Quote for the day:
"The most powerful leadership tool you have is your own personal example." -- John Wooden
How to lead humans in the age of AI
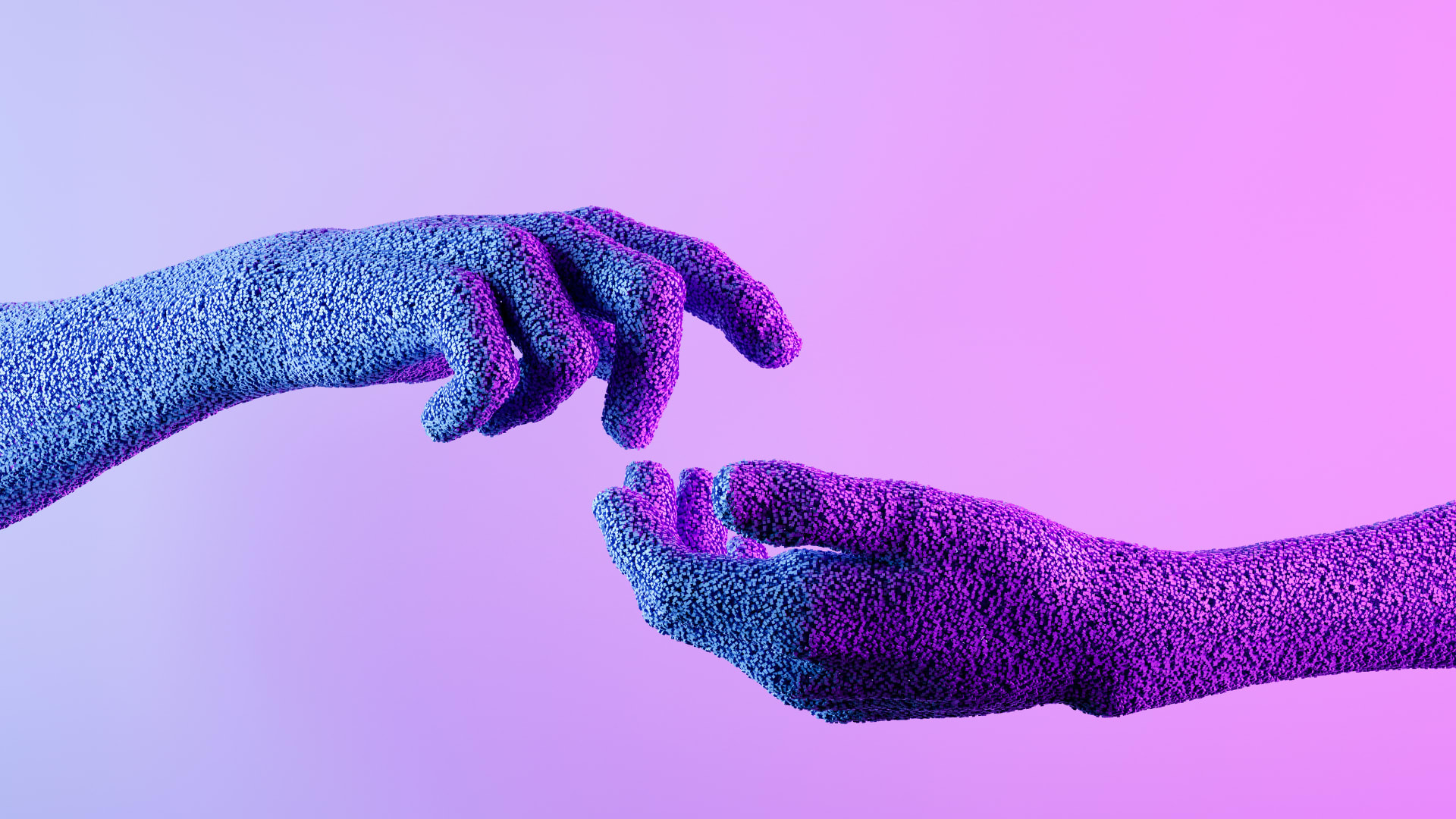
Quiet the noise around AI and you will find the simple truth that the most
crucial workplace capabilities remain deeply human. ... This human skills gap is
even more urgent when Gen Z is factored in. They entered the workforce aligned
with a shift to remote and hybrid environments, resulting in fewer opportunities
to hone interpersonal skills through real-life interactions. This is not a
critique of an entire generation, but rather an acknowledgment of a broad
workplace challenge. And Gen Z is not alone in needing to strengthen
communication across generational divides, but that is a topic for another
day. ... Leaders must embrace their inner improviser. Yes, improvisation,
like what you have watched on Whose Line Is It Anyway? Or the awkward
performance your college roommate invited you to in that obscure college lounge.
The skills of an improviser are a proven method for striving amidst uncertainty.
Decades of experience at Second City Works and studies published by The
Behavioral Scientist confirm the principles of improv equip us to handle change
with agility, empathy, and resilience. ... Make listening intentional and
visible. Respond with the phrase, “So what I’m hearing is,” followed by
paraphrasing what you heard. Pose thoughtful questions that indicate your
priority is understanding, not just replying.
When companies merge, so do their cyber threats
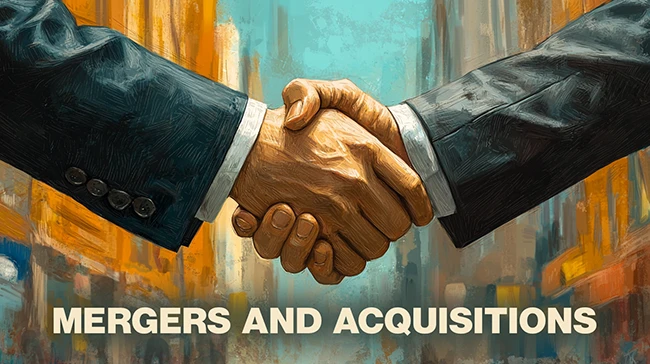
Merging two companies means merging two security cultures. That is often harder
than unifying tools or policies. While the technical side of post-M&A
integration is important, it’s the human and procedural elements that often
introduce the biggest risks. “When CloudSploit was acquired, one of the most
underestimated challenges wasn’t technical, it was cultural,” said Josh
Rosenthal, Holistic Customer Success Executive at REPlexus.com. “Connecting two
companies securely is incredibly complex, even when the acquired company is much
smaller.” Too often, the focus in M&A deals lands on surface-level
assurances like SOC 2 certifications or recent penetration tests. While
important, those are “table stakes,” Rosenthal noted. “They help, but they don’t
address the real friction: mismatched security practices, vendor policies, and
team behaviors. That’s where M&A cybersecurity risk really lives.” As AI
accelerates the speed and scale of attacks, CISOs are under increasing pressure
to ensure seamless integration. “Even a phishing attack targeting a vendor
onboarding platform can introduce major vulnerabilities during the M&A
process,” Rosenthal warned. To stay ahead of these risks, he said, smart
security leaders need to dig deeper than documentation.
Measuring success in dataops, data governance, and data security

If you are on a data governance or security team, consider the metrics that
CIOs, chief information security officers (CISOs), and chief data officers
(CDOs) will consider when prioritizing investments and the types of initiatives
to focus on. Amer Deeba, GVP of Proofpoint DSPM Group, says CIOs need to
understand what percentage of their data is valuable or sensitive and quantify
its importance to the business—whether it supports revenue, compliance, or
innovation. “Metrics like time-to-insight, ROI from tools, cost savings from
eliminating unused shadow data, or percentage of tools reducing data incidents
are all good examples of metrics that tie back to clear value,” says Deeba. ...
Dataops technical strategies include data pipelines to move data, data streaming
for real-time data sources like IoT, and in-pipeline data quality automations.
Using the reliability of water pipelines as an analogy is useful because no one
wants pipeline blockages, leaky pipes, pressure drops, or dirty water from their
plumbing systems. “The effectiveness of dataops can be measured by tracking the
pipeline success-to-failure ratio and the time spent on data preparation,” says
Sunil Kalra, practice head of data engineering at LatentView. “Comparing planned
deployments with unplanned deployments needed to address issues can also provide
insights into process efficiency.”
How Safe Is the Code You Don’t Write? The Risks of Third-Party Software
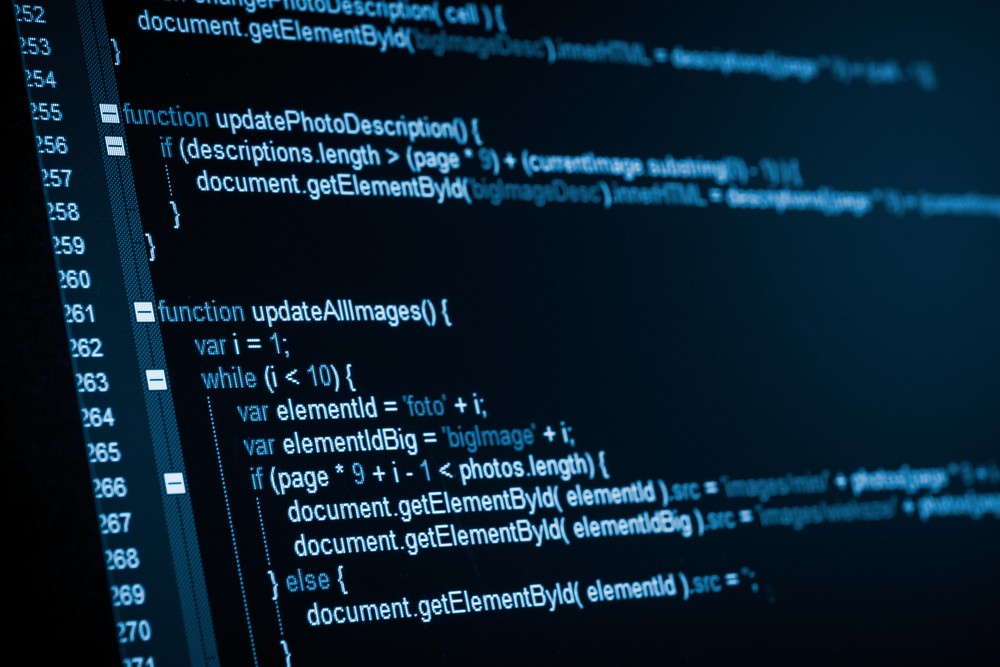
Open-source and commercial packages and public libraries accelerate innovation,
drive down development costs, and have become the invisible scaffolding of the
Internet. GitHub recently highlighted that 99% of all software projects use
third-party components. But with great reuse comes great risk. Third-party code
is a double-edged sword. On the one hand, it’s indispensable. On the other hand,
it’s a potential liability. In our race to deliver software faster, we’ve
created sprawling software supply chains with thousands of dependencies, many of
which receive little scrutiny after the initial deployment. These dependencies
often pull in other dependencies, each one potentially introducing outdated,
vulnerable, or even malicious code into environments that power
business-critical operations. ... The risk is real, so what do we do? We can
start by treating third-party code with the same caution and scrutiny we apply
to everything else that enters the production pipeline. This includes
maintaining a living inventory of all third-party components across every
application and monitoring their status to prescreen updates and catch
suspicious changes. With so many ways for threats to hide, we can’t take
anything on trust, so next comes actively checking for outdated or vulnerable
components as well as new vulnerabilities introduced by third-party
code.
The AI Leadership Crisis: Why Chief AI Officers Are Failing (And How To Fix It)
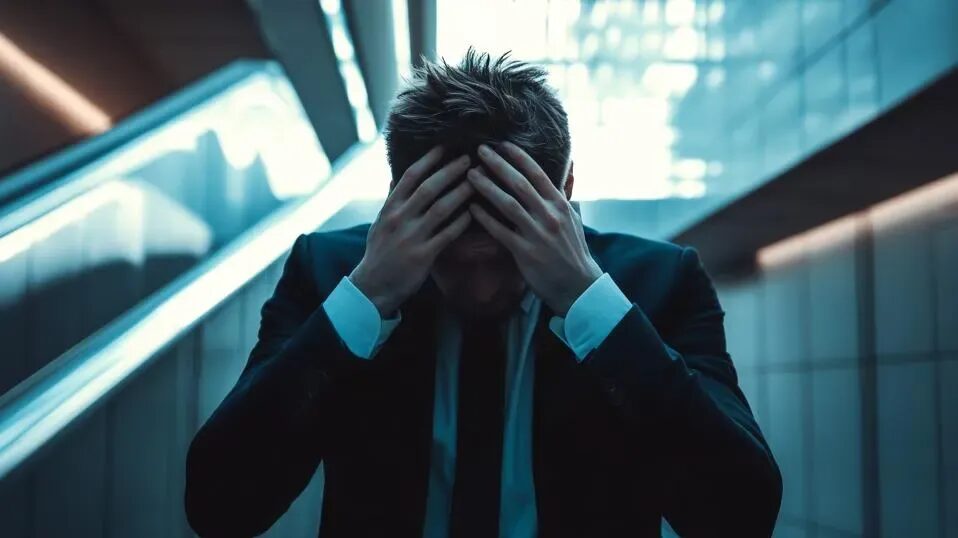
Perhaps the most dangerous challenge facing CAIOs is the profound disconnect
between expectations and reality. Many boards anticipate immediate,
transformative results from AI initiatives – the digital equivalent of demanding
harvest without sowing. AI transformation isn't a sprint; it's a marathon with
hurdles. Meaningful implementation requires persistent investment in data
infrastructure, skills development, and organizational change management. Yet
CAIOs often face arbitrary deadlines that are disconnected from these realities.
One manufacturing company I worked with expected their newly appointed CAIO to
deliver $50 million in AI-driven cost savings within 12 months. When those
unrealistic targets weren't met, support for the role evaporated – despite
significant progress in building foundational capabilities. ... There are
many potential risks of AI, from bias to privacy concerns, and the right level
of governance is essential. CAIOs are typically tasked with ensuring responsible
AI use yet frequently lack the authority to enforce guidelines across
departments. This accountability-without-authority dilemma places CAIOs in an
impossible position. They're responsible for AI ethics and risk management, but
departmental leaders can ignore their guidance with minimal consequences.
OT security: how AI is both a threat and a protector
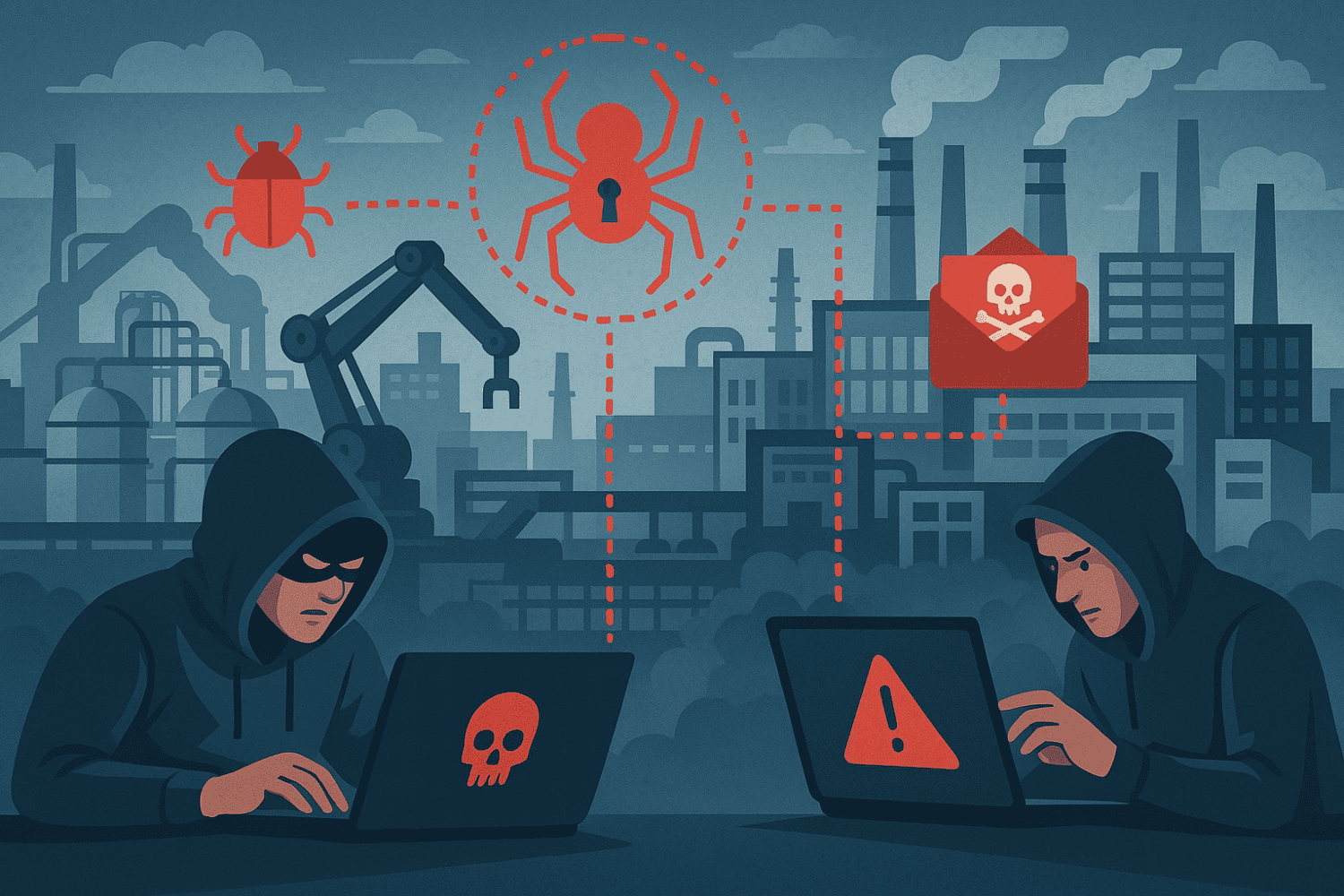
Burying one’s head in the sand, a favorite pastime among some OT personnel, no
longer works. Security through obscurity is and remains a bad idea. Heinemeyer:
“I’m not saying that everyone will be hacked, but it is increasingly likely
these days.” Possibly, the ostrich policy has to do with, yes, the reporting on
OT vulnerabilities, including by yours truly. Ancient protocols, ICS systems and
PLCs with exploitable vulnerabilities are evidently risk factors. However, the
people responsible for maintaining these systems at manufacturing and utility
facilities know better than anyone that the actual exploits of these obscure
systems are improbable. ... Given the increasing threat, is the new focus on
common best practices enough? We have already concluded that vulnerabilities
should not be judged solely on the CVSS score. They are an indication,
certainly, but a combination of CVEs with middle-of-the-range scoring appears to
have the most serious consequences. Heinemeyer says that the resolve to identify
all vulnerabilities as the ultimate solution was well established from the 1990s
to the 2010s. He says that in recent years, security professionals have realized
that specific issues need to be prioritized, quantifying technical
exploitability through various measurements (e.g., EPSS).
In a Social Engineering Showdown: AI Takes Red Teams to the Mat

In a revelation that shouldn’t surprise, but still should alarm security
professionals, AI has gotten much more proficient in social engineering. Back in
the day, AI was 31% less effective than human beings in creating simulated
phishing campaigns. But now, new research from Hoxhunt suggests that the
game-changing technology’s phishing performance against elite human red teams
has improved by 55%. ... Using AI offensively can raise legal and regulatory
hackles related to privacy laws and ethical standards, Soroko adds, as well as
creating a dependency risk. “Over-reliance on AI could diminish human expertise
and intuition within cybersecurity teams.” But that doesn’t mean bad actors will
win the day or get the best of cyber defenders. Instead, security teams could
and should turn the tables on them. “The same capabilities that make AI an
effective phishing engine can — and must — be used to defend against it,” says
Avist. With an emphasis on “must.” ... It seems that tried and true basics are a
good place to start. “Ensuring transparency, accountability and responsible use
of AI in offensive cybersecurity is crucial,” Kowski. As with any aspect of tech
and security, keeping AI models “up-to-date with the latest threat intelligence
and attack techniques is also crucial,” he says. “Balancing AI capabilities with
human expertise remains a key challenge.”
Optimizing CI/CD for Trust, Observability and Developer Well-Being

While speed is often cited as a key metric for CI/CD pipelines, the quality and
actionability of the feedback provided are equally, if not more, important for
developers. Jones, emphasizing the need for deep observability, stresses, “Don’t
just tell me that the steps of the pipeline succeeded or failed, quantify that
success or failure. Show me metrics on test coverage and show me trends and
performance-related details. I want to see stack traces when things fail. I want
to be able to trace key systems even if they aren’t related to code that I’ve
changed because we have large complex architectures that involve a lot of
interconnected capabilities that all need to work together.” This level of
technical insight empowers developers to understand and resolve issues quickly,
highlighting the importance of implementing comprehensive monitoring and logging
within your CI/CD pipeline to provide developers with detailed insights into
build, test, and deployment processes. And shifting feedback earlier in the
development lifecycle serves everyone well. The key is shifting feedback earlier
in the process, ensuring it is contextual, before code is merged. For example,
running security scans at the pull request stage, rather than after deployment,
ensures developers get actionable feedback while still in context.
AI agents vs. agentic AI: What do enterprises want?

If AI and AI agents are application components, then they fit both into business
processes and workflow. A business process is a flow, and these days at least
part of that flow is the set of data exchanges among applications or their
components—what we typically call a “workflow.” It’s common to think of the
process of threading workflows through both applications and workers as a
process separate from the applications themselves. Remember the “enterprise
service bus”? That’s still what most enterprises prefer for business processes
that involve AI. Get an AI agent that does something, give it the output of some
prior step, and let it then create output for the step beyond it. The decision
as to whether an AI agent is then “autonomous” is really made by whether its
output goes to a human for review or is simply accepted and implemented. ...
What enterprises like about their vision of an AI agent is that it’s possible to
introduce AI into a business process without having AI take over the process or
require the process be reshaped to accommodate AI. Tech adoption has long
favored strategies that let you limit scope of impact, to control both cost and
the level of disruption the technology creates. This favors having AI integrated
with current applications, which is why enterprises have always thought of AI
improvements to their business operation overall as being linked to
incorporating AI into business analytics.
Liquid Cooling is ideal today, essential tomorrow, says HPE CTO
We’re moving from standard consumption levels—like 1 kilowatt per rack—to as
high as 3 kilowatts or more. The challenge lies in provisioning that much power
and doing it sustainably. Some estimates suggest that data centers, which
currently account for about 1% of global power consumption, could rise to 5% if
trends continue. This is why sustainability isn’t just a checkbox anymore—it’s a
moral imperative. I often ask our customers: Who do you think the world belongs
to? Most pause and reflect. My view is that we’re simply renting the world from
our grandchildren. That thought should shape how we design infrastructure today.
... Air cooling works until a point. But as components become denser, with more
transistors per chip, air struggles. You’d need to run fans faster and use more
chilled air to dissipate heat, which is energy-intensive. Liquid, due to its
higher thermal conductivity and density, absorbs and transfers heat much more
efficiently. Some DLC systems use cold plates only on select components. Others
use them across the board. There are hybrid solutions too, combining liquid and
air. But full DLC systems, like ours, eliminate the need for fans altogether.
... Direct liquid cooling (DLC) is becoming essential as data centers
support AI and HPC workloads that demand high performance and density.
No comments:
Post a Comment