AI agents are coming to work — here’s what businesses need to know

Defining exactly what an agent is can be tricky, however: LLM-based agents are
an emerging technology, and there’s a level of variance in the sophistication of
tools labelled as “agents,” as well as how related terms are applied by vendors
and media. And as with the first wave of generative AI (genAI) tools, there are
question marks around how businesses will use the technology. ... With so many
tools in development or coming to the market, there’s a certain amount of
confusion among businesses that are struggling to keep pace. “The vendors are
announcing all of these different agents, and you can imagine what it’s like for
the buyers: instead of ‘The Russians are coming, the Russians are coming,’ it’s
‘the agents are coming, the agents are coming,’” said Loomis. “They’re being
bombarded by all of these new offerings, all of this new terminology, and all of
these promises of productivity.” Software vendors also offer varying
interpretations of the term “agent” at this stage, and tools coming to market
exhibit a broad spectrum of complexity and autonomy. ... Many of the agent
builder tools coming to business and work apps require little or no expertise.
This accessibility means a wide range of workers could manage and coordinate
their own agents.
The limits of AI-based deepfake detection
In terms of inference-based detection, ground truth is never known and assumed
as such, so detection is based on a one to ninety-nine percentage that the
content in question is or is not likely manipulated. Inference-based platform
needs no buy-in from platforms, but instead needs robust models trained on a
wide variety of deepfaking techniques and technologies in various use cases and
circumstances. To stay ahead of emerging threat vectors and groundbreaking new
models, those making an inference-based solution can look to emerging gen AI
research to implement such methods into detection models as or before such
research becomes productized. ... Greater public awareness and education will
always be of immense importance, especially in places where content is consumed
that could potentially be deepfaked or artificially manipulated. Yet deepfakes
are getting so convincing, so realistic that even storied researchers now have a
hard time differentiating real from fake simply by looking at or listening to a
media file. This is how advanced deepfakes have become, and they will only
continue to grow in believability and realism. This is why it is crucial to
implement deepfake detection solutions in the aforementioned content platforms
or anywhere deepfakes can and do exist.
Quantum error correction research yields unexpected quantum gravity insights

So far, scientists have not found a general way of differentiating trivial and
non-trivial AQEC codes. However, this blurry boundary motivated Liu, Daniel
Gottesman of the University of Maryland, US; Jinmin Yi of Canada’s Perimeter
Institute for Theoretical Physics; and Weicheng Ye at the University of British
Columbia, Canada, to develop a framework for doing so. To this end, the team
established a crucial parameter called subsystem variance. This parameter
describes the fluctuation of subsystems of states within the code space, and, as
the team discovered, links the effectiveness of AQEC codes to a property known
as quantum circuit complexity. ... The researchers also discovered that their
new AQEC theory carries implications beyond quantum computing. Notably, they
found that the dividing line between trivial and non-trivial AQEC codes also
arises as a universal “threshold” in other physical scenarios – suggesting that
this boundary is not arbitrary but rooted in elementary laws of nature. One such
scenario is the study of topological order in condensed matter physics.
Topologically ordered systems are described by entanglement conditions and their
associated code properties.
Towards greener data centers: A map for tech leaders
The transformation towards sustainability can be complex, involving key
decisions about data center infrastructure. Staying on-premises offers control
over infrastructure and data but poses questions about energy sourcing. Shifting
to hybrid or cloud models can leverage the innovations and efficiencies of
hyperscalers, particularly regarding power management and green energy
procurement. One of the most significant architectural advancements in this
context is hyperconverged infrastructure (HCI). As we know, traditionally data
centers operate using a three-tier architecture comprising separate servers,
storage, and network equipment. This model, though reliable, has clear
limitations in terms of energy consumption and cooling efficiency. By merging
the server and storage layers, HCI reduces both the power demands and the
associated cooling requirements. ... The drive to create more efficient and
environmentally conscious data centers is not just about cost control; it’s also
about meeting the expectations of regulators, customers, and stakeholders. As AI
and other compute-intensive technologies continue to proliferate, organizations
must reassess their infrastructure strategies, not just to meet sustainability
goals but to remain competitive.
What is a data architect? Skills, salaries, and how to become a data framework master

The data architect and data engineer roles are closely related. In some
ways, the data architect is an advanced data engineer. Data architects and
data engineers work together to visualize and build the enterprise data
management framework. The data architect is responsible to visualize the
blueprint of the complete framework that data engineers then build. ... Data
architect is an evolving role and there’s no industry-standard certification
or training program for data architects. Typically, data architects learn on
the job as data engineers, data scientists, or solutions architects, and
work their way to data architect with years of experience in data design,
data management, and data storage work. ... Data architects must have the
ability to design comprehensive data models that reflect complex business
scenarios. They must be proficient in conceptual, logical, and physical
model creation. This is the core skill of the data architect and the most
requested skill in data architect job descriptions. This often includes SQL
development and database administration. ... With regulations continuing to
evolve, data architects must ensure their organization’s data management
practices meet stringent legal and ethical standards. They need skills to
create frameworks that maintain data quality, security, and privacy.
AI – Implementing the Right Technology for the Right Use Case
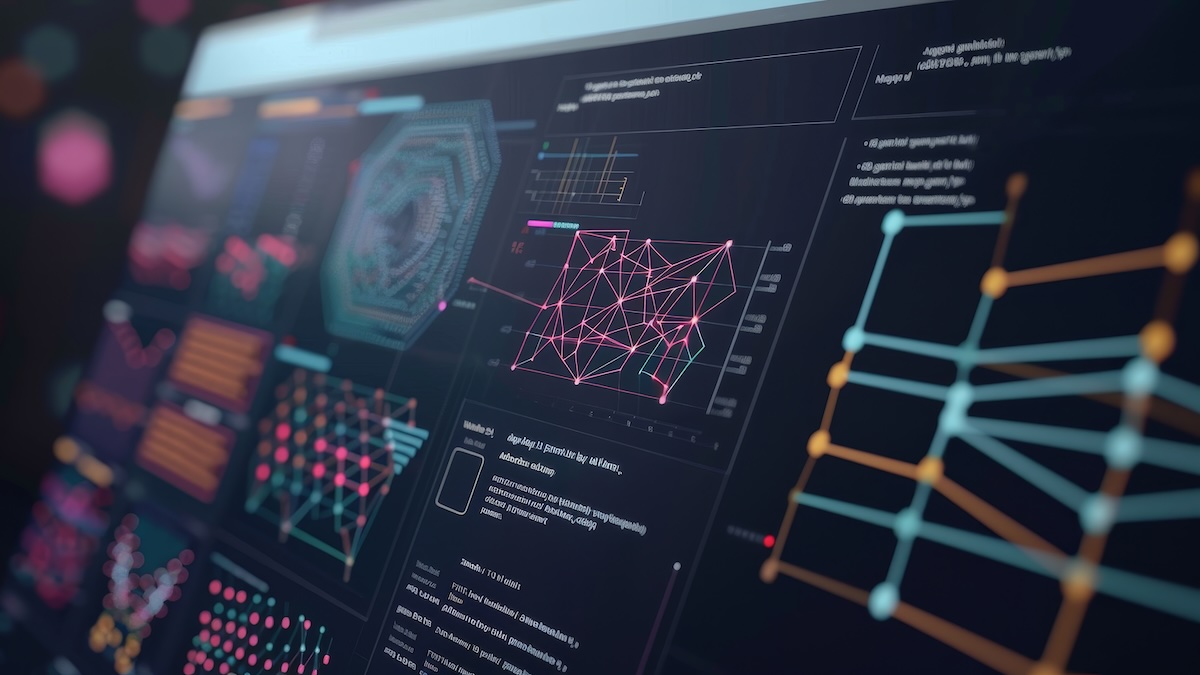
Right now, we very much see AI in this “peak of inflated expectations” phase
and predict that it will dip into the “trough of disillusionment”, where
organizations realize that it is not the silver bullet they thought it would
be. In fact, there are already signs of cynicism as decision-makers are
bombarded with marketing messages from vendors and struggle to discern what
is a genuine use case and what is not relevant for their organization. This
is a theme that also emerged as cybersecurity automation matured – the need
to identify the right use case for the technology, rather than try to apply
it across the board.. ... That said, AI is and will continue to be a useful
tool. In today’s economic climate, as businesses adapt to a new normal of
continuous change, AI—alongside automation—can be a scale function for
cybersecurity teams, enabling them to pivot and scale to defend against
evermore diverse attacks. In fact, our recent survey of 750 cybersecurity
professionals found that 58% of organizations are already using AI in
cybersecurity to some extent. However, we do anticipate that AI in
cybersecurity will pass through the same adoption cycle and challenges
experienced by “the cloud” and automation, including trust and technical
deployment issues, before it becomes truly productive.
A GRC framework for securing generative AI

Understanding the three broad categories of AI applications is just the
beginning. To effectively manage risk and governance, further classification
is essential. By evaluating key characteristics such as the provider,
hosting location, data flow, model type, and specificity, enterprises can
build a more nuanced approach to securing AI interactions. A crucial factor
in this deeper classification is the provider of the AI model. ... As AI
technology advances, it brings both transformative opportunities and
unprecedented risks. For enterprises, the challenge is no longer whether to
adopt AI, but how to govern AI responsibly, balancing innovation against
security, privacy, and regulatory compliance. By systematically categorizing
generative AI applications—evaluating the provider, hosting environment,
data flow, and industry specificity—organizations can build a tailored
governance framework that strengthens their defenses against AI-related
vulnerabilities. This structured approach enables enterprises to anticipate
risks, enforce robust access controls, protect sensitive data, and maintain
regulatory compliance across global jurisdictions. The future of enterprise
AI is about more than just deploying the latest models; it’s about embedding
AI governance deeply into the fabric of the organization.
Business Continuity Depends on the Intersection of Security and Resilience
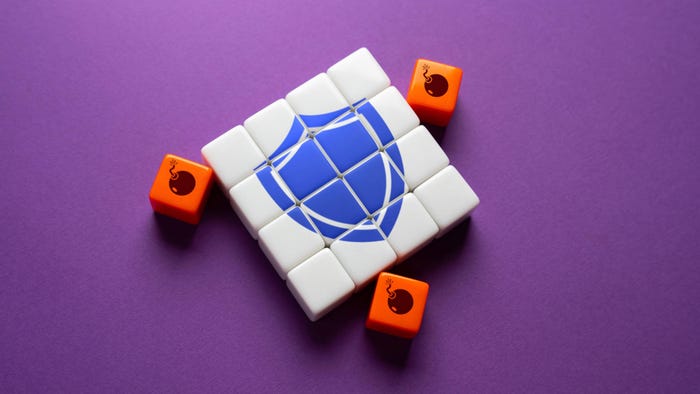
The focus of security, or the goal of security, or the intended purpose of
security in its most natural and traditional form, right before we start to
apply it to other things, is to prevent bad things from happening, or
protect the organization or protect assets. It doesn't necessarily have to
be technology that does it. This is where your policies and procedures come
into place. Letting users know what acceptable use policies are or what
things are accepted when leveraging corporate resources. From a technology
perspective, it's your firewalls, antivirus, intrusion detection systems and
things of that nature. So, this is where we focus on good cyber hygiene.
We're controlling the controllables and making sure that we're taking care
of the things that are within our control. What about resilience? This one
is near and dear to my heart. That's because I've been in tech and security
for almost 25 years, and I've kind of gone through this evolution of what I
think is important. We're trained as practitioners in this industry to
believe that the goal is to reduce risk. We must reduce or mitigate cyber
risk, or we can make other risk decisions. We can avoid it, we can accept
it, or we can transfer it. But practically speaking, when we show up to work
every day and we're doing something active, we're reducing risk.
How to stop data mesh turning into a data mess
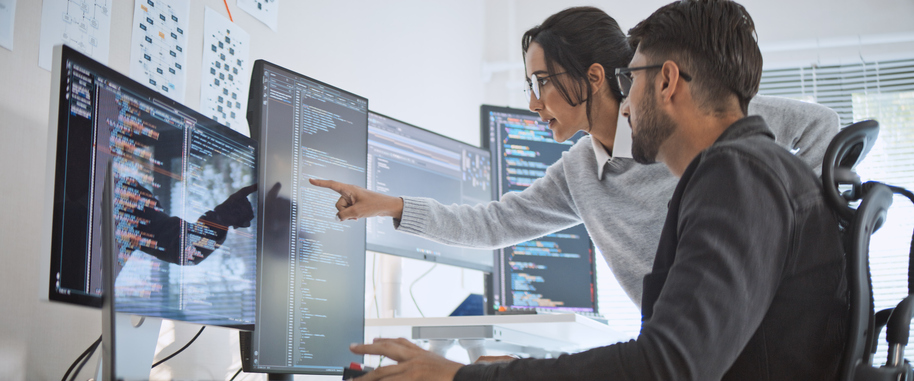
Realistically, expecting employees to remember to follow data quality and
compliance guidelines is neither fair nor enforceable. Adherence must be
implemented without frustrating users, and become an integral part of the
project delivery process. Unlikely as this sounds, a computational
governance platform can impose the necessary standards as ‘guardrails’ while
also accelerating the time to market of products. Sitting above an
organisation’s existing range of data enablement and management tools, a
computational governance platform ensures every project follows
pre-determined policies, for quality, compliance, security, and
architecture. Highly customisable standards can be set at global or local
levels, whatever is required. ... While this might seem restrictive, there
are many benefits from having a standardised way of working. To streamline
processes, intelligent automated templates help data practitioners quickly
initiate new projects and search for relevant data. The platform can oversee
the deployment of data products by checking their compliance and taking care
of the resource provisioning, freeing the teams from the burden of coping
with infrastructure technicalities (on cloud or on-prem) and certifying data
product compliance at the same time, before data products enter
production.
The SEC Fines Four SolarWinds Breach Victims
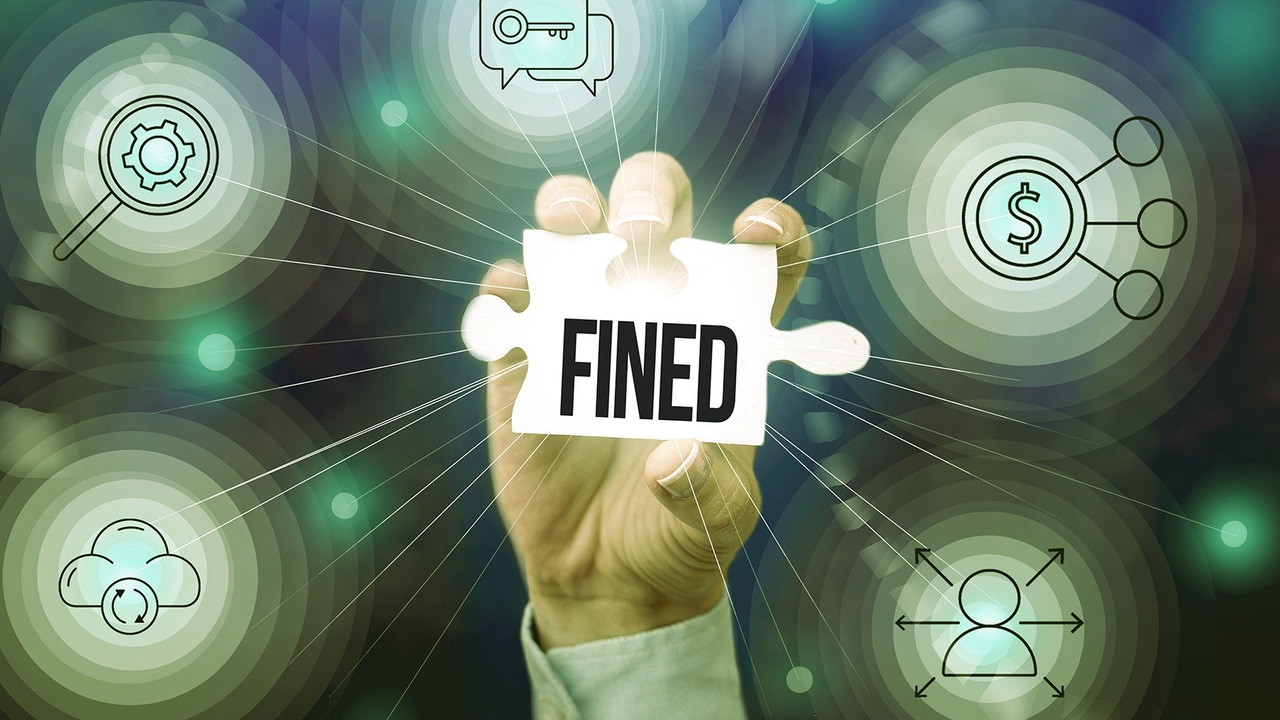
Companies should ensure the cyber and data security information they share
within their organizations is consistent with what they share with
government agencies, shareholders and the public, according to Buchanan
Ingersoll & Rooney’s Sanger. This applies to their security posture
prior to a breach, as well as their responses afterward. “Consistent
messaging is difficult to manage given that dozens, hundreds or thousands
could be responsible for an organization’s cybersecurity. Investigators will
always be able to find a dissenting or more pessimistic outlook among the
voices involved,” says Sanger. “If there is a credible argument that
circumstances are or were worse than what the organization shares publicly,
leadership should openly acknowledge it and take steps to justify the
official perspective.” Corporate cybersecurity breach reporting is still
relatively uncharted territory, however. “Even business leaders who intend
to act with complete transparency can make inadvertent mistakes or
communicate poorly, particularly because the language used to discuss
cybersecurity is still developing and differs between communities,” says
Sanger. “It’s noteworthy that the SEC framed each penalized company as
having, ‘negligently minimized its cybersecurity incident in its public
disclosures.’
Quote for the day:
"Perfection is not attainable, but
if we chase perfection we can catch excellence." --
Vince Lombardi
No comments:
Post a Comment