Generative AI and the legal landscape: Evolving regulations and implications

So far we’ve seen AI giants as the primary targets of several lawsuits that
revolve around their use of copyrighted data to create and train their models.
Recent class action lawsuits filed in the Northern District of California,
including one filed on behalf of authors and another on behalf of aggrieved
citizens raise allegations of copyright infringement, consumer protection and
violations of data protection laws. These filings highlight the importance of
responsible data handling, and may point to the need to disclose training data
sources in the future. However, AI creators like OpenAI aren’t the only
companies dealing with the risk presented by implementing gen AI models. When
applications rely heavily on a model, there is risk that one that has been
illegally trained can pollute the entire product. ... It is clear that CEOs feel
pressure to embrace gen AI tools to augment productivity across their
organizations. However, many companies lack a sense of organizational readiness
to implement them. Uncertainty abounds while regulations are hammered out, and
the first cases prepare for litigation.
Cars are a ‘privacy nightmare on wheels’
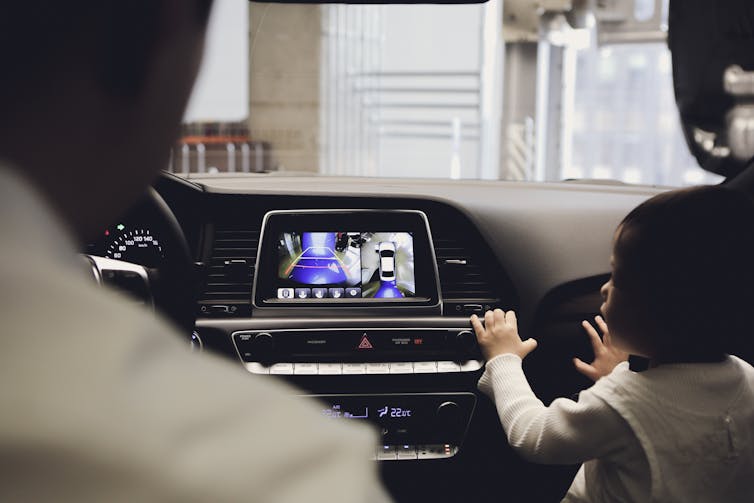
Apart from data entered directly into a car’s “infotainment” system, many cars
can collect data in the background via cameras, microphones, sensors and
connected phones and apps. A lot of these data are used, at least in part, for
legitimate purposes such as making driving more enjoyable and safer for the
driver, passengers and pedestrians. But they can also be supplemented with data
collected from other sources and used for other purposes. For instance, data may
be collected from your website visit, your test drive at a dealership, or from
third parties including “marketing agencies” and “providers of data-collecting
devices, products or systems that you use”. ... It’s safe to say car
manufacturers generally don’t want privacy laws tightened. The Federal Chamber
of Automotive Industries (FCAI) represents companies distributing 68 brands of
various types of vehicles in Australia. During the recent review of our privacy
legislation, the FCAI made a submission to the Attorney General’s department
arguing against many of the privacy law reforms under consideration.
The Impact of AI and Machine Learning in HR: Enhancing Recruitment and Employee Engagement
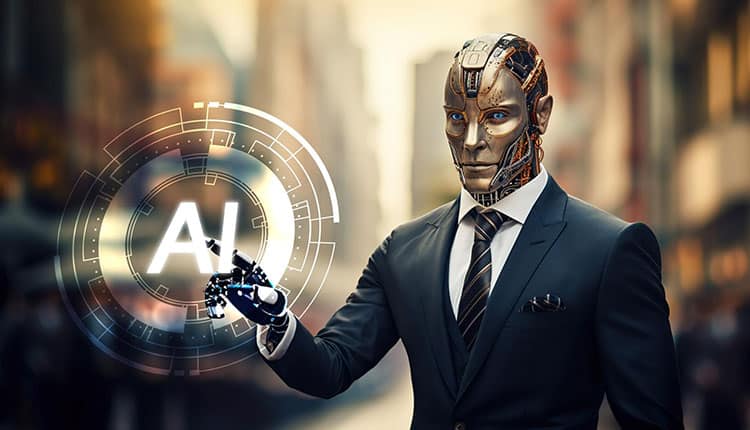
Amid a new digital landscape, rising employee expectations, and evolving
business dynamics, HR professionals contend with a slew of challenges. Adapting
HR processes and systems to digital transformation, especially in organisations
with legacy systems can prove demanding. HR leaders today grapple with tasks
ranging from keeping up with talent acquisition in the digital world and rapidly
evolving HR technology such as HRIS, AI Tools, and Data Analytics to boost
employee engagement. They also have to adapt to various recruitment strategies
to find the right talent in a competitive job market. While navigating these
challenges, leaders should also remain vigilant of potential advantages on the
horizon. These encompass enhancing the overall employee journey, embracing a
variety of learning and growth initiatives, and streamlining decision-making
through AI to enhance results while safeguarding efficiency. Furthermore, AI can
analyze large amounts of data quickly, empowering decision-makers with useful
insights to help them make informed decisions. This data-driven decision-making
can result in better resource allocation, better strategy, and increased work
satisfaction.
Microsoft to create team dedicated to data center automation and robotics
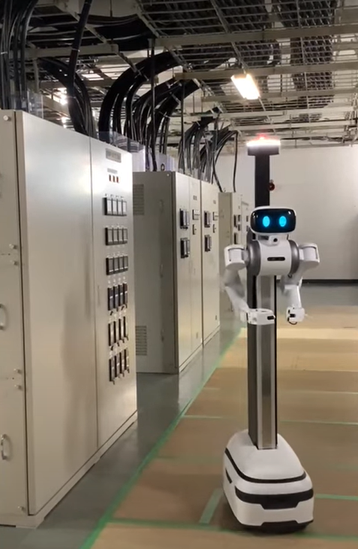
The move comes a month after a Microsoft Azure outage in Australia was partially
blamed on poor software automation. A utility power sag tripped cooling units,
shutting them down and causing temperatures to rise. With insufficient staff on
site to reboot the units, the automated system shut down servers to protect them
from overheating. Instead, the system could have been designed to reboot the
cooling units. “We are exploring ways to improve existing automation to be more
resilient to various voltage sag event types,” Microsoft said in a post-mortem.
Robots and data centers have a long history, with numerous companies and
research groups trying to build computers that could look after fellow
computers. ... "As far as robotics, our hyperscale data centers are more like
warehouses and most of the processes require a robot to navigate to a specific
location to perform a task," Google's VP of data centers Joe Kava told DCD in
2021. “However, even as advanced as robotics have become, many of the tests in
data centers are much more complicated than in other industries that have
employed large-scale robotic implementations."
What does the DPDP Act mean for philanthropy in India?
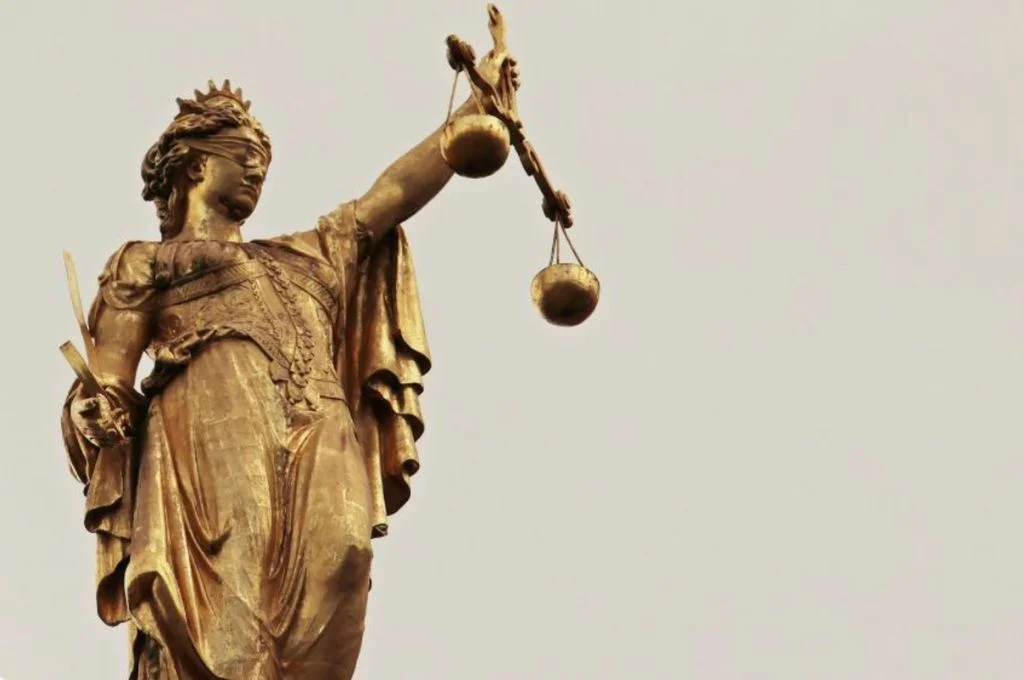
Given the stringent requirements of the DPDP Act, there’s a pressing need for
revisiting and potentially revising the CSR guidelines. Striking a balance
between accountability and privacy becomes crucial in ensuring compliance with
both CSR and data protection mandates. While accountability remains paramount,
it’s time to transition from rigid metrics to narratives of change. By fostering
relationships built on mutual respect and shared learning, practices followed by
donor organisations can resonate with the ethos of the DPDP Act and nurture a
more collaborative philanthropic ecosystem. This necessitates a fundamental
rethinking of how social impact can be measured, and shifting the focus from
data collection to storytelling and community empowerment. By upholding privacy
and agency, as per Sections 6 and 12, the law provides an opening to develop
more participatory and human-centred evaluation frameworks. Funders are pivotal
in enabling this evolution by modifying expectations, building capacity, and
championing new trust-based and collaborative models of assessing progress.
LLMs Demand Observability-Driven Development
With good observability data, you can use that same data to feed back into your
evaluation system and iterate on it in production. The first step is to use this
data to evaluate the representativity of your production data set, which you can
derive from the quantity and diversity of use cases. You can make a surprising
amount of improvements to an LLM based product without even touching any prompt
engineering, simply by examining user interactions, scoring the quality of the
response, and acting on the correctable errors (mainly data model mismatches and
parsing/validation checks). You can fix or handle for these manually in the
code, which will also give you a bunch of test cases that your corrections
actually work! These tests will not verify that a particular input always yields
a correct final output, but they will verify that a correctable LLM output can
indeed be corrected. You can go a long way in the realm of pure software,
without reaching for prompt engineering. But ultimately, the only way to improve
LLM-based software is by adjusting the prompt, scoring the quality of the
responses, and readjusting accordingly.
Feds Warn Healthcare Sector of 'NoEscape' RaaS Gang Threats
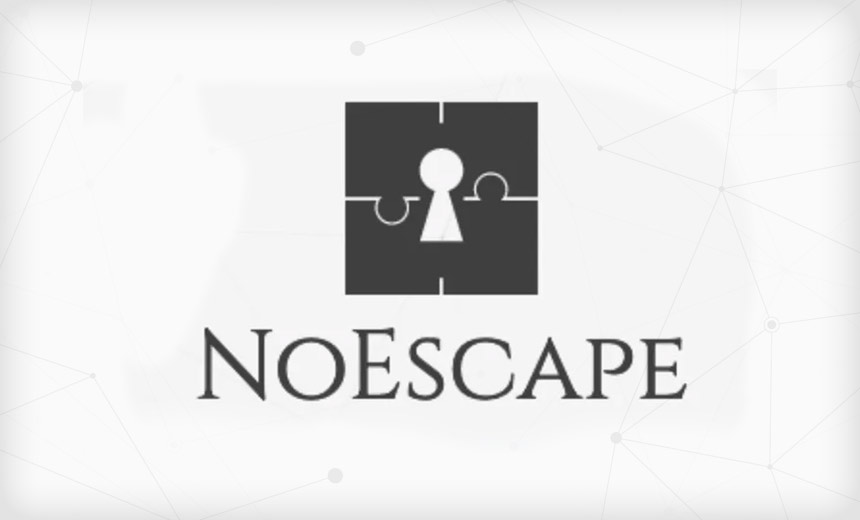
The developers of NoEscape ransomware are unknown but they claim to have created
their malware and associated infrastructure "entirely from scratch," HHS HC3
said. But security researchers have noted that the ransomware encryptors of
NoEscape and Avaddon’s are nearly identical, with only one notable change in
encryption algorithms, HHS HC3 wrote. "Previously, the Avaddon encryptor
utilized AES for file encryption, with NoEscape switching to the Salsa20
algorithm. Otherwise, the encryptors are virtually identical, with the
encryption logic and file formats almost identical, including a unique way of
'chunking of the RSA-encrypted blobs.'” While researchers have observed evidence
suggesting that NoEscape is related to Avaddon, unlike Avaddon, it has yet to be
determined if there is a free NoEscapte decryptor that organizations can utilize
to recover the encrypted files, HHS HC3 said. "Until then, unless certain
detection and prevention method are put in place, a successful exploitation by
NoEscape ransomware will almost certainly result in the encryption and
exfiltration of significant quantities of data."
5 Steps For Building Your Enterprise Semantic Recommendation Engine
After creating the supporting data models, the next step in building a semantic
recommendation engine is to construct the graph. The graph acts as a database of
nodes and connections between nodes (called edges) that houses all of the
content relationships defined in the ontology model. Building the graph involves
both ingesting and enriching source data. Ingestion maps raw data to nodes and
edges in the graph. Enrichment appends additional attributes, tags, and metadata
to enhance the data. This enriched data is then be transformed into semantic
triples, which are subject-predicate-object structures that capture
relationships. In our example, the healthcare provider could transform their
enriched data into triples that capture the relationships between diagnoses and
medical subjects, and medical subjects and content. Converting data into a web
of semantic triples and loading it into the graph enables efficient querying.
The knowledge graph’s flexibility also enables continuous integration of new
data to keep recommendations relevant.
Agile Architecture: A Comparison of TOGAF and SAFe Framework for Agile Enterprise Architecture
In TOGAF, the Enterprise Architect plays a vital role in creating,
maintaining, and evolving the enterprise architecture of an organization. They
are responsible for aligning business and IT strategies, processes, and
systems, and ensuring that the architecture supports the organization’s goals
and objectives. The Enterprise Architect in TOGAF follows the Architecture
Development Method (ADM), a structured approach that guides the creation and
implementation of enterprise architecture. ... In SAFe, the Enterprise
Architect has a slightly different focus and responsibilities. While the core
principles of enterprise architecture remain the same, the Enterprise
Architect in SAFe works within the context of Agile development practices and
the broader framework of SAFe. They collaborate closely with Agile teams as
well as other architect roles and play a crucial role in providing technical
leadership, guidance, and support. The Enterprise Architect in SAFe helps
teams align their technical solutions with the overall enterprise
architecture, ensuring that the architectural vision is realized, and
technical debt is managed effectively.
Cyber Insecurity, AI and the Rise of the CISO

Adding to cyber insecurity is the unease in the use of artificial intelligence
not only by public employees but by cyber criminals too. It comes as no
surprise that artificial intelligence (AI) is being used by cyber criminals to
further exploit cyber weaknesses and vulnerabilities. In PTI’s City and County
AI Survey, AI was listed as the No. 1 application to help thwart cyberattacks.
They recognize how AI can actively scan for suspicious patterns and anomalies
as well as assist in remediation and recovery strategies. What’s more AI
systems continue to learn and act. Also new this year is the renewed focus on
zero trust frameworks and strategies. Zero trust has never been more critical
and unfortunately it takes both time and talent to fully comprehend all its
dependencies leading towards deployment. This year also saw for the first time
in years the National Institute of Standards and Technology (NIST) has
modified its Cybersecurity Framework to include an underlying layer of
governance in each of its traditional five pillars.
Quote for the day:
"Great leaders do not desire to lead
but to serve." -- Myles Munroe
No comments:
Post a Comment