How generative AI is redefining data analytics
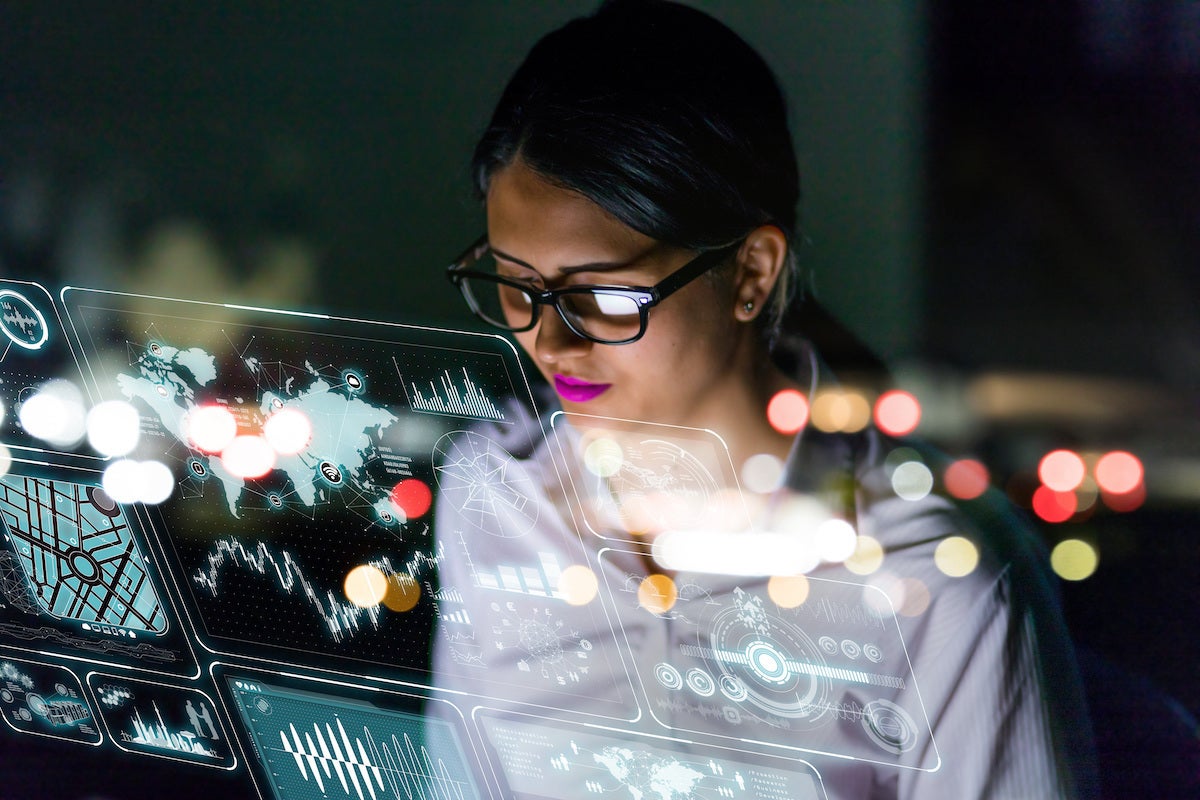
When applied to analytics, generative AI:Streamlines the foundational data
stages of ELT: Predictive algorithms are applied to optimize data extraction,
intelligently organize data during loading, and transform data with automated
schema recognition and normalization techniques. Accelerates data preparation
through enrichment and data quality: AI algorithms predict and fill in missing
values, identify and integrate external data sources to enrich the data set,
while advanced pattern recognition and anomaly detection ensure data accuracy
and consistency. Enhances analysis of data, such as geospatial and autoML:
Mapping and spatial analysis through AI-generated models enable accurate
interpretation of geographical data, while automated selection, tuning, and
validation of machine learning models increase the efficiency and accuracy of
predictive analytics. Elevates the final stage of analytics, reporting: Custom,
generative AI-powered applications provide interactive data visualizations and
analytics tailored to specific business needs.
Open-source or closed-source AI? Data quality and adaptability matter more

Licensing and usage terms of services matter in that they dictate how you use a
particular model — and even what you use it for. Even so, getting caught up in
the closed vs. open zealotry is shortsighted at a time when 70% of CEOs surveyed
expect gen AI to significantly alter the way their companies create, deliver and
capture value over the next three years, according to PwC. Rather, you should
focus on the quality of your data. After all, data will be your competitive
differentiator — not the model. ... Experimenting with different model types and
sizes to suit your use cases is a critical part of the trial-and-error process.
Right-sizing, or deploying the most appropriate model sizes for your business,
is more crucial. Do you require a broad, boil-the-ocean approach that spans as
much data as possible to build a digital assistant with encyclopedic knowledge?
A large LLM cultivating hundreds of billions of data points may work well. ...
Of course, the gen AI model landscape is ever evolving. Future models will look
and function differently than those of today. Regardless of your choices, with
the right partner you can turn your data ocean into a wellspring of insights.
Tips for Building a Platform Engineering Discipline That Lasts

A great platform engineer is defined both by their ability to create
infrastructure and advocate for and guide others (which is where communication
skills come in) — especially in the platforms that are maturing today. As far as
hard skills go, the platform engineer should have experience in cloud platforms,
CI/CD, IaC, security, and automation. Other roles you’ll need include a product
owner to manage platform stakeholders and track KPIs. Our 2024 State of DevOps
report found that 70% of respondents said a product manager was important to the
platform team – 52% of whom called the role “critical”. To avoid complexity and
scaling issues, you’ll also need architects with the vision and skills to help
the platform engineering team design and build it. Infrastructure as code
(IaC) is version control for your infrastructure. It makes infrastructure
human-readable, auditable, repeatable, scalable, and securable. IaC also lets
disparate teams — developers, operations, and QA — review, collaborate, iterate,
and maintain infrastructure code simultaneously.
What Is the American Privacy Rights Act, and Who Supports It?
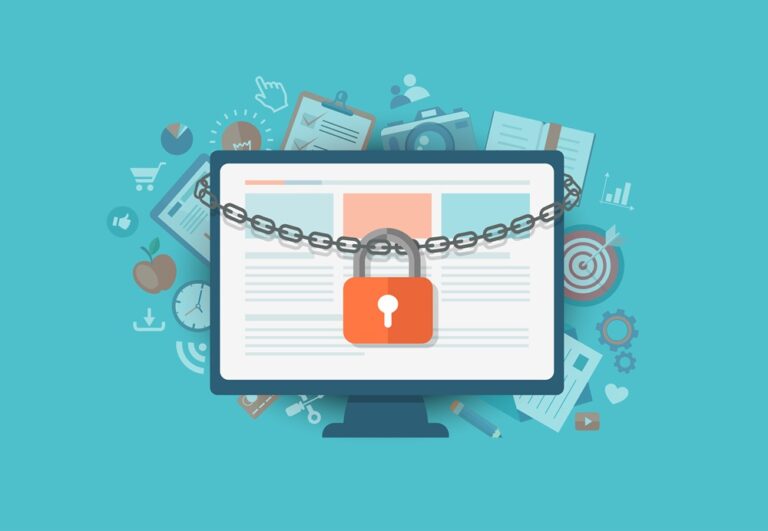
The APRA ostensibly is about data, but AI is also covered a bit. Companies must
evaluate their “covered algorithms” before deploying it and provide that
evaluation to the FTC and the public. Companies must also adhere to people’s
request to opt out of the use of any algorithm related to housing, employment,
education, health care, insurance, credit, or access to places of public
accommodation. The APRA would be enforced by a new bureau operating under the
Federal Trade Commission (FTC). State attorneys general would also be able to
enforce the new law. It would also allow individuals to file private lawsuits
against companies that violate the law. There are several important exceptions
in the APRA. For instance, small businesses, defined as having less than $40
million in annual revenue or collecting data on 200,000 or fewer individuals (as
long as they’re not in the data-selling business themselves), are exempt from
the APRA’s requirements. Governmental agencies and organizations working for
them are also exempt, in addition to non-profit organizations whose main purpose
is fraud-fighting, as well.
Empowering Users: Embracing Product-Centric Strategies in SaaS
/dq/media/media_files/rxBPNl6RNRHwY9SBCNMW.jpg)
A non-negotiable requirement for a SaaS product to succeed with a
product-centric strategy is for it to be intuitively designed with minimal
friction and a focus on delivering value as quickly as possible. This is not a
set-and-forget task demanding a profound understanding of the critical user
journey and ruthlessly prioritizing friction and pain point elimination instead
of just plastering feature promotions through in- and out-of-product
interventions. However, this cannot be done if teams don’t use data analytics or
prioritize the voice of the customer through feedback loops to further product
development and work towards building a loved and delightful product. A great
example of a PLG pioneer is Figma. ... On the other hand, adopting a
product-led growth approach requires fundamental organizational shifts. The
success of PLG requires a combined, multidisciplinary team dedicated to
continuous improvement and adaptation of the product to support both new
customer acquisition as well as retention and growth.
6 tips to implement security gamification effectively
Gamification leverages elements of traditional gaming, online and offline, to
boost engagement and investment in the learning process. Points, badges, and
leaderboards reward successful actions, fostering a sense of achievement and
friendly competition. Engaging scenarios and challenges simulate real-world
threats, allowing trainees to apply knowledge practically. Difficulty levels
keep learners engaged, while immediate feedback on decisions solidifies learning
and highlights areas for improvement. Effective implementation hinges on
transparency, simplicity, and a level playing field. A central dashboard that
displays the same security data for everyone keeps things simple, fostering a
shared understanding of progress. ... Personalized challenges help ensure
engagement. New security teams might focus on mastering foundational tasks like
vulnerability scans, while seasoned teams tackle advanced challenges like
reducing time for response to critical security events. This keeps everyone
motivated and learning, while offering continuous improvement for the entire
team.
Rethinking ‘Big Data’ — and the rift between business and data ops

Just as data scientists need to think more like businesspeople, so too must
businesspeople think more like data scientists. This goes to the issue of
occupational identity. Executives need to expand their professional identities
to include data. Data professionals need to recognize that DI (changes in
information) do not necessarily equate to DB (changes in behavior). Going
forward data professionals are not just in the information/insight delivery
business, they are in the “create insight that drives value creating behavior”
business. The portfolio of tools available now have democratized the practice of
data science. One no longer needs to be a math genius or coding phenom to
extract value from data — see Becoming a Data Head: How to Think, Speak, and
Understand Data Science, Statistics, and Machine Learning by Alex J. Gutman,
Jordan Goldmeier. ... Executives need ready access to data professionals to
guide their use of data power tools. Data professionals need to be embedded in
the business rather than quarantined in specialized data gulags.
The Technical Product Owner
There is a risk that the technical Product Owner or product manager might no
longer focus on the “why” but start interfering with the “how,” which is the
Developers’ domain. Otherwise, a technical Product Owner might help the
Developers understand the long-term business implications of technical
decisions made today. ... A technical Product Owner would be highly beneficial
when the product involves complex technical requirements or relies heavily on
specific technologies. For example, in projects involving intricate software
architecture or specialized domain knowledge, a technical Product Owner can
provide valuable guidance, facilitate more informed decision-making, and
effectively communicate with the Developers. This deep technical understanding
can lead to better solutions, improved product quality, and increased customer
satisfaction, especially in industries with critical technical expertise, such
as software development or engineering.
The digital transformation divide in Europe’s banking industry

Europe’s digital divide is a product of typical characteristics: internet
connectivity, digital literacy, the availability of smartphones and digital
devices. Disparities in broadband access in urban and rural communities remain
stubbornly persistent. According to Eurostat, around 21% of rural households
in the European Union do not have access to broadband internet, compared to
only 2% of urban households. In Romania, which ranked lowest on the EU’s
Digital Economy and Society Index in 2022, the market is dominated by
incumbent banks. Only 69.1% of adults hold a bank account, pointing to low
levels of financial literacy and inclusion – underpinned by a preference for a
cash economy. In contrast, the UK has a rate of over 60% fintech adoption
growth according to data from Tipalti, and Lithuania has established itself as
an impressive fintech ecosystem backed by the nation’s central bank. However,
it is too simplistic to reduce the digital divide to regional disparities, as
the starker differences lie between countries themselves.
Why AMTD Is the Key to Stopping Zero-Day Attacks
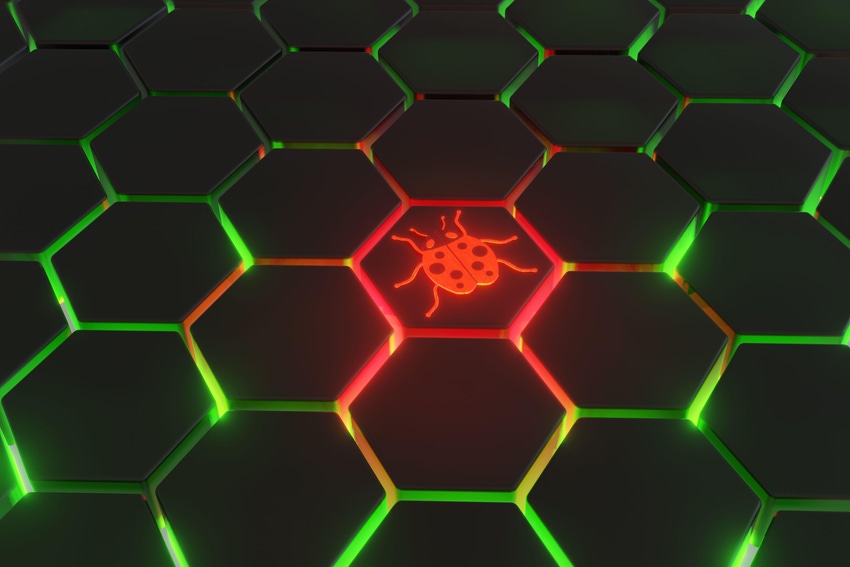
AMTD technology uses polymorphism to create a randomized, dynamic runtime
memory environment. Deployable on endpoints and servers, this polymorphism
ability creates a prevention-focused solution that constantly moves system
resources while leaving decoy traps in their place. What occurs next is that
threats see these decoy resources where real ones should be and end up
trapped. For users, it’s business as usual because as they don't notice any
difference—system performance is unaffected while security teams gain a new
layer of preventative telemetry. Today, more and more companies are turning to
AMTD technologies to defeat zero days. In fact, industry analysts like Gartner
suggest that AMTD technology is paving the way for a new era of cyber defense
possibilities. That’s because instead of trying to detect zero-day compromise,
these technologies prevent exploits from deploying in the first place. Against
zero-day attacks, this is the only defensive approach organizations can rely
on.
Quote for the day:
"Always remember, your focus
determines your reality." -- George Lucas
No comments:
Post a Comment