Quote for the day:
"We are only as effective as our people's perception of us." -- Danny Cox
Why data literacy is essential - and elusive - for business leaders in the AI age

The rising importance of data-driven decision-making is clear but elusive.
However, the trust in the data underpinning these decisions is falling. Business
leaders do not feel equipped to find, analyze, and interpret the data they need
in an increasingly competitive business environment. The added complexity is the
convergence of macro and micro uncertainties -- including economic, political,
financial, technological, competitive landscape, and talent shortage
variables. ... The business need for greater adoption of AI capabilities,
including predictive, generative and agentic AI solutions, is increasing the
need for businesses to have confidence and trust in their data. Survey results
show that higher adoption of AI will require stronger data literacy and access
to trustworthy data. ... The alarming part of the survey is that 54% of business
leaders are not confident in their ability to find, analyze, and interpret data
on their own. And fewer than half of business leaders are sure they can use data
to drive action and decision-making, generate and deliver timely insights, or
effectively use data in their day-to-day work. Data literacy and confidence in
the data are two growth opportunities for business leaders across all lines of
business.
Cyber threats against energy sector surge as global tensions mount
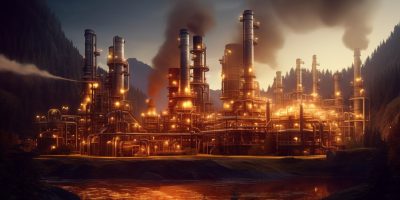
These cyber-espionage campaigns are primarily driven by geopolitical
considerations, as tensions shaped by the Russo-Ukraine war, the Gaza conflict,
and the U.S.’ “great power struggle” with China are projected into cyberspace.
With hostilities rising, potentially edging toward a third world war, rival
nations are attempting to demonstrate their cyber-military capabilities by
penetrating Western and Western-allied critical infrastructure networks.
Fortunately, these nation-state campaigns have overwhelmingly been limited to
espionage, as opposed to Stuxnet-style attacks intended to cause harm in the
physical realm. A secondary driver of increasing cyberattacks against energy
targets is technological transformation, marked by cloud adoption, which has
largely mediated the growing convergence of IT and OT networks. OT-IT
convergence across critical infrastructure sectors has thus made networked
industrial Internet of Things (IIoT) appliances and systems more penetrable to
threat actors. Specifically, researchers have observed that adversaries are
using compromised IT environments as staging points to move laterally into OT
networks. Compromising OT can be particularly lucrative for ransomware actors,
because this type of attack enables adversaries to physically paralyze energy
production operations, empowering them with the leverage needed to command
higher ransom sums.
The Active Data Architecture Era Is Here, Dresner Says
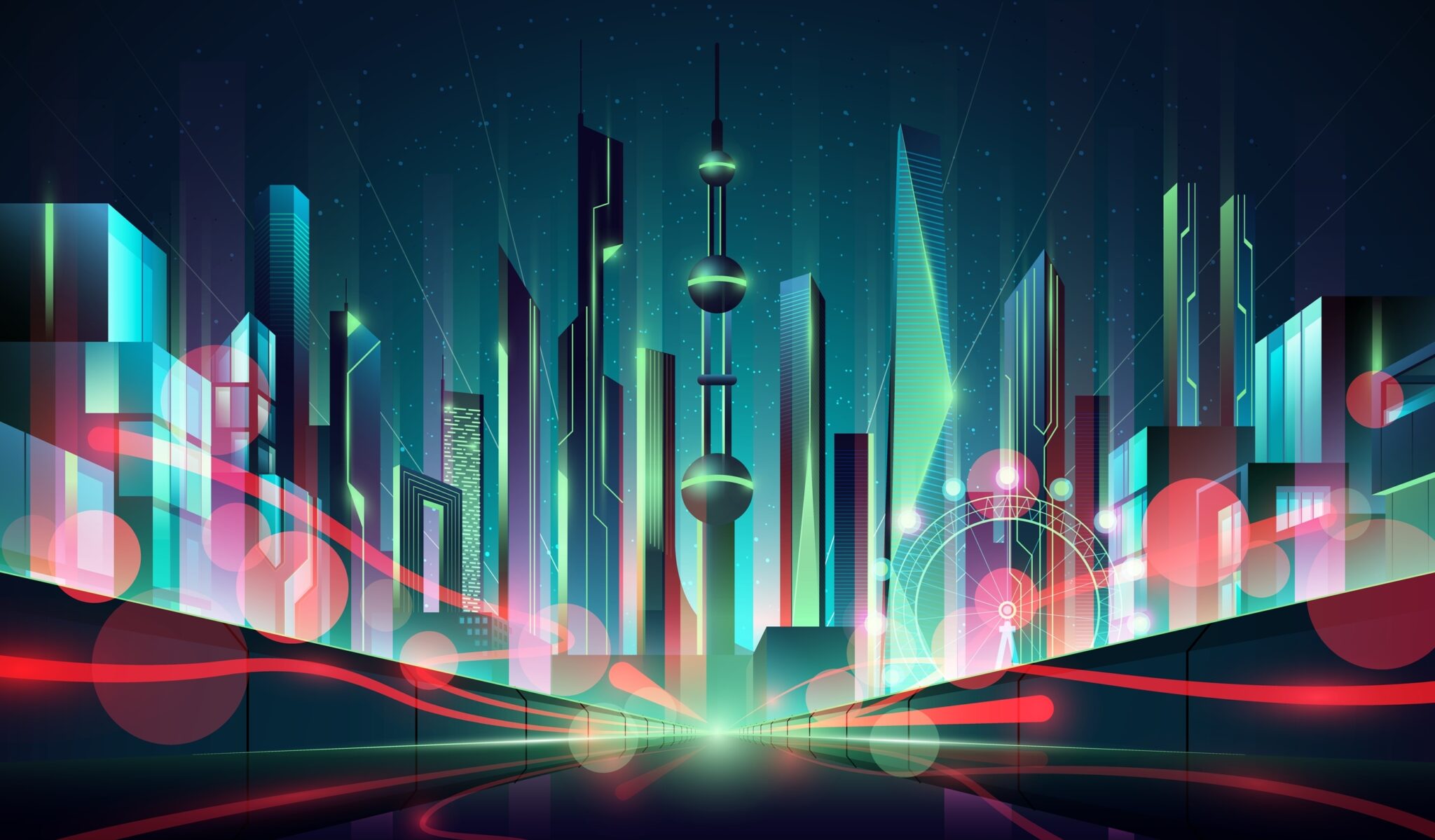
“The buildout of an active data architecture approach to accessing, combining,
and preparing data speaks to a degree of maturity and sophistication in
leveraging data as a strategic asset,” Dresner Advisory Services writes in the
report. “It is not surprising, then, that respondents who rate their BI
initiatives as a success place a much higher relative importance on active
data architecture concepts compared with those organizations that are less
successful.” Data integration is a major component of an active data
architecture, but there are different ways that users can implement data
integration. According to Dresner, the majority of active data architecture
practitioners are utilizing batch and bulk data integration tools, such as
ETL/ELT offerings. Fewer organizations are utilizing data virtualization as
the primary data integration method, or real-time event streaming (i.e. Apache
Kafka) or message-based data movement (i.e. RabbitMQ). Data catalogs and
metadata management are important aspects of an active data architecture. “The
diverse, distributed, connected, and dynamic nature of active data
architecture requires capabilities to collect, understand, and leverage
metadata describing relevant data sources, models, metrics, governance rules,
and more,” Dresner writes.
How can businesses solve the AI engineering talent gap?

“It is unclear whether nationalistic tendencies will encourage experts to
remain in their home countries. Preferences may not only be impacted by
compensation levels, but also by international attention to recent US
treatment of immigrants and guests, as well as controversy at academic
institutions,” says Bhattacharyya. But businesses can mitigate this global
uncertainty, to some extent, by casting their hiring net wider to include
remote working. Indeed, Thomas Mackenbrock, CEO-designate of Paris
headquartered BPO giant Teleperformance says that the company’s global
footprint helps it to fulfil AI skills demand. “We’re not reliant on any
single market [for skills] as we are present in almost 100 markets,” explains
Mackenbrock. ... “The future workforce will need to combine human ingenuity
with new and emerging AI technologies; going beyond just technical skills
alone,” says Khaled Benkrid, senior director of education and research at Arm.
“Academic institutions play a pivotal role in shaping this future workforce.
By collaborating with industry to conduct research and integrate AI into their
curricula, they ensure that graduates possess the skills required by the
industry. “Such collaborations with industry partners keep academic programs
aligned with research frontiers and evolving job market demands, creating a
seamless transition for students entering the workforce,” says Benkrid.
Breaking Down the Walls Between IT and OT
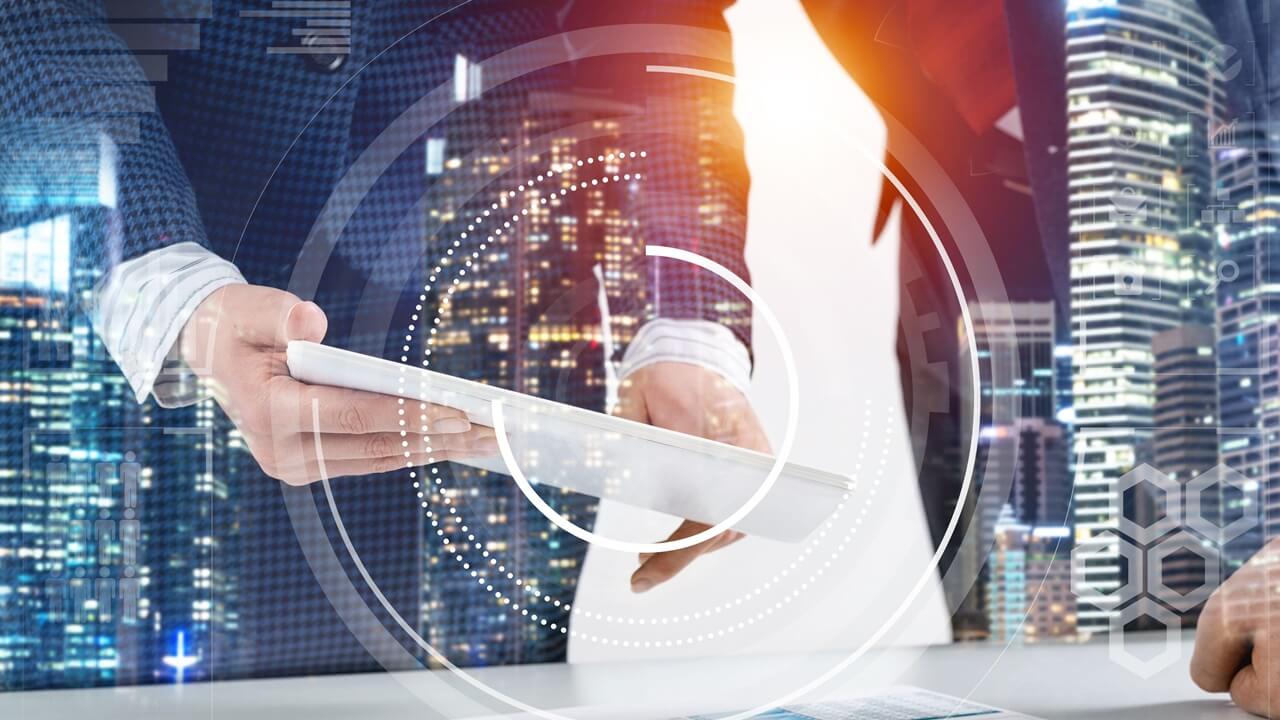
“Even though there's cyber on both sides, they are fundamentally different in
concept,” Ian Bramson, vice president of global industrial cybersecurity at
Black & Veatch, an engineering, procurement, consulting, and construction
company, tells InformationWeek. “It's one of the things that have kept them
more apart traditionally.” ... “OT is looked at as having a much longer
lifespan, 30 to 50 years in some cases. An IT asset, the typical laptop these
days that's issued to an individual in a company, three years is about when
most organization start to think about issuing a replacement,” says Chris
Hallenbeck, CISO for the Americas at endpoint management company Tanium. ...
The skillsets required of the teams to operate IT and OT systems are also
quite different. On one side, you likely have people skilled in traditional
systems engineering. They may have no idea how to manage the programmable
logic controllers (PLC) commonly used in OT systems. The divide between IT and
OT has been, in some ways, purposeful. The Purdue model, for example, provides
a framework for segmenting ICS networks, keeping them separate from corporate
networks and the internet. ... Cyberattack vectors on IT and OT environments
look different and result in different consequences. “On the IT side, the
impact is primarily data loss and all of the second order effects of your data
getting stolen or your data getting held for ransom,” says Shankar.
Are Return on Equity and Value Creation New Metrics for CIOs?
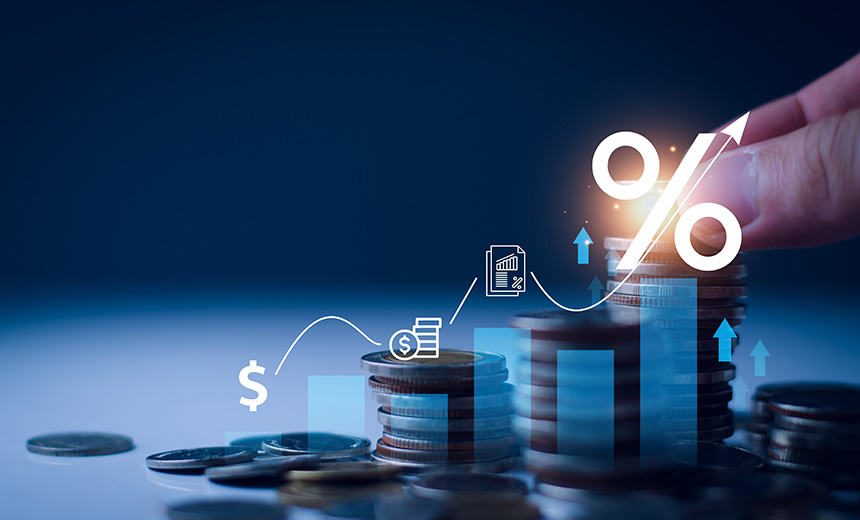
While driving efficiency is not a new concept for technology leaders, what
is different today is the scale and significance of their efforts. In many
organizations, CIOs are being tasked with reimagining how value is
generated, assessed and delivered. ... Traditionally, technology ROI
discussions have focused on cost savings, automation consolidation and
reduced headcount. But that perspective is shifting rapidly. CIOs are now
prioritizing customer acquisition, retention pricing power and speed to
market. CIOs also play an integral role in product innovation than ever
before. To remain relevant, they must speak the language of gross margin,
not just uptime. This evolution is increasingly reflected in boardroom
conversations. CIOs once presented dashboards of uptime and service-level
agreements, but today, they discuss customer value, operational efficiency
and platform monetization. ... In some cases, technology leaders scale too
quickly before proving value. For example, expensive cloud migrations may
proceed without a corresponding shift in the business model. This can result
in data lakes with no clear application or platforms launched without
product-market fit. These missteps can severely undermine ROE.
AI brings order to observability disorder

Artificial intelligence has contributed to complexity. Businesses now want
to monitor large language models as well as applications to spot anomalies
that may contribute to inaccuracies, bias, and slow performance. Legacy
observability systems were never designed for the ability to bring together
these disparate sources of data. A unified observability platform leveraging
AI can radically simplify the tools and processes for improved visibility
and resolving problems faster, enabling the business to optimize operations
based on reliable insights. By consolidating on one set of integrated
observability solutions, organizations can lower costs, simplify complex
processes, and enable better cross-function collaboration. “Noise overwhelms
site reliability engineering teams,” says Gagan Singh, Vice President of
Product Marketing at Elastic. Irrelevant and low-priority alerts can
overwhelm engineers, leading them to overlook critical issues and delaying
incident response. Machine learning models are ideally suited to
categorizing anomalies and surfacing relevant alerts so engineers can focus
on critical performance and availability issues. “We can now leverage GenAI
to enable SREs to surface insights more effectively,” Singh says.
Why Most IaC Strategies Still Fail — And How To Fix Them

There are a few common reasons IaC strategies fail in practice. Let’s
explore what they are, and dive into some practical, battle-tested fixes to
help teams regain control, improve consistency and deliver on the original
promise of IaC. ... Without a unified direction, fragmentation sets in.
Teams often get locked into incompatible tooling — some using AWS
CloudFormation for perceived enterprise alignment, others favoring Terraform
for its flexibility. These tool silos quickly become barriers to
collaboration. ... Resistance to change also plays a role. Some engineers
may prefer to stick with familiar interfaces and manual operations, viewing
IaC as an unnecessary complication. Meanwhile, other teams might be fully
invested in reusable modules and automated pipelines, leading to fractured
workflows and collaboration breakdowns. Successful IaC implementation
requires building skills, bridging silos and addressing resistance with
empathy and training — not just tooling. To close the gap, teams need clear
onboarding plans, shared coding standards and champions who can guide others
through real-world usage — not just theory. ... Drift is inevitable: manual
changes, rushed fixes and one-off permissions often leave code and reality
out of sync. Without visibility into those deviations, troubleshooting
becomes guesswork.
What will the sustainable data center of the future look like?
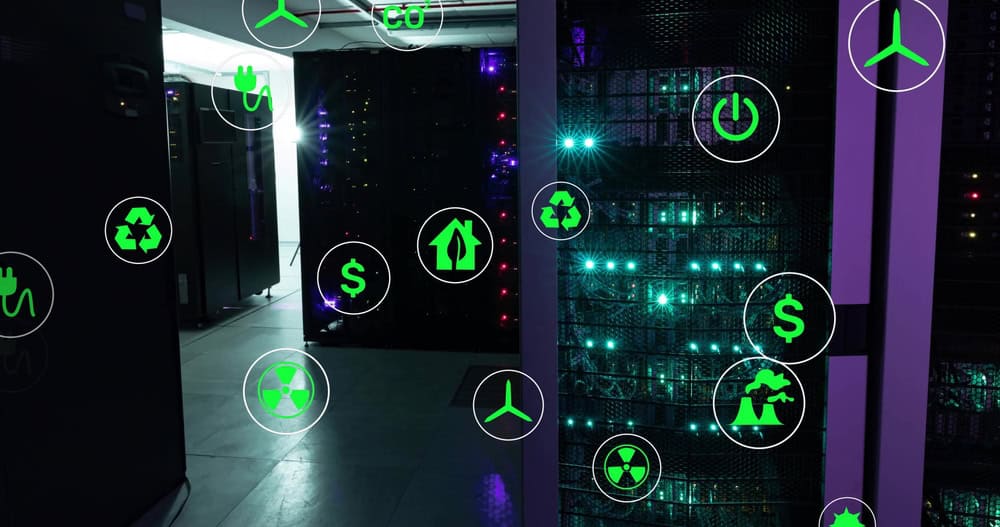
The energy issue not only affects operators/suppliers. If a customer uses a
lot of energy, they will get a bill to match, says Van den Bosch. “I [as a
supplier] have to provide the customer with all kinds of details about my
infrastructure. That includes everything from air conditioning to the
specific energy consumption of the server racks. The customer is then able
to reduce that energy consumption.” This can be done, for example, by
replacing servers earlier than they have been before, a departure from the
upgrade cycles of yesteryear. Ruud Mulder of Dell Technologies calls for the
sustainability of equipment to be made measurable in great detail. This can
be done by means of a digital passport, showing where all the materials come
from and how recyclable they are. He thinks there is still much room for
improvement in this area. For example, future designs can be recycled better
by separating plastic and gold from each other, refurbishing components and
more. This yield increase is often attractive, as more computing power is
required for ambitious AI plans, and the efficiency of chips increases with
each generation. “The transition to AI means that you sometimes have to say
goodbye to your equipment sooner,” says Mulder. The AI issue is highly
relevant to the future of the modern data center in any case.
Fitness Functions for Your Architecture
/articles/fitness-functions-architecture/en/smallimage/fitness-functions-architecture-thumbnail-1744359549803.jpg)
Fitness functions offer us self-defined guardrails for certain aspects of
our architecture. If we stay within certain (self-chosen) ranges, we're safe
(our architecture is "good"). ... Many projects already use some kinds of
fitness functions, although they might not use the term. For example,
metrics from static code checkers, linters, and verification tools (such as
PMD, FindBugs/SpotBugs, ESLint, SonarQube, and many more). Collecting the
metrics alone doesn't make it a fitness function, though. You'll need fast
feedback for your developers, and you need to define clear measures: limits
or ranges for tolerated violations and actions to take if a metric indicates
a violation. In software architecture, we have certain architectural styles
and patterns to structure our code in order to improve understandability,
maintainability, replaceability, and so on. Maybe the most well-known
pattern is a layered architecture with, quite often, a front-end layer above
a back-end layer. To take advantage of such layering, we'll allow and
disallow certain dependencies between the layers. Usually, dependencies are
allowed from top to down, i.e. from the front end to the back end, but not
the other way around. A fitness function for a layered architecture will
analyze the code to find all dependencies between the front end and the back
end.
No comments:
Post a Comment