Quote for the day:
"Create a compelling vision, one that takes people to a new place, and then translate that vision into a reality." -- Warren G. Bennis
The CIO Role Is Expanding -- And So Are the Risks of Getting It Wrong
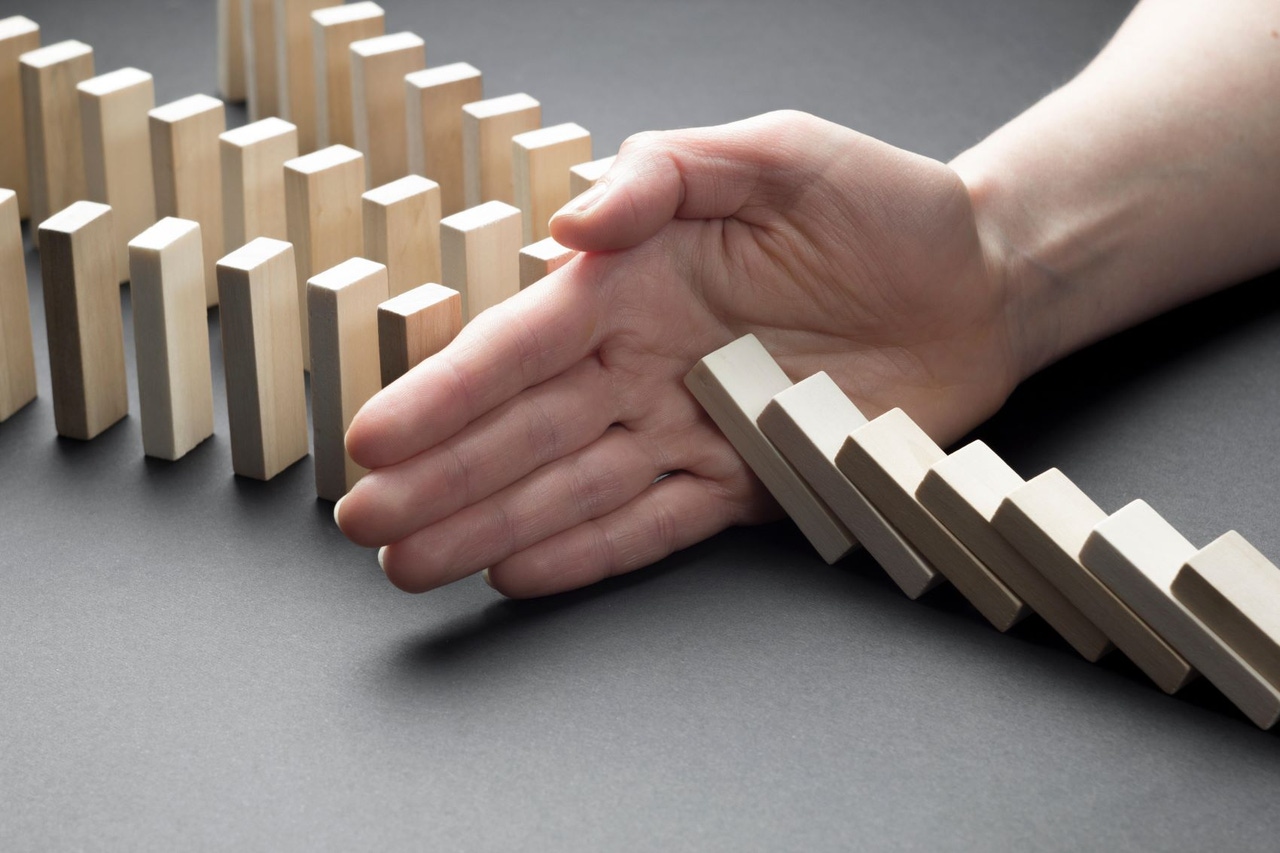
“We are seeing an increased focus of organizations giving CIOs more
responsibility to impact business strategy as well as tie it into revenue
growth,” says Sal DiFranco, managing partner of the global advanced technology
and CIO/CTO practices at DHR Global. He explains CIOs who are focused on
technology only for technology's sake and don’t have clear examples of business
strategy and impact are not being sought after. “While innovation experience is
important to have, it must come with a strong operational mindset,” DiFranco
says. ... He adds it is critical for CIOs to understand and articulate the
return on investment concerning technology investments. “Top CIOs have shifted
their thinking to a P&L mindset and act, speak, and communicate as the CEO
of the technology organization versus being a functional support group,” he
says. ... Gilbert says the greatest risk isn’t technical failure, it’s
leadership misalignment. “When incentives, timelines, or metrics don’t sync
across teams, even the strongest initiatives falter,” he explains. To counter
this, he works to align on a shared definition of value from day one, setting
clear, business-focused key performance indicators (KPIs), not just deployment
milestones. Structured governance helps, too: Transparent reporting,
cross-functional steering committees, and ongoing feedback loops keep everyone
on track.
How to Build a Lean AI Strategy with Data
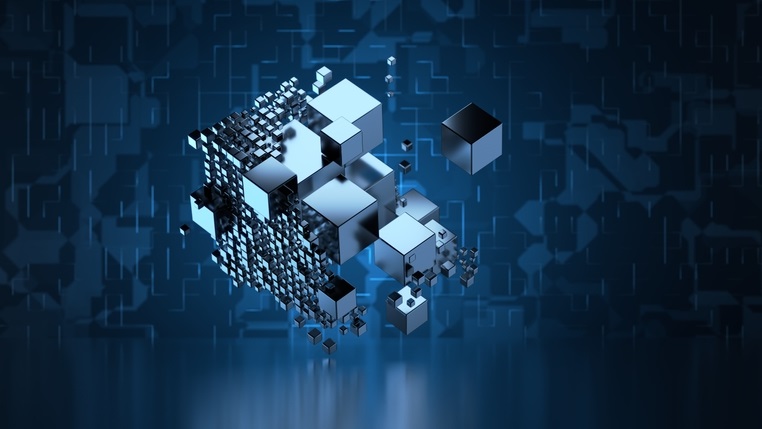
In simple terms, Lean AI means focusing on trusted, purpose-driven data to
power faster, smarter outcomes with AI—without the cost, complexity, and
sprawl that defines most enterprise AI initiatives today. Traditional
enterprise AI often chases scale for its own sake: more data, bigger models,
larger clouds. Lean AI flips that model—prioritizing quality over quantity,
outcomes over infrastructure, and agility over over-engineering. ... A lean AI
strategy focuses on curating high-quality, purpose-driven datasets tailored to
specific business goals. Rather than defaulting to massive data lakes,
organizations continuously collect data but prioritize which data to activate
and operationalize based on current needs. Lower-priority data can be archived
cost-effectively, minimizing unnecessary processing costs while preserving
flexibility for future use. ... Data governance plays a pivotal role in lean
AI strategies—but it should be reimagined. Traditional governance frameworks
often slow innovation by restricting access and flexibility. In contrast, lean
AI governance enhances usability and access while maintaining security and
compliance. ... Implementing lean AI requires a cultural shift in how
organizations manage data. Focusing on efficiency, purpose, and continuous
improvement can drive innovation without unnecessary costs or risks—a
particularly valuable approach when cost pressures are increasing.
Networking errors pose threat to data center reliability

“Data center operators are facing a growing number of external risks beyond
their control, including power grid constraints, extreme weather, network
provider failures, and third-party software issues. And despite a more
volatile risk landscape, improvements are occurring.” ... “Power has been the
leading cause. Power is going to be the leading cause for the foreseeable
future. And one should expect it because every piece of equipment in the data
center, whether it’s a facilities piece of equipment or an IT piece of
equipment, it needs power to operate. Power is pretty unforgiving,” said Chris
Brown, chief technical officer at Uptime Institute, during a webinar sharing
the report findings. “It’s fairly binary. From a practical standpoint of being
able to respond, it’s pretty much on or off.” ... Still, IT and networking
issues increased in 2024, according to Uptime Institute. The analysis
attributed the rise in outages due to increased IT and network complexity,
specifically, change management and misconfigurations. “Particularly with
distributed services, cloud services, we find that cascading failures often
occur when networking equipment is replicated across an entire network,”
Lawrence explained. “Sometimes the failure of one forces traffic to move in
one direction, overloading capacity at another data center.”
Unlocking ROI Through Sustainability: How Hybrid Multicloud Deployment Drives Business Value

One of the key advantages of hybrid multicloud is the ability to optimise
workload placement dynamically. Traditional on-premises infrastructure often
forces businesses to overprovision resources, leading to unnecessary energy
consumption and underutilisation. With a hybrid approach, workloads can
seamlessly move between on-prem, public cloud, and edge environments based on
real-time requirements. This flexibility enhances efficiency and helps
mitigate risks associated with cloud repatriation. Many organisations have
found that shifting back from public cloud to on-premises infrastructure is
sometimes necessary due to regulatory compliance, data sovereignty concerns,
or cost considerations. A hybrid multicloud strategy ensures organisations can
make these transitions smoothly without disrupting operations. ... With the
dynamic nature of cloud environments, enterprises really require solutions
that offer a unified view of their hybrid multicloud infrastructure.
Technologies that integrate AI-driven insights to optimise energy usage and
automate resource allocation are gaining traction. For example, some
organisations have addressed these challenges by adopting solutions such as
Nutanix Cloud Manager (NCM), which helps businesses track sustainability
metrics while maintaining operational efficiency.
'Lemon Sandstorm' Underscores Risks to Middle East Infrastructure
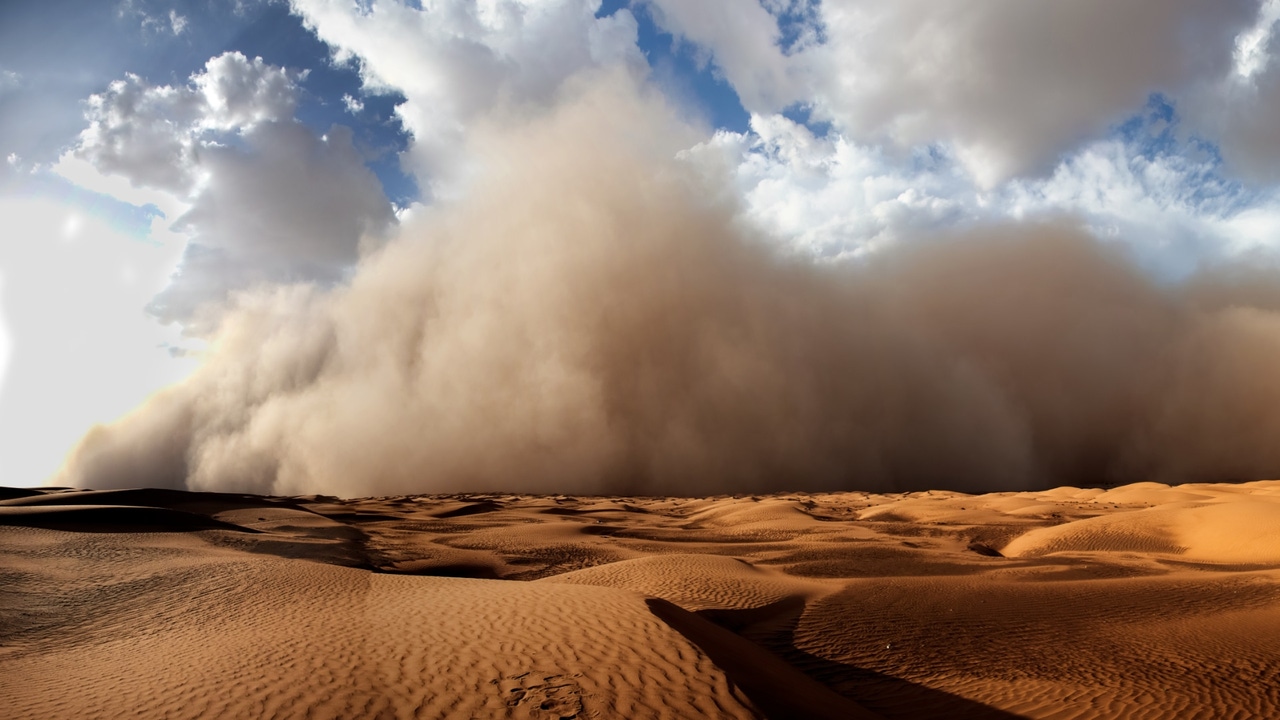
The compromise started at least two years ago, when the attackers used stolen
VPN credentials to gain access to the organization's network, according to a May
1 report published by cybersecurity firm Fortinet, which helped with the
remediation process that began late last year. Within a week, the attacker had
installed Web shells on two external-facing Microsoft Exchange servers and then
updated those backdoors to improve their ability to remain undetected. In the
following 20 months, the attackers added more functionality, installed
additional components to aid persistence, and deployed five custom attack tools.
The threat actors, which appear to be part of an Iran-linked group dubbed "Lemon
Sandstorm," did not seem focused on compromising data, says John Simmons,
regional lead for Fortinet's FortiGuard Incident Response team. "The threat
actor did not carry out significant data exfiltration, which suggests they were
primarily interested in maintaining long-term access to the OT environment," he
says. "We believe the implication is that they may [have been] positioning
themselves to carry out a future destructive attack against this CNI." Overall,
the attack follows a shift by cyber-threat groups in the region, which are now
increasingly targeting CNI.
Cloud repatriation hits its stride

Many enterprises are now confronting a stark reality. AI is expensive, not just
in terms of infrastructure and operations, but in the way it consumes entire IT
budgets. Training foundational models or running continuous inference pipelines
takes resources of an order of magnitude greater than the average SaaS or data
analytics workload. As competition in AI heats up, executives are asking tough
questions: Is every app in the cloud still worth its cost? Where can we redeploy
dollars to speed up our AI road map? ... Repatriation doesn’t signal the end of
cloud, but rather the evolution toward a more pragmatic, hybrid model. Cloud
will remain vital for elastic demand, rapid prototyping, and global scale—no
on-premises solution can beat cloud when workloads spike unpredictably. But for
the many applications whose requirements never change and whose performance is
stable year-round, the lure of lower-cost, self-operated infrastructure is too
compelling in a world where AI now absorbs so much of the IT spend. In this new
landscape, IT leaders must master workload placement, matching each application
to a technical requirement and a business and financial imperative.
Sophisticated cost management tools are on the rise, and the next wave of cloud
architects will be those as fluent in finance as they are in Kubernetes or
Terraform.
6 tips for tackling technical debt

Like most everything else in business today, debt can’t successfully be managed
if it’s not measured, Sharp says, adding that IT needs to get better at
identifying, tracking, and measuring tech debt. “IT always has a sense of where
the problems are, which closets have skeletons in them, but there’s often not a
formal analysis,” he says. “I think a structured approach to looking at this
could be an opportunity to think about things that weren’t considered
previously. So it’s not just knowing we have problems but knowing what the
issues are and understanding the impact. Visibility is really key.” ... Most
organizations have some governance around their software development programs,
Buniva says. But a good number of those governance programs are not as strong as
they should be nor detailed enough to inform how teams should balance speed with
quality — a fact that becomes more obvious with the increasing speed of
AI-enabled code production. ... Like legacy tech more broadly, code debt is a
fact of life and, as such, will never be completely paid down. So instead of
trying to get the balance to zero, IT exec Rishi Kaushal prioritizes fixing the
most problematic pieces — the ones that could cost his company the most. “You
don’t want to want to focus on fixing technical debt that takes a long time and
a lot of money to fix but doesn’t bring any value in fixing,” says Kaushal
AI Won’t Save You From Your Data Modeling Problems

Historically, data modeling was a business intelligence (BI) and analytics
concern, focused on structuring data for dashboards and reports. However, AI
applications shift this responsibility to the operational layer, where real-time
decisions are made. While foundation models are incredibly smart, they can also
be incredibly dumb. They have vast general knowledge but lack context and your
information. They need structured and unstructured data to provide this context,
or they risk hallucinating and producing unreliable outputs. ... Traditional
data models were built for specific systems, relational for transactions,
documents for flexibility and graphs for relationships. But AI requires all of
them at once because an AI agent might talk to the transactional database first
for enterprise application data, such as flight schedules from our previous
example. Then, based on that response, query a document to build a prompt that
uses a semantic web representation for flight-rescheduling logic. In this case,
a single model format isn’t enough. This is why polyglot data modeling is key.
It allows AI to work across structured and unstructured data in real time,
ensuring that both knowledge retrieval and decision-making are informed by a
complete view of business data.
Your password manager is under attack, and this new threat makes it worse

"Password managers are high-value targets and face constant attacks across
multiple surfaces, including cloud infrastructure, client devices, and browser
extensions," said NordPass PR manager Gintautas Degutis. "Attack vectors range
from credential stuffing and phishing to malware-based exfiltration and supply
chain risks." Googling the phrase "password manager hacked" yields a
distressingly long list of incursions. Fortunately, in most of those cases,
passwords and other sensitive information were sufficiently encrypted to limit
the damage. ... One of the most recent and terrifying threats to make headlines
came from SquareX, a company selling solutions that focus on the real-time
detection and mitigation of browser-based web attacks. SquareX spends a great
deal of its time obsessing over the degree to which browser extension
architectures represent a potential vector of attack for hackers. ... For
businesses and enterprises, the attack is predicated on one of two possible
scenarios. In the first scenario, users are left to make their own decisions
about what extensions are loaded onto their systems. In this case, they are
putting the entire enterprise at risk. In the second scenario, someone in an IT
role with the responsibility of managing the organization's approved browser and
extension configurations has to be asleep at the wheel.
Developing Software That Solves Real-World Problems – A Technologist’s View
Software architecture is not just a technical plan but a way to turn an idea
into reality. A good system can model users’ behaviors and usage, expand to meet
demand, secure data and combine well with other systems. It takes the concepts
of distributed systems, APIs, security layers and front-end interfaces into one
cohesive and easy-to-use product. I have been involved with building APIs that
are crucial for the integration of multiple products to provide a consistent
user experience to consumers of these products. Along with the group of
architects, we played a crucial role in breaking down these complex integrations
into manageable components and designing easy-to-implement API interfaces. Also,
using cloud services, these APIs were designed to be highly resilient. ... One
of the most important lessons I have learned as a technologist is that just
because we can build something does not mean we should. While working on a
project related to financing a car, we were able to collect personally
identifiable information (PII). Initially, we had it stored for a long duration.
However, we were unaware of the implications. When we discussed the situation
with the architecture and security teams, we found out that we don’t have
ownership of the data and it was very risky to store that data for a long
period. We mitigated the risk by reducing the data retention period to what will
be useful to users.
No comments:
Post a Comment