What You Need to Know About Neuromorphic Computing
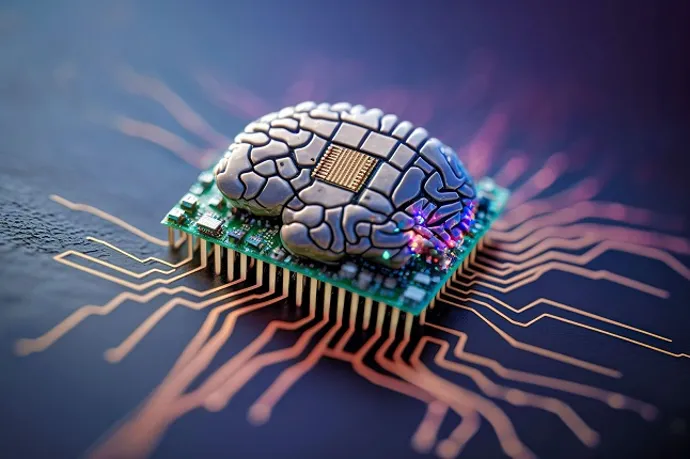
Neuromorphic computing is a type of computer engineering that mimics the human
brain and nervous system. “It's a hardware and software computing element that
combines several specializations, such as biology, mathematics, electronics, and
physics,” explains Abhishek Khandelwal, vice president, life sciences, at
engineering consulting firm Capgemini Engineering. While current AI technology
has become better at outperforming human capabilities in multiple fields, such
as Level 4 self-driving vehicles and generative models, it still offers only a
crude approximation of human/biological capabilities and is only useful in a
handful of fields. ... Neuromorphic supporters believe the technology will lead
to more intelligent systems. “Such systems could also learn automatically and
self-regulate what to learn and where to learn from,” Natarajan says. Meanwhile,
combining neuromorphic technology with neuro-prosthetics, (such as Neuralink)
could lead to breakthroughs in prosthetic limb control and various other types
of human assistive and augmented technologies.
How the influence of data and the metaverse will revolutionize businesses and industries
Today, business is all about data: collecting, storing, transforming, and
analysing it to gain insights—to make decisions. Just like how ChatGPT requires
massive amounts of data to create human-like language, businesses need data to
augment human decision-making. From machine and building performance to energy
and emissions, data is the crucial link between the physical and digital worlds.
It’s also the key to solving efficiency and sustainability challenges that are
now more urgent than ever. If the metaverse is meant to transform business and
industries, it must be built on solid data foundations. ... Digital
transformation started with connecting physical assets via IoT and edge
controls. Its disruptive potential has proven to carry operational and energy
efficiency across all levels of an enterprise. When we introduce powerful
software capabilities and start leveraging the generated data, we can create
virtual representations of the real world by combining simulation, augmented
reality (AR), data sharing, and visualization all at once.
Distributed Tracing Is Failing. How Can We Save It?

Engineers are to some degree creatures of habit. The engineering organizations
I’ve spent time with have a deep level of comfort with dashboards, and
statistics show that’s where engineers spend the most time — they provide data
in an easy-to-understand graphical user interface (GUI) for engineers to quickly
answer questions. However, it’s challenging when trace data is kept in its own
silo. To access its value, an engineer must navigate away from their primary
investigation to a separate place in the app — or worse, a separate app. Then
the engineer must try to recreate whatever context they had when they determined
that trace data could supplement the investigation. Over time, all but a few
power users start to drift away from using the trace query page on a regular
basis. Not because the trace query page is any less useful. It’s simply outside
of the average engineer’s scope. It’s like a kitchen appliance with lots of uses
when you’re cooking, but because it’s kept out of sight in the back of a drawer,
you never think to use it — even if it’s the best tool for the job.
We’re Still in the ‘Wild West’ When it Comes to Data Governance, StreamSets Says
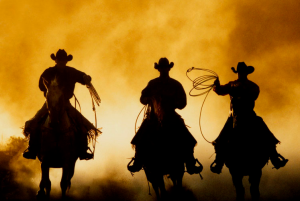
A lack of visibility into data pipelines raises the risk of other data security
problems, the company says. “The research reveals that 48% of businesses can’t
see when data is being used in multiple systems, and 40% cannot ensure data is
being pulled from the best source,” it says. “Moreover, 54% cannot integrate
pipelines with a data catalog, and 57% cannot integrate pipelines into a data
fabric.” Who holds responsibility for cleaning up the data mess? Well, that’s
another area with a bit of murkiness. About half (47%) of StreamSets survey
respondents say the centralized IT team bears responsibility for managing the
data. However, 18% said the line of business holds primary responsibility, while
it’s split between the business and IT in 35% of cases. A second survey released
by StreamSets last week highlights the difficulty in running data pipelines in
the modern enterprise. Many companies have thousands of data pipelines in use
and are hard pressed to build, manage, and maintain them at the pace required by
the business, according to StreamSets.
Quantum computing: What are the data storage challenges?
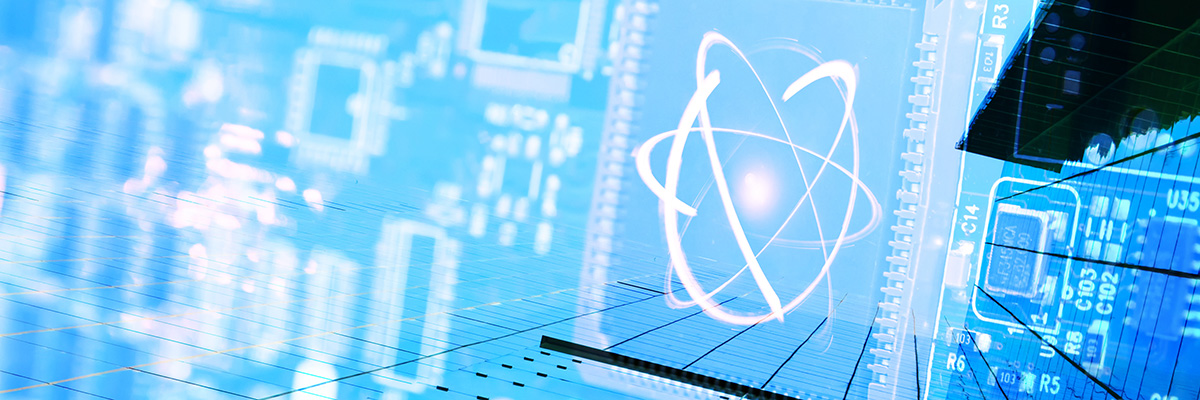
One of the core challenges of quantum computers is that their storage systems
are unsuitable for long-term storage due to quantum decoherence, the effect of
which can build up over time. Decoherence occurs when quantum computing data is
brought into existing data storage frameworks and causes qubits to lose their
quantum status, resulting in corrupted data and data loss. “Quantum mechanical
bits can’t be stored for long times as they tend to decay and collapse after a
while,” says Weides. “Depending on the technology used, they can collapse within
seconds, but the best ones are in a minute. You don’t really achieve 10 years of
storage. ...” Quantum computers will need data storage during computation, but
that needs to be a quantum memory for storing super-positioned or entangled
states, and storage durations are going to present a challenge. So, it’s likely
data storage for quantum computing will need to rely on conventional storage,
such as in high-performance computing (HPC). Considering the massive financial
investment required for quantum computing, to introduce a limitation of “cheap”
data storage elements as a cost-saving exercise would be counter-productive.
7 speed bumps on the road to AI
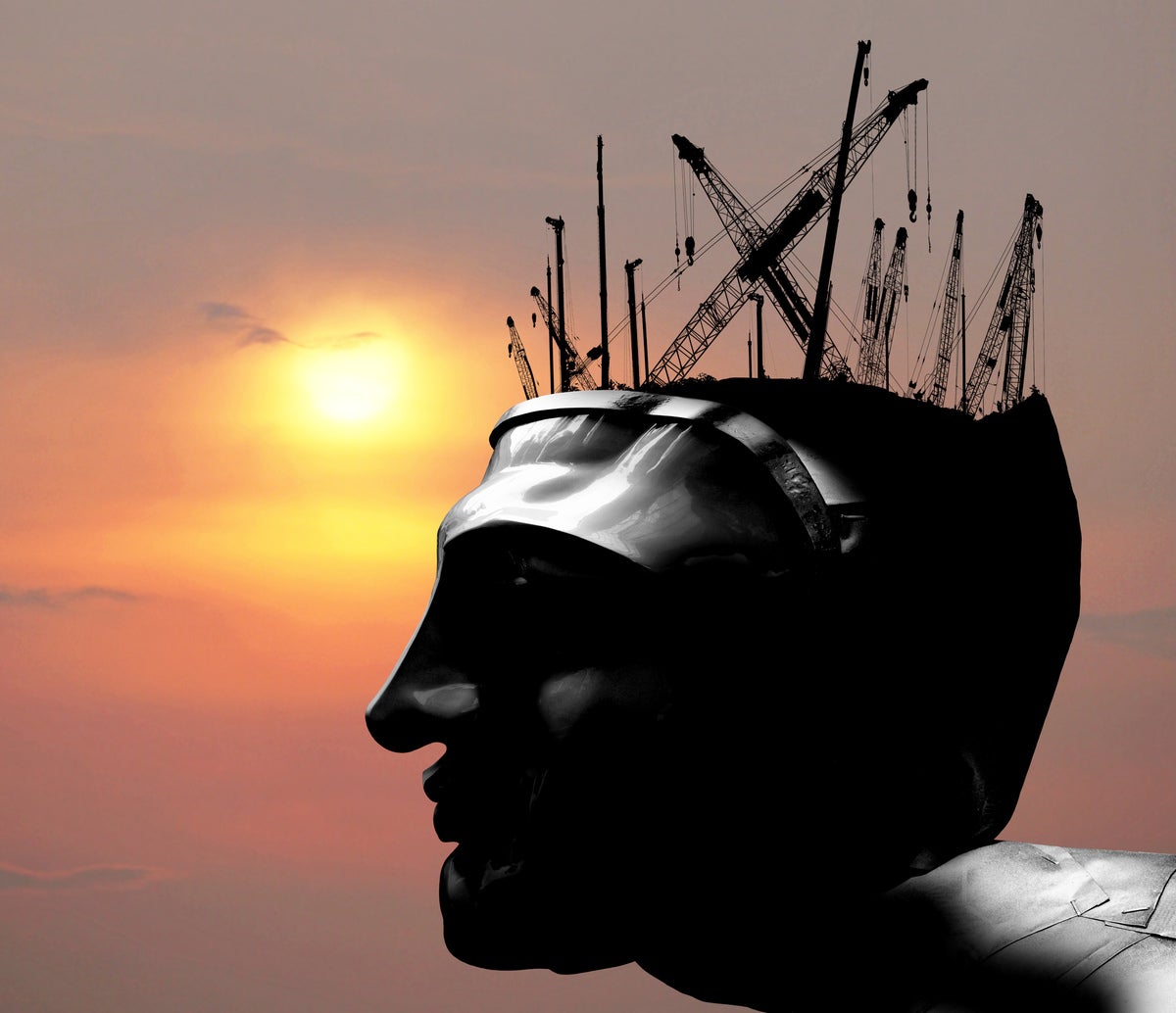
There are many issues and debates that humans know to avoid in certain contexts,
such as holiday dinners or the workplace. AIs, though, need to be taught how to
handle such issues in every context. Some large language models are programmed
to deflect loaded questions or just refuse to answer them, but some users simply
won't let a sleeping dog lie. When such a user notices the AI dodging a tricky
question, such as one that invokes racial or gender bias, they'll immediately
look for ways to get under those guardrails. Bias in data and insufficient data
are issues that can be corrected for over time, but in the meantime, the
potential for mischief and misuse is huge. And, while getting AI to churn out
hate speech is bad enough, the plot thickens considerably when we start using AI
to explore the moral implications of real life decisions. Many AI projects
depend on human feedback to guide their learning. Often, a project of scale
needs a high volume of people to build the training set and adjust the model’s
behavior as it grows. For many projects, the needed volume is only economically
feasible if trainers are paid low wages in poor countries.
7 ways to improve employee experience and workplace culture

The traditional hierarchical way of managing employees has been shown to be
largely ineffective. Companies run as adhocracies are more productive as they
foster knowledge sharing, workplace collaboration, and rapid adaptation—some of
the most important attributes for companies in the knowledge-based age. By
encouraging employees to be more self-sufficient and less dependent on their
superiors, you can promote greater efficiency and effectiveness in the
workplace. Start adopting more self-service options for employees. Modern IT and
HR systems can be calibrated to your employees’ needs and enable them to help
themselves, whether they want to book a vacation, access important documents,
get a better screen, or access an enterprise app. Although hybrid and remote
work seems to be the preferred model for many organizations, it still has
disadvantages. Many remote and hybrid employees struggle to manage the blurred
boundary between work and personal life, or the often less-than-ideal workplace
setups.
What Does a Strong Agile Culture Look Like?
A strong culture is critical for Agile organizations to be successful. Agile
requires organizations, and therefore its employees, to be ready to welcome
changing requirements and inspect and adapt at any given moment. Teams are
supposed to be self-managing and self-organizing. Stakeholders need to see
working products frequently. Breaking that down, expectations are that projects
change all the time but still need to be delivered in quick increments to
stakeholders, all the while teams are managing themselves. ... Psychological
safety in the workplace refers to the extent to which employees feel safe to
speak up, share their ideas, and take risks without fear of negative
consequences. It is the belief that one will not be punished or humiliated for
speaking up with ideas, questions, concerns, or mistakes. When there is
psychological safety in the workplace, employees are more likely to be engaged,
motivated, and productive. They are also more likely to collaborate, share their
knowledge and expertise, and contribute to innovation.
9 ways to avoid falling prey to AI washing

It’s not uncommon for a company to acquire dubious AI solutions, and in such
situations, the CIO may not necessarily be at fault. It could be “a symptom of
poor company leadership,” says Welch. “The business falls for marketing hype and
overrules the IT team, which is left to pick up the pieces.” To prevent moments
like these, organizations need to foster a collaborative culture in which the
opinion of tech professionals is valued and their arguments are listed
thoroughly. At the same time, CIOs and tech teams should build their reputation
within the company so their opinion is more easily incorporated into
decision-making processes. To achieve that, they should demonstrate expertise,
professionalism, and soft skills. “I don’t feel there’s a problem with detecting
AI washing for the CIO,” says Max Kovtun, chief innovation officer at Sigma
Software Group. “The bigger problem might be the push from business stakeholders
or entrepreneurs to use AI in any form because they want to look innovative and
cutting edge. So the right question would be how not to become an AI washer
under the pressure of entrepreneurship.”
Skilling up the security team for the AI-dominated era
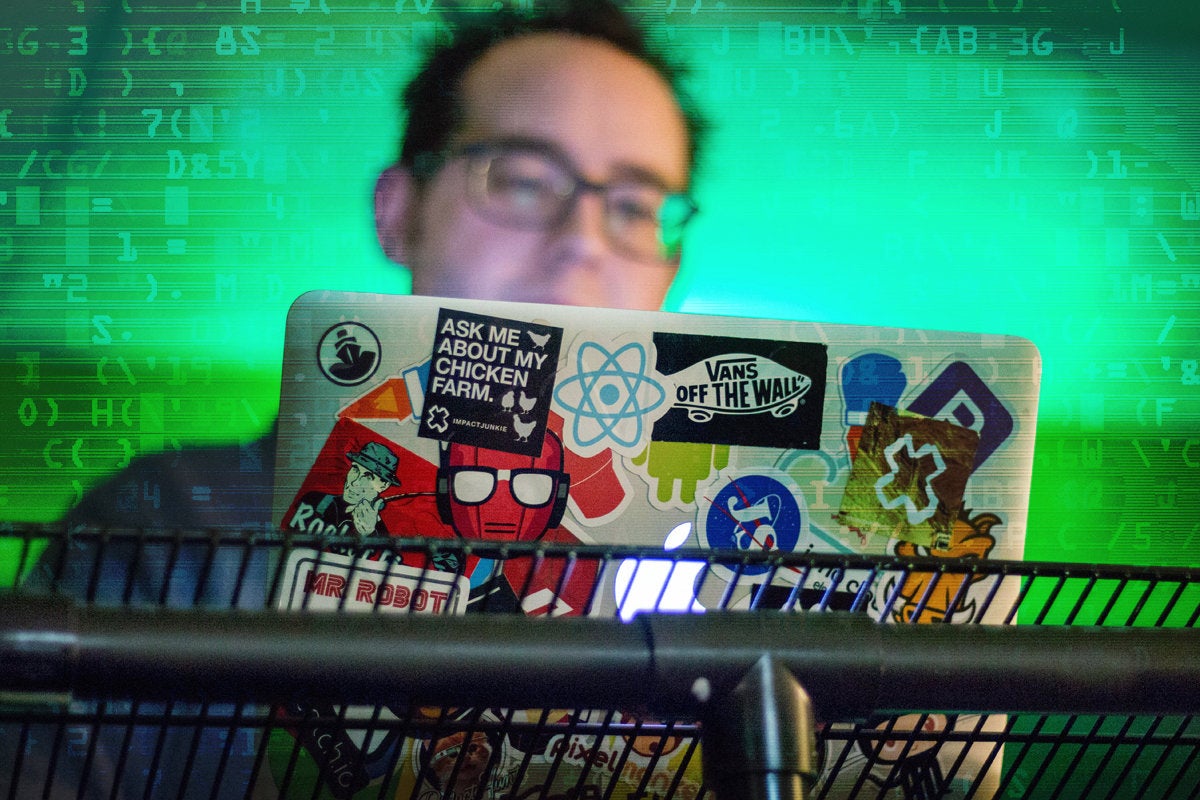
The increasing reliance of AI and machine learning models in all technological
walks of life is expected to rapidly change the complexion of the threat
landscape. Meanwhile, organically training security staff, bringing in AI
experts who can be trained to aid in security activities, and evangelizing the
hardening of AI systems will all take considerable runway. Experts share what
security leaders will need to shape their skill base and prepare to face both
sides of growing AI risk: risk to AI systems and risks from AI-based attacks.
There is some degree of crossover in each domain. For example, machine learning
and data science skills are going to be increasingly relevant on both sides. In
both cases existing security skills in penetration testing, threat modeling,
threat hunting, security engineering, and security awareness training will be as
important as ever, just in the context of new threats. However, the techniques
needed to defend against AI and to protect AI from attack also have their own
unique nuances, which will in turn influence the make-up of the teams called to
execute on those strategies.
Quote for the day:
"Remember teamwork begins by building
trust. And the only way to do that is to overcome our need for
invulnerability." -- Patrick Lencioni
No comments:
Post a Comment