the automation of decision-making. Traditional reporting and analytics is generally an input for a human who would ultimately make and carry out the resulting decision manually. Machine learning is aimed at automatically producing an optimal decision. The good news is that by taking the human out of the loop, many more decisions can be made: instead of making one global decision (that is often all humans have time for), automated decision-making allows making decisions continuously and dynamically in a personalized way as part of every customer experience on things that are far too messy for traditional, manually specified business rules. The challenge this presents, though, is that it generally demands directly integrating sophisticated prediction algorithms into production software systems.
Machine Learning And The Future of Finance
Some brokerage and banking functions can be streamlined as investment advisers are cued to sales opportunities by software that considers customers’ past trades and investment preferences. Credit verification already uses a high degree of automation and could be made even more accurate by considering more complex patterns of the borrower and adjusting the weights to these factors based on changes seen in customer behaviour. All these software tools are used within the world of human activities that have consequences for individuals and whose rules are set by humans. For the immediate future, humans will need to give oversight to computer activities and continue to handle the exceptional situations. This is especially true since the reasons a program makes a decision are very different from the heuristics used by humans.
5 Pitfalls Of Self-Service BI
To get value out of BI tools, business units need to feed them data. In general, this means business units stand up and manage their own data marts — subsets of data warehouses that contain data specific to a business line. Because individual business units are typically responsible for all the hardware, software, and data that comprise their data marts in a self-service BI environment, those business units will inevitably create their own definitions and metrics. That's not such a big problem if that business unit is the only user of the data, but it becomes a large problem when trying to compare reports from different business units. "You've gone from a central model where there was tight control of the business metrics, and you've put that in the hands of the masses and it creates conflicting definitions," says Mariani. Mariani notes that during his tenure at Yahoo, the company's business units had myriad definitions of ad impressions and visits.
Quote for the day:
"When human judgment and big data intersect there are some funny things that happen." -- Nate Silver
No comments:
Post a Comment