Enter the ‘Whisperverse’: How AI voice agents will guide us through our days

Within the next few years, an AI-powered voice will burrow into your ears and
take up residence inside your head. It will do this by whispering guidance to
you throughout your day, reminding you to pick up your dry cleaning as you
walk down the street, helping you find your parked car in a stadium lot and
prompting you with the name of a coworker you pass in the hall. It may even
coach you as you hold conversations with friends and coworkers, or when out on
dates, give you interesting things to say that make you seem smarter, funnier
and more charming than you really are. ... Most of these devices will be
deployed as AI-powered glasses because that form-factor gives the best vantage
point for cameras to monitor our field of view, although camera-enabled
earbuds will be available too. The other benefit of glasses is that they can
be enhanced to display visual content, enabling the AI to provide silent
assistance as text, images, and realistic immersive elements that are
integrated spatially into our world. Also, sensored glasses and earbuds will
allow us to respond silently to our AI assistants with simple head nod
gestures of agreement or rejection, as we naturally do with other people. ...
On the other hand, deploying intelligent systems that whisper in your ears as
you go about your life could easily be abused as a dangerous form of targeted
influence.
How to Optimize Last-Mile Delivery in the Age of AI
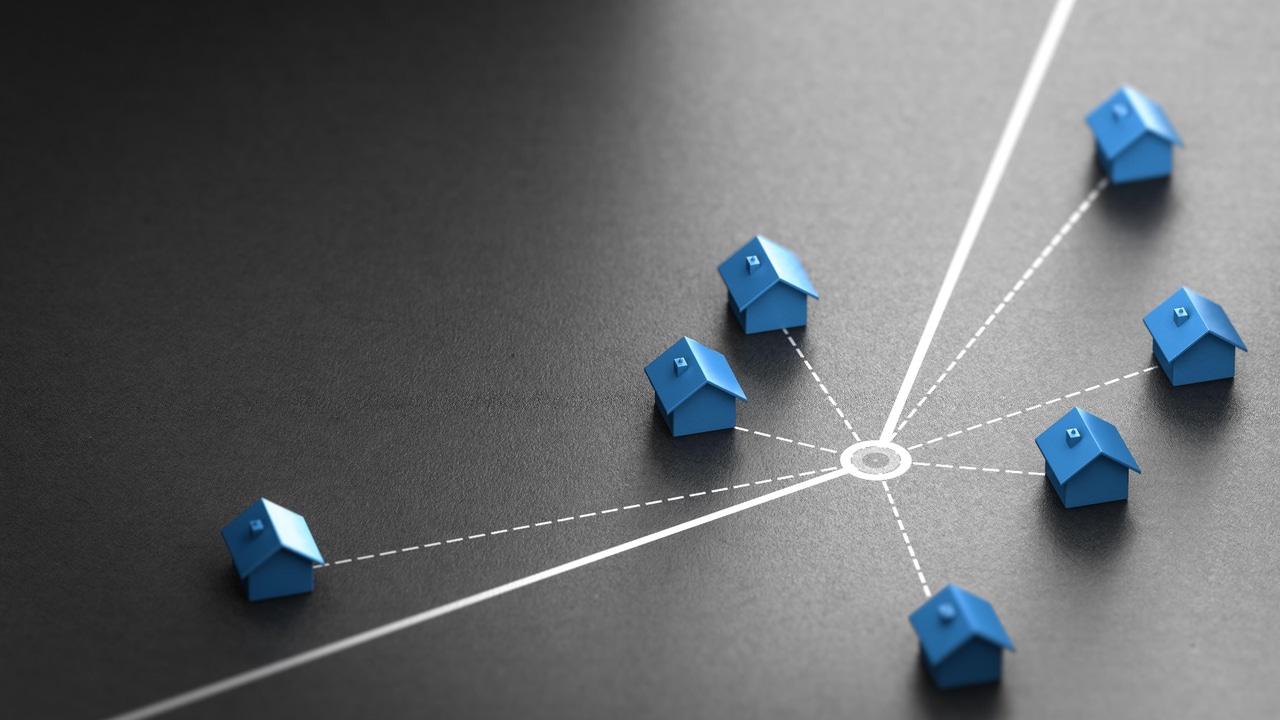
Technology is at the heart of all advancements in last-mile delivery. For
instance, a typical map application gives the longitude and latitude of a
building — its location — and a central access point. That isn't enough data
when it comes to deliveries. In addition to how much time it takes to drive or
walk from point A to point B, it's also essential for a driver to understand
what to do at point B. At an apartment complex, for example, they need to know
what units are in each building and on which level, whether to use a front,
back, or side entrance, how to navigate restricted or gated areas, and how to
access parking and loading docks or package lockers. Before GenAI, third-party
vendors usually acquired this data, sold it to companies, and applied it to
map applications and routing algorithms to provide delivery estimates and
instructions. Now, companies can use GenAI in-house to optimize routes and
create solutions to delivery obstacles. Suppose the data surrounding an
apartment complex is ambiguous or unclear. For instance, there may be
conflicting delivery instructions — one transporter used a drop-off area, and
another used a front door. Or perhaps one customer was satisfied with their
delivery, but another parcel delivered to the same location was damaged or
stolen.
Cloud providers make bank with genAI while projects fail

Poor data quality is a central factor contributing to project failures. As
companies venture into more complex AI applications, the demand for tailored,
high-quality data sets has exposed deficiencies in existing enterprise data.
Although most enterprises understood that their data could have been better,
they haven’t known how bad. For years, enterprises have been kicking the data
can down the road, unwilling to fix it, while technical debt gathered. AI
requires excellent, accurate data that many enterprises don’t have—at least,
not without putting in a great deal of work. This is why many enterprises are
giving up on generative AI. The data problems are too expensive to fix, and
many CIOs who know what’s good for their careers don’t want to take it on. The
intricacies in labeling, cleaning, and updating data to maintain its relevance
for training models have become increasingly challenging, underscoring another
layer of complexity that organizations must navigate. ... The disparity
between the potential and practicality of generative AI projects is leading to
cautious optimism and reevaluations of AI strategies. This pushes
organizations to carefully assess the foundational elements necessary for AI
success, including robust data governance and strategic planning—all things
that enterprises are considering too expensive and too risky to deploy just to
make AI work.
Why cybersecurity needs a better model for handling OSS vulnerabilities

Identifying vulnerabilities and navigating vulnerability databases is of
course only part of the dependency problem; the real work lies in remediating
identified vulnerabilities impacting systems and software. Aside from general
bandwidth challenges and competing priorities among development teams,
vulnerability management also suffers from challenges around remediation, such
as the real potential that implementing changes and updates can potentially
impact functionality or cause business disruptions. ... Reachability analysis
“offers a significant reduction in remediation costs because it lowers the
number of remediation activities by an average of 90.5% (with a range of
approximately 76–94%), making it by far the most valuable single
noise-reduction strategy available,” according to the Endor report. While the
security industry can beat the secure-by-design drum until they’re blue in the
face and try to shame organizations into sufficiently prioritizing security,
the reality is that our best bet is having organizations focus on risks that
actually matter. ... In a world of competing interests, with organizations
rightfully focused on business priorities such as speed to market, feature
velocity, revenue and more, having developers quit wasting time and focus on
the 2% of vulnerabilities that truly present risks to their organizations
would be monumental.
The new calling of CIOs: Be the moral arbiter of change

Unfortunately, establishing a strategy for democratizing innovation through
gen AI is far from straightforward. Many factors, including governance,
security, ethics, and funding, are important, and it’s hard to establish
ground rules. ... What’s clear is tech-led innovation is no longer the sole
preserve of the IT department. Fifteen years ago, IT was often a solution
searching for a problem. CIOs bought technology systems, and the rest of the
business was expected to put them to good use. Today, CIOs and their teams
speak with their peers about their key challenges and suggest potential
solutions. But gen AI, like cloud computing before it, has also made it much
easier for users to source digital solutions independently of the IT team.
That high level of democratization doesn’t come without risks, and that’s
where CIOs, as the guardians of enterprise technology, play a crucial role. IT
leaders understand the pain points around governance, implementation, and
security. Their awareness means responsibility for AI, and other emerging
technologies have become part of a digital leader’s ever-widening role, says
Rahul Todkar, head of data and AI at travel specialist Tripadvisor.
5 Strategies For Becoming A Purpose-Driven Leader

Purpose-driven leaders are fueled by more than sheer ambition; they are driven
by a commitment to make a meaningful impact. They inspire those around them to
pursue a shared purpose each day. This approach is especially powerful in
today’s workforce, where 70% of employees say their sense of purpose is
closely tied to their work, according to a recent report by McKinsey. Becoming
a purpose-driven leader requires clarity, strategic foresight, and a
commitment to values that go beyond the bottom line. ... Aligning your values
with your leadership style and organizational goals is essential for authentic
leadership. “Once you have a firm grasp of your personal values, you can align
them with your leadership style and organizational goals. This alignment is
crucial for maintaining authenticity and ensuring that your decisions reflect
your deeper sense of purpose,” Blackburn explains. ... Purpose-driven leaders
embody the values and behaviors they wish to see reflected in their teams.
Whether through ethical decision-making, transparency, or resilience in the
face of challenges, purpose-driven leaders set the tone for how others in the
organization should act. By aligning words with actions, leaders build
credibility and trust, which are the foundations of sustainable success.
Chaos Engineering: The key to building resilient systems for seamless operations
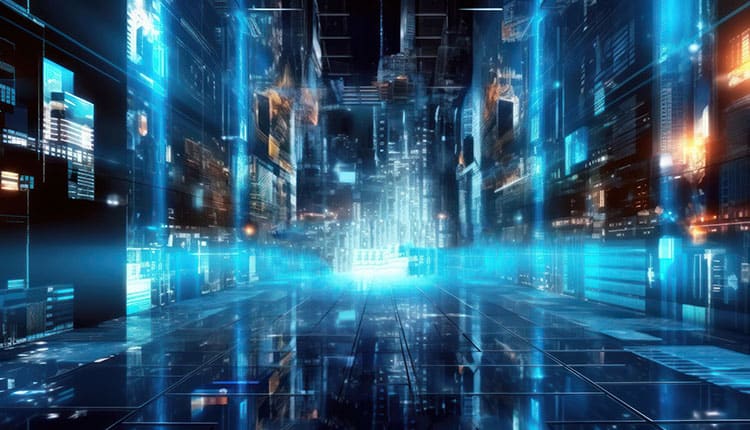
The underlying philosophy of Chaos Engineering is to encourage building
systems that are resilient to failures. This means incorporating redundancy
into system pathways, so that the failure of one path does not disrupt the
entire service. Additionally, self-healing mechanisms can be developed such as
automated systems that detect and respond to failures without the need for
human intervention. These measures help ensure that systems can recover
quickly from failures, reducing the likelihood of long-lasting disruptions. To
effectively implement Chaos Engineering and avoid incidents like the payments
outage, organisations can start by formulating hypotheses about potential
system weaknesses and failure points. They can then design chaos experiments
that safely simulate these failures in controlled environments. Tools such as
Chaos Monkey, Gremlin, or Litmus can automate the process of failure injection
and monitoring, enabling engineers to observe system behaviour in response to
simulated disruptions. By collecting and analysing data from these
experiments, organisations can learn from the failures and use these insights
to improve system resilience. This process should be iterative, and
organisations should continuously run new experiments and refine their systems
based on the results.
Shifting left with telemetry pipelines: The future of data tiering at petabyte scale
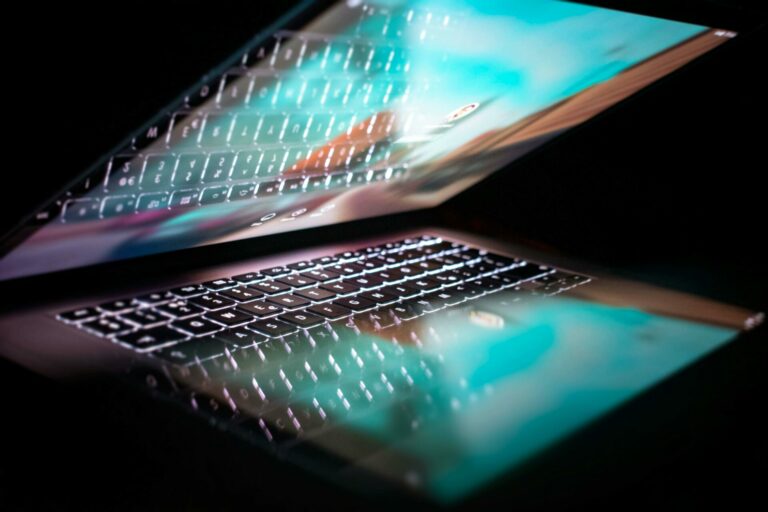
In the context of observability and security, shifting left means
accomplishing the analysis, transformation, and routing of logs, metrics,
traces, and events very far upstream, extremely early in their usage lifecycle
— a very different approach in comparison to the traditional “centralize then
analyze” method. By integrating these processes earlier, teams can not only
drastically reduce costs for otherwise prohibitive data volumes, but can even
detect anomalies, performance issues, and potential security threats much
quicker, before they become major problems in production. The rise of
microservices and Kubernetes architectures has specifically accelerated this
need, as the complexity and distributed nature of cloud-native applications
demand more granular and real-time insights, and each localized data set is
distributed when compared to the monoliths of the past. ... As telemetry data
continues to grow at an exponential rate, enterprises face the challenge of
managing costs without compromising on the insights they need in real time, or
the requirement of data retention for audit, compliance, or forensic security
investigations. This is where data tiering comes in. Data tiering is a
strategy that segments data into different levels based on its value and use
case, enabling organizations to optimize both cost and performance.
A Transformative Journey: Powering the Future with Data, AI, and Collaboration
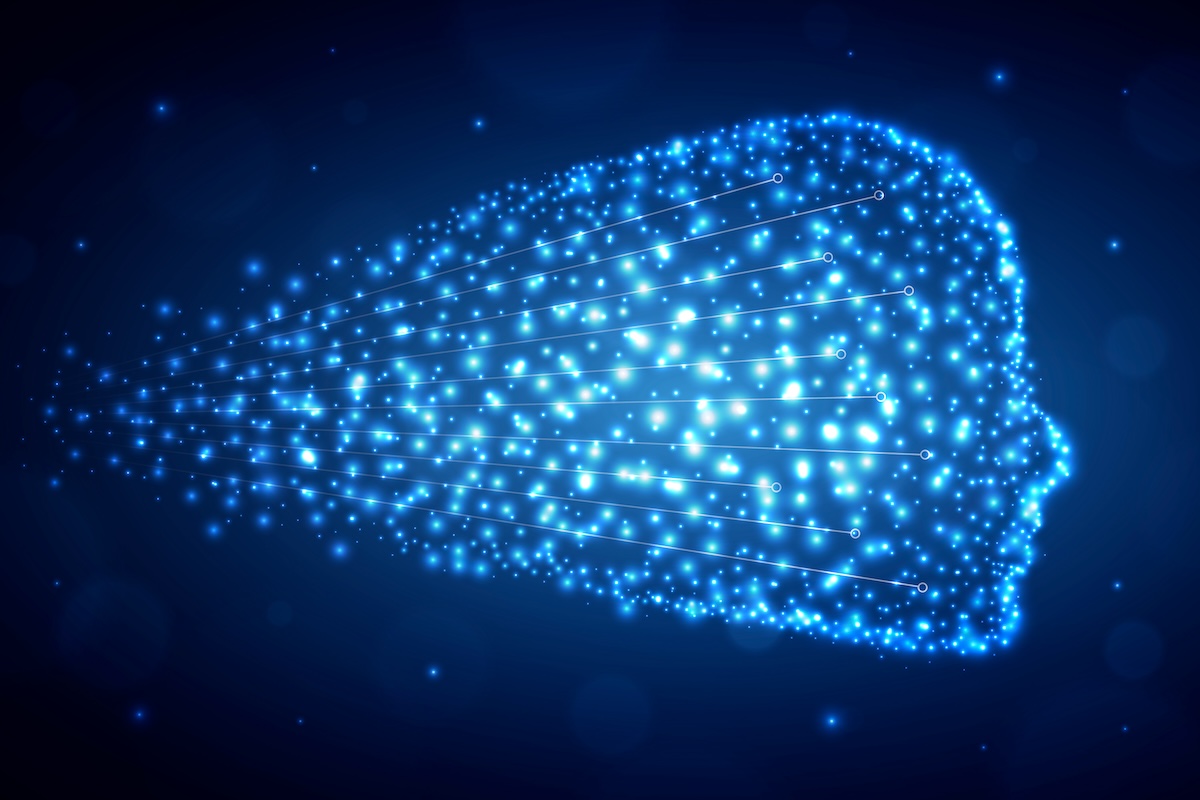
The advancements in industrial data platforms and contextualization have been
nothing short of remarkable. By making sense of data from different
systems—whether through 3D models, images, or engineering diagrams—Cognite is
enabling companies to build a powerful industrial knowledge graph, which can
be used by AI to solve complex problems faster and more effectively than ever
before. This new era of human-centric AI is not about replacing humans but
enhancing their capabilities, giving them the tools to make better decisions,
faster. Without the buy in from the people who will be affected by any new
innovation or technology the probability of success is unlikely. Engaging
these individuals early on in the process to solve the issues they find
challenging, mundane, or highly repetitive, is critical to driving adoption
and creating internal champions to further catalyze adoption. In a fascinating
case study shared by one of Cognite’s partners, we learned about the
transformative potential of data and AI in the chemical manufacturing sector.
A plant operator described how the implementation of mobile devices powered by
Cognite’s platform has drastically improved operational efficiency.
Four Steps to Balance Agility and Security in DevSecOps

Tools like OWASP ZAP and Burp Suite can be integrated into continuous
integration/continuous delivery (CI/CD) pipelines to automate security
testing. For example, LinkedIn uses Ansible to automate its infrastructure
provisioning, which reduces deployment times by 75%. By automating security
checks, LinkedIn ensures that its rapid delivery processes remain secure.
Automating security not only enhances speed but also improves the overall
quality of software by catching issues before they reach production. Automated
tools can perform static code analysis, vulnerability scanning and penetration
testing without disrupting the development cycle, helping teams deploy secure
software faster. ... As organizations look to the future, artificial
intelligence (AI) and machine learning (ML) will play a crucial role in
enhancing both security and agility. AI-driven security tools can predict
potential vulnerabilities, automate incident response and even self-heal
systems without human intervention. This not only improves security but also
reduces the time spent on manual security reviews. AI-powered tools can
analyze massive amounts of data, identifying patterns and potential threats
that human teams may overlook. This can reduce downtime and the risk of
cyberattacks, ultimately allowing organizations to deploy faster and more
securely.
Quote for the day:
"If you are truly a leader, you will
help others to not just see themselves as they are, but also what they can
become." -- David P. Schloss
No comments:
Post a Comment